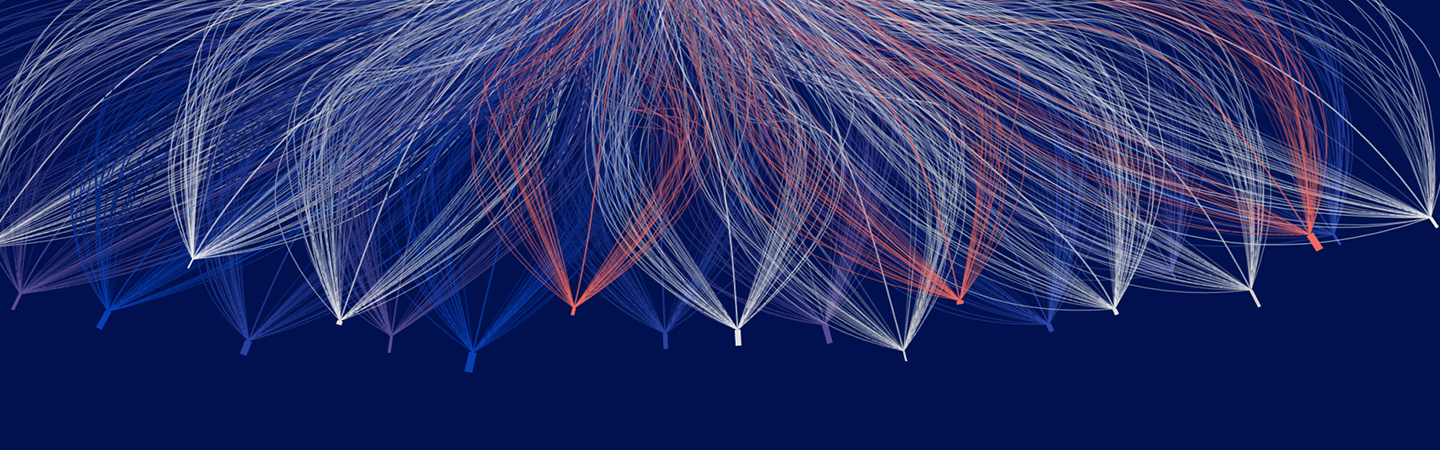
HIDA Events
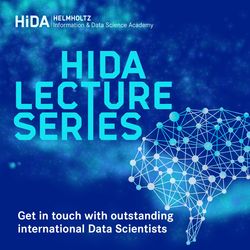
Talk to outstanding international Data Scientists about their research
The HIDA Lectures are a series of events organized by HIDA together with the six Helmholtz Information & Data Science Schools. Learn more about it here
The HIDA Lectures are a series of events organized by HIDA together with the six Helmholtz Information & Data Science Schools. Learn more about it here