At DZNE, researchers use state-of-the-art technology to decipher the mechanisms of neurodegenerative diseases.
Through HIDA’s mobility programs, data science talents can contribute to groundbreaking research projects on neurodegenerative diseases at the DZNE. The goal is to gain a deeper understanding of these conditions – and thereby significantly improve diagnostics, therapy, and prevention.
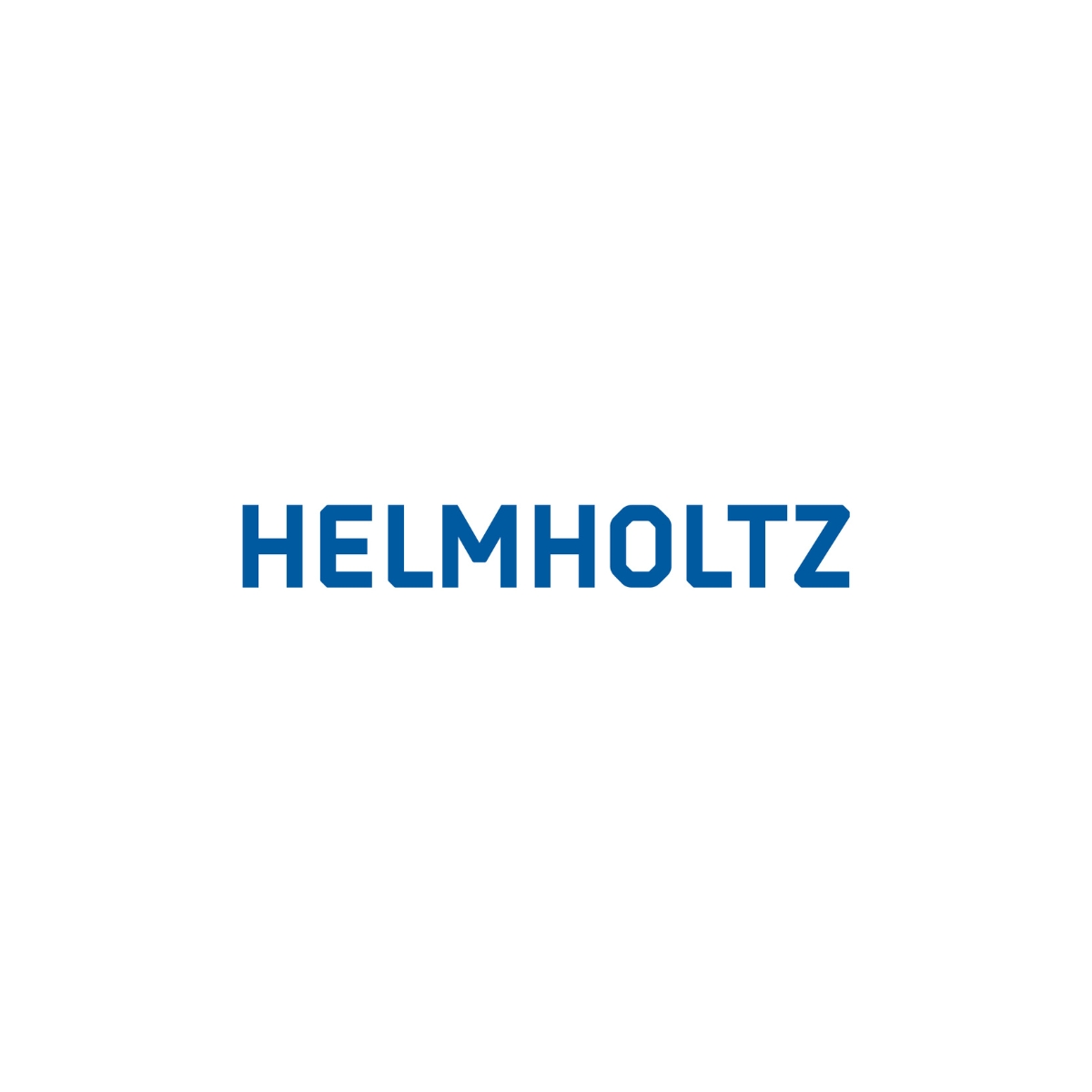
About the Helmholtz Association
About the Helmholtz Association
The Helmholtz Association is Germany’s largest scientific organization. Our cross-cutting research programs connect the 18 Helmholtz research centers.
Each center has its own scientific focus areas and infrastructures. The research is thematically structured into six fields:
- Energy
- Earth & Enviroment
- Health
- Information
- Aeronautics, Space & Transport
- Matter
The German Center for Neurodegenerative Diseases (DZNE) is one of the leading research institutions dedicated to studying disorders of the nervous system such as Alzheimer’s, Parkinson’s, and ALS. DZNE combines state-of-the-art biomedical research with innovative data analysis and AI methods to better understand the mechanisms of neurodegenerative diseases and to develop new therapeutic approaches. Its mission is to rapidly translate scientific discoveries into clinical applications in order to improve the prevention, diagnosis, and treatment of these conditions.
Research priorities:
- Cellular and molecular mechanisms of neurodegenerative diseases
- Clinical research and personalized medicine
- Epidemiology and risk factors of neurodegenerative diseases
- Translational research and the development of new therapies
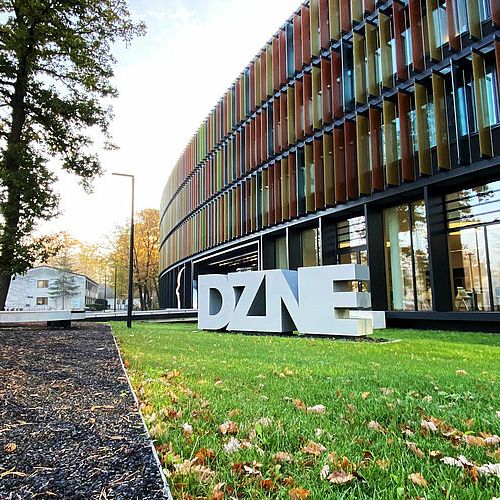
The sites
The sites
Main site: Bonn
Additional sites:
- Berlin
- Dresden
- Göttingen
- Magdeburg
- München
- Rostock/Greifswald
- Tübingen
- Ulm
- Witten
DZNE's expertise in the field of Data Science and AI
DZNE leverages outstanding AI technologies and data-driven analytics to decode complex neurological data. Interdisciplinary teams collaborate on innovative models for the prediction, diagnosis, and treatment of neurodegenerative diseases.
By applying AI and big data analytics, vast amounts of imaging, genetic, and clinical data can be efficiently analyzed to uncover new insights into disease mechanisms and advance personalized medicine.
- AI-based analysis of MRI and PET scans
- Automated pattern recognition in genetic and molecular data
- Deep learning for early diagnosis of neurodegenerative diseases
- Development of simulation models for disease progression
- Multimodal data integration to optimize personalized therapies
Across ten sites, 1,200 employees at DZNE are working to uncover the causes and progression of neurodegenerative diseases.
Application
Would you like to conduct research and work at DZNE? Then apply now for the HIDA Mobility Program!
Please contact your potential supervisor by email before applying to propose and discuss a research project. Only submit your application after this has been clarified.
You can find more information about the application requirements here.
Note for external applicants:
If you have any questions about application formalities or organizational procedures, please contact your home institution directly.
The Hosts at DZNE
Get to know some of the hosts at DZNE and learn more about their respective research based on data science.
Please note: The listed hosts represent only a selection of possible supervisors.
You are also welcome to independently contact other potential hosts at the center and coordinate your participation in the HIDA Mobility Program directly with them.
If you have any questions, please send an email to: hida@helmholtz.de
Are you interested in becoming a Helmholtz host yourself and looking for support for your research project?
Then please also contact the above-mentioned email address.
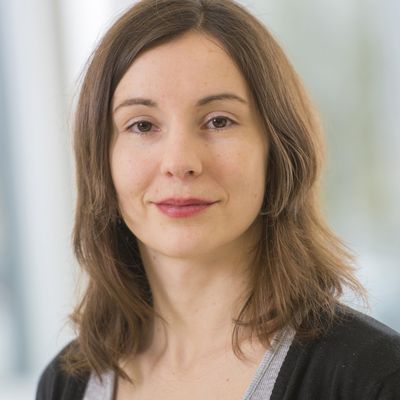
Maren Büttner
Systems Medicine
Contacts
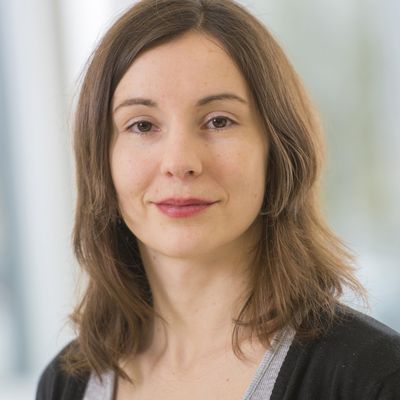
Short summary of your group's research: Systems medicine at the DZNE aims to develop holistic approaches to tackle diseases such as Alzheimer’s, Parkinson’s, or amyotrophic lateral sclerosis (ALS) and translate new insights into the clinics. We aim to change how flow cytometry data is currently analyzed. Technological advances in flow cytometry increased the possible number of features to be comparable to mass cytometry. However, the most widely used analysis methods for flow data is the targeted gating approach, which relies on manual selection of cell populations. This approach has several shortcomings as results vary across experts and for one person over time, does not scale well for hundreds of patients and samples, and tends to focus on known cell types, such that new discoveries especially in the disease context become unlikely.
What could a participant of the HIDA Trainee Network learn in your group? How could he or she support you in your group? A guest researcher can learn how to leverage deep learning models to jointly integrate flow cytometry data from different sites and enable joint integration without sharing data with our swarm learning approach.
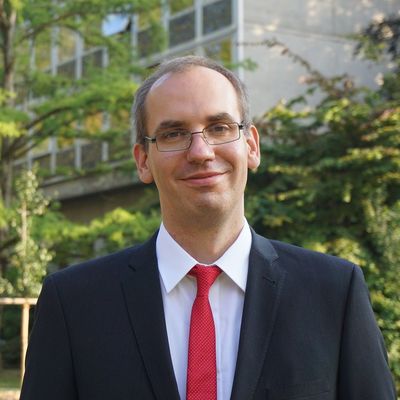
Matthias Becker
Modular High Performance Computing & AI
Contacts
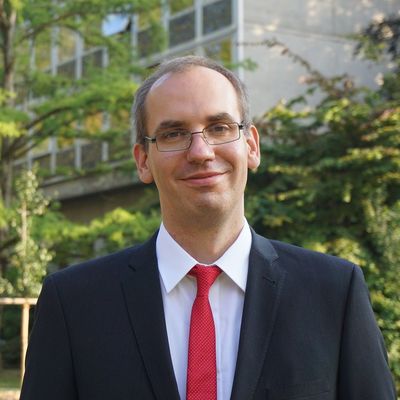
Short summary of your group's research: The Modular High Performance Computing and Artificial Intelligence group works on building and improving computational architectures for growing data sets in neurodegeneration with the focus on memory-centric systems. Furthermore, we employ swarm learning for federated machine learning in many different applications. Another application of artificial intelligence is the use of GANs and VAEs to create synthetic cohorts in a Helmholtz AI project.
What infrastructure, programs and tools are used in your group?
- We use high-memory systems (6TB+) for improving the processing of genomics data analysis applications using c/C++ and Rust.
- For ML applications, we have GPU ressoucres available at the institute and access to Jülich. Development is mainly done in Python using common libraries like Pytorch (Lightning) or Tensorflow.
What could a participant of the HIDA Trainee Network learn in your group? How could he or she support you in your group? A guest researcher will join a highly interdisciplinary group at the intersection of computer science and medicine. We are well integrated with in the systems medicine research area and collaborate with clinical partners. Projects can be either focused on the performance analysis and optimization of data processing task in genomics or on ML applications. Here the implementation of a swarm learning use case in a new domain would be option as well as work synthetic cohort generation.