Forschungszentrum Jülich is working on solutions for a sustainable and digital future—powered by interdisciplinary know-how and strong international networks.
Through HIDA's mobility programs, data science talents have the opportunity to contribute to pioneering projects at Forschungszentrum Jülich that address complex challenges of the future.
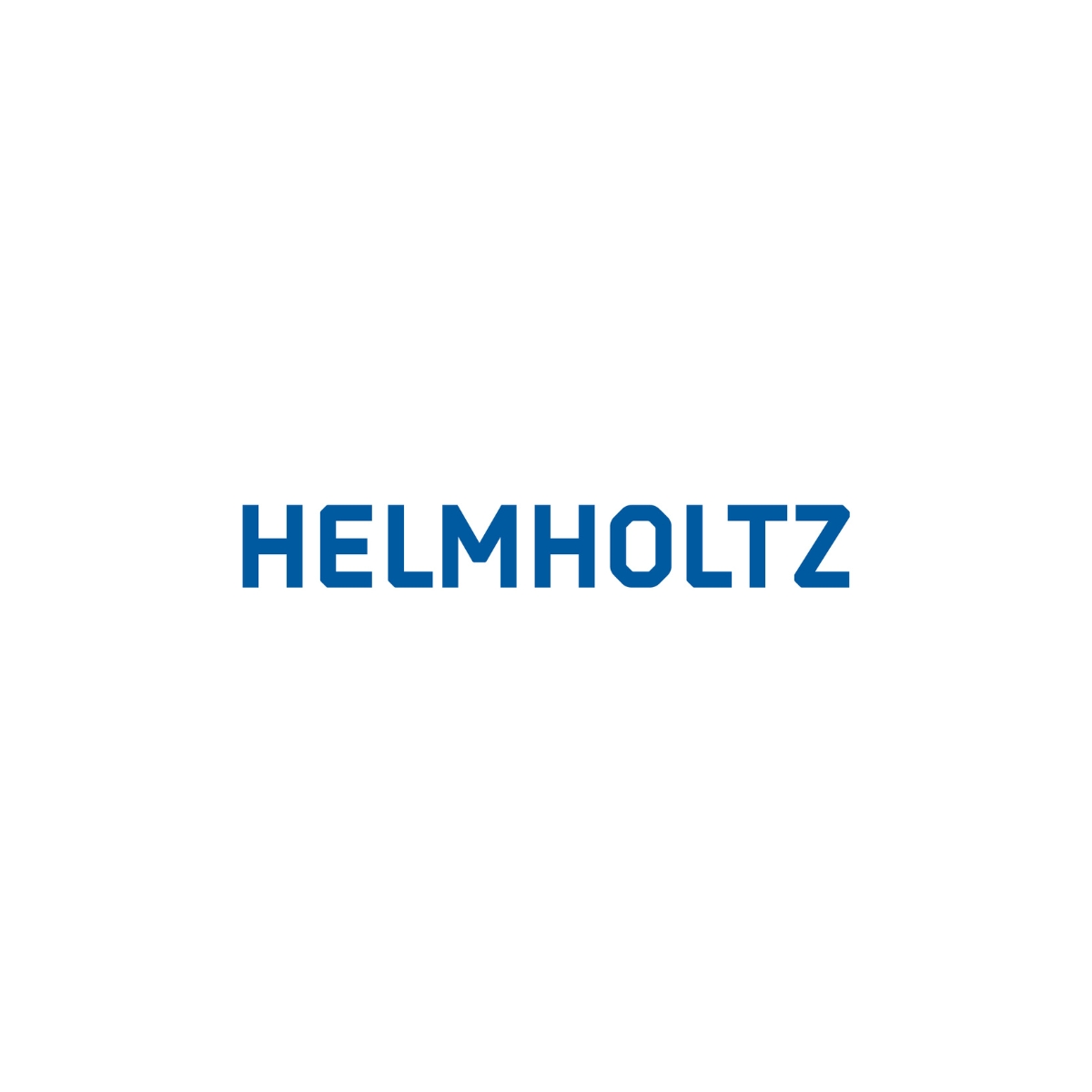
About the Helmholtz Association
The Helmholtz Association
The Helmholtz Association is Germany’s largest scientific organization. Our cross-cutting research programs connect the 18 Helmholtz research centers.
Each center has its own scientific focus areas and infrastructures. The research is thematically structured into six fields:
- Energy
- Earth & Enviroment
- Health
- Information
- Aeronautics, Space & Transport
- Matter
Forschungszentrum Jülich is one of the major interdisciplinary research institutions in Europe and, as a member of the Helmholtz Association, contributes to addressing the grand societal challenges of our time.
The goal is to conduct research that makes a real impact. For this reason, fundamental principles, technologies, and systems are explored across disciplines—for a digitalized society, a climate-friendly energy system, and sustainable economic practices. Research in the natural sciences, life sciences, and engineering focuses on the fields of information, energy, and bioeconomy, and is closely linked with expertise in high-performance computing and artificial intelligence.
In this context, Jülich operates unique scientific infrastructures that are also made available to external users.
Research priorities:
- Information
- Energy
- Bioeconomy
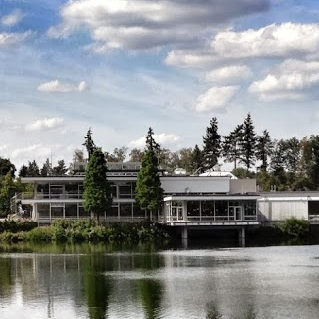
The sites
Sites & Research Collaborations of Forschungszentrum Jülich
Main Location: Jülich
Forschungszentrum Jülich operates branch offices both in Germany and abroad, featuring unique large-scale research facilities. These include joint institutes with universities and locations of project management organizations.
Research Collaborations
Forschungszentrum Jülich works closely with numerous partners at national and international levels.
In 2023, the center was involved in 638 nationally funded research projects, 132 of which had a contract volume of €1 million or more. A total of 277 projects involved multiple partners, and Jülich coordinated 66 research consortia.
Infrastructure
Researchers at Forschungszentrum Jülich have access to extensive and highly specialized research infrastructures. Facilities such as the
- Helmholtz Nano Facility (HNF)
- Ernst Ruska-Centre for Microscopy and Spectroscopy with Electrons (ER-C)
- Jülich Centre for Neutron Science (JCNS)
complement one another and are also available to external scientists as world-class infrastructures.
Further infrastructures include:
- Jülich Supercomputing Centre (JSC)
- Jülich Unified Infrastructure for Quantum Computing (JUNIQ)
- Helmholtz Nano Facility
- German Plant Phenotyping Network (DPPN)
- EBRAINS
- Ernst Ruska-Centre (ER-C)
- Jülich Centre for Neutron Science (JCNS)
FZJ expertise in the field of Data Science and AI
Forschungszentrum Jülich leverages cutting-edge AI technologies and high-performance computing capabilities to analyze complex data from various scientific domains. Interdisciplinary teams develop innovative algorithms and models to simulate and optimize scientific processes.
By applying AI and data-driven methods, new approaches are emerging in materials research, climate simulations, quantum computing, and personalized medicine.
- AI-based analysis of brain data for neuroscience
- Automated pattern recognition in high-throughput simulations
- Machine learning for quantum computing and supercomputing
- Development of simulation models for sustainable energy concepts
- Multimodal data integration to optimize scientific prediction models
At Forschungszentrum Jülich, nearly 7,450 people work hand in hand with 1,457 guest researchers from 72 countries.
Application
Would you like to conduct research and work at Forschungszentrum Jülich? Then apply now for the HIDA Mobility Program!
Please contact your potential supervisor by email before applying to propose and discuss a research project. Only submit your application after this has been clarified.
You can find more information about the application requirements here.
Note for external applicants:
If you have any questions about application formalities or organizational procedures, please contact your home institution directly.
The Hosts at FZJ
Get to know some of the hosts at FZJ and learn more about their respective research based on data science.
Please note: The listed hosts represent only a selection of possible supervisors.
You are also welcome to independently contact other potential hosts at the center and coordinate your participation in the HIDA Mobility Program directly with them.
If you have any questions, please send an email to: hida@helmholtz.de
Are you interested in becoming a Helmholtz host yourself and looking for support for your research project?
Then please also contact the above-mentioned email address.
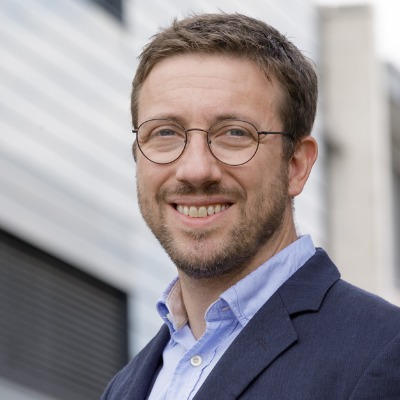
Timo Dickscheid
Structural and functional organisation of the brain
Contacts
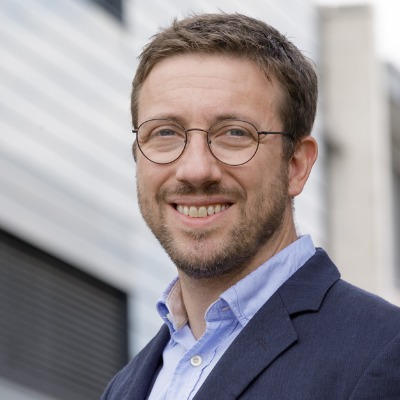
Short summary of your group's research: The Big Data Analytics group in Jülich develops software and methodology to build a microscopic resolution 3D model of the human brain, which includes detailed information about distributions of neuronal cells and nerve fibers, and microstructurally defined 3D maps of brain areas. This requires microscopic imaging and analysis of large quantities of histological brain sections at high throughput, leading to image datasets at the Petabyte scale.
At the intersection of neuroinformatics, computer vision and artificial intelligence (AI), our research addresses
- Data and workflow management for high throughput microscopic imaging,
- Machine learning and computer vision algorithms for biomedical image analysis on high performance computers,
- Software development for structured access to large image datasets and interactive 3D exploration of high-resolution brain atlases over the web.
What infrastructure, programs and tools are used in your group? The trainee will be part of the local Helmholtz AI unit in Jülich. Our data management and image analysis workflows run on a distributed environment which includes Jülich’s modular high-performance computing systems. Image acquisition of whole-brain sections at micrometer resolution is performed on a high throughput microscopy facility. Outputs of our research are typically integrated with the human brain atlas hosted in the European research infrastructure EBRAINS, to which we contribute key developments. Most of our software is implemented in Python. Image analysis and machine learning approaches use common Deep Learning frameworks, typically PyTorch or Tensorflow.
What could a participant of the HIDA Trainee Network learn in your group? How could he or she support you in your group?
A trainee in our group can learn about
- biomedical computer vision, especially processing and analysis of high resolution image data using state of the art AI methods
- distributed workflow management and parallel processing of big image data on supercomputers
- neuroinformatics.
A trainee can support our group with practical experience in machine learning approaches for image analysis from the point of view of another application domain. A trainee could also contribute with experience in inverse modelling, simulation, or computer graphics.
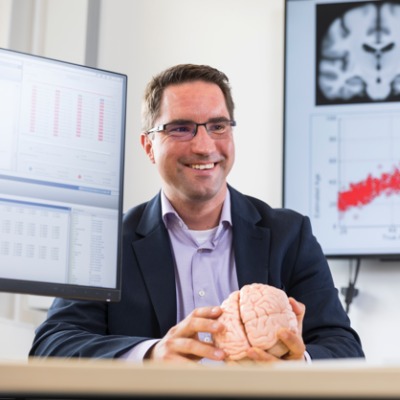
Simon Eickhoff
Brain and Behaviour
Contacts
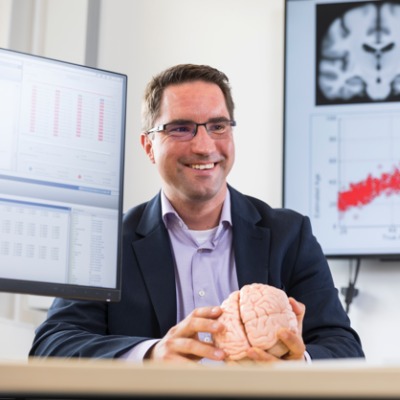
Short summary of your group's research: We develop and apply novel methods to better understand the organization of the human brain and to relate it to behavioral phenotypes in healthy and as biomarkers in clinical populations. To do so, we capitalize on machine learning approaches. In addition to these scientific objectives, we also develop open access methods and tools for data management and reproducible research.
What infrastructure, programs and tools are used in your group? Python, R, MATLAB, HPC, machine learning, neuroimaging
What could a participant of the HIDA Trainee Network learn in your group? How could he or she support you in your group? A HIDA trainee can choose from diverse topics from machine learning, data management to biomarker discovery. For technically oriented trainees we offer opportunities to contribute to our growing set of tools. For application-oriented trainees we offer participation in ongoing projects to uncover brain-behavior relationships.
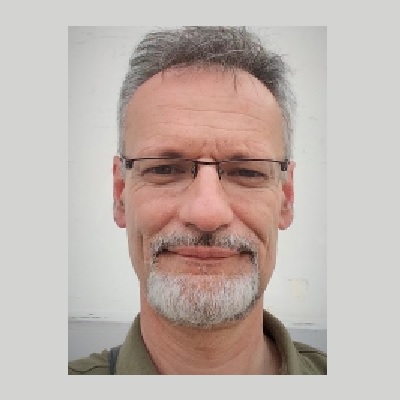
Fabio Fiorani
Plant Sciences
Contacts
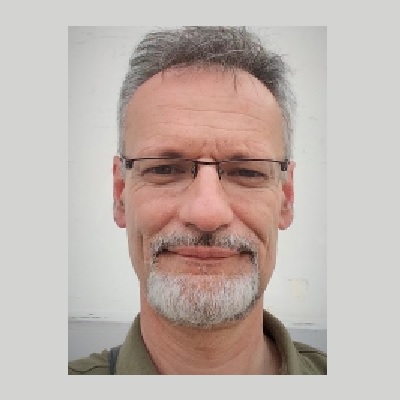
Short summary of your group's research: We focus on the application and development of high-throughput, non-invasive phenotyping methods for screening of plant shoot and root productivity traits. In our group teams of plant biologists, engineers and image processing specialists cooperate to realize screening methodologies for shoot and root growth and architecture combined with physiological measurements of plant performance and plasticity to environmental challenges. Interpretation of results requires the evaluation of multi-dimensional data structures which include dynamic changes of plant phenotypes over time, environmental and imaging sensors data.
What infrastructure, programs and tools are used in your group? We have developed and operate a unique set of automated experimental platforms to analyze plant phenotypes in a minimally-invasive fashion, please click here to learn more.
What could a participant of the HIDA Trainee Network learn in your group? How could he or she support you in your group? Participants to the HIDA Trainee Network would benefit from learning: a) methodologies for high-throughput screening in plants; b) data analyses and visualization of complex data sets; c) experimental designs for plant phenotyping. HIDA Trainee Network participants would support our continued efforts in improving and automating data analyses using existing data stored in several institutional databases.
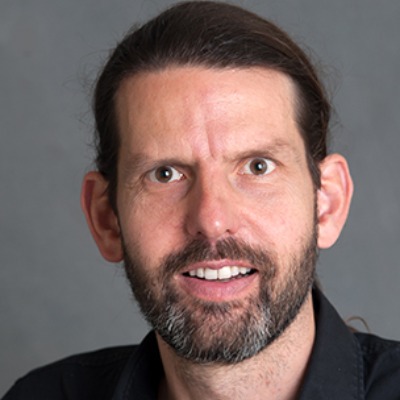
Eric von Lieres
Modeling and Simulation
Contacts
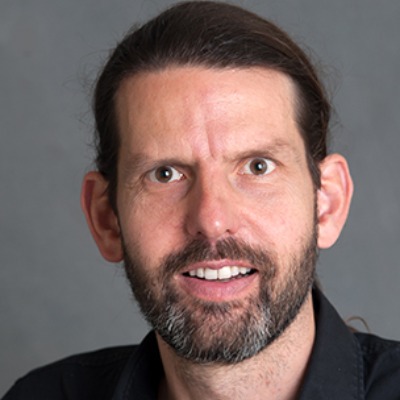
Short summary of your group's research: We provide our local colleagues and the scientific community with state-of-the-art models, algorithms and software. Current challenges in strain selection and optimization as well as process analysis and development are addressed in a strongly data-driven fashion in close collaboration between theory, simulation and experiment. Typical projects involve data analysis, model calibration or training, uncertainty analysis, experimental design. Model predictions are applied for testing hypotheses and focusing experimental work.
What could a participant of the HIDA Trainee Network learn in your group? How could he or she support you in your group? Many projects require advanced numerical methods and high-performance computing, in particular when machine learning/ artificial intelligence, Monte Carlo simulations, global optimization or CFD simulations are applied. We develop and maintain dedicated software packages, most of which are published as open source code https://github.com/modsim.
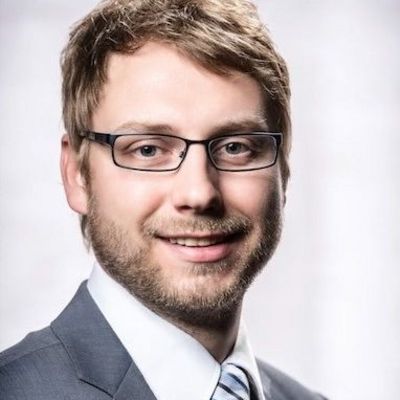
Andreas Lintermann
Fluids & Solids Engineering
Contacts
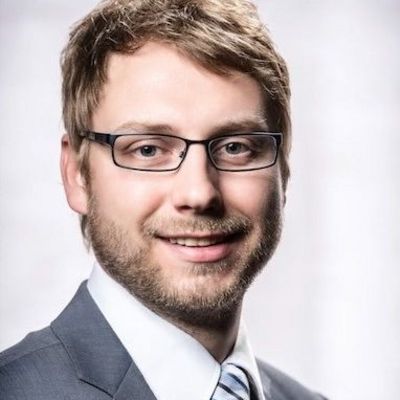
Short summary of your group's research: The SDL FSE's research focuses, amongst others, on lattice-Boltzmann methods, artificial intelligence, high-performance computing, heterogeneous computing on modular supercomputing architectures, high-scaling meshing methods, efficient multi-physics coupling strategies, and bio-fluidmechanical analyses of respiratory diseases. Furthermore, the SDL FSE aims at supporting users from engineering sciences who have already developed parallel codes but need support for the use of massively parallel systems regarding high scalability, memory optimization, programming of hierarchic computer architectures, and performance optimization on compute nodes.
What infrastructure, programs and tools are used in your group? The group mainly uses the high-performance computing (HPC) systems available at JSC for its various simulation applications. As a simulation code, a massively parallel multi-physics framework, jointly developed with the Institute of Aerodynamics and Chair of Fluid Mechanics, RWTH Aachen University, is employed. The big data outputs generated by the simulation tools are post-processed, e.g., by in-house developed tools, ParaView, machine-learning algorithms, or by Jupyter-based scripts.
What could a participant of the HIDA Trainee Network learn in your group? How could he or she support you in your group? The doctoral researcher will have access to the latest supercomputing hardware installed at JSC and will learn from the experts in the group to develop, adapt, and optimize simulation codes. He or she will support the member of the SDL FSE in running large-scale simulations on the production HPC machines at JSC and post-process the data to gain new insights to physical phenomena in the realm of fluid mechanics. Furthermore, the doctoral researcher will be able to learn how research in a European Center of Excellence in Exascale Computing is performed and has the opportunity to contribute to the corresponding cutting-edge research in bringing AI technologies along various use-cases to exascale.
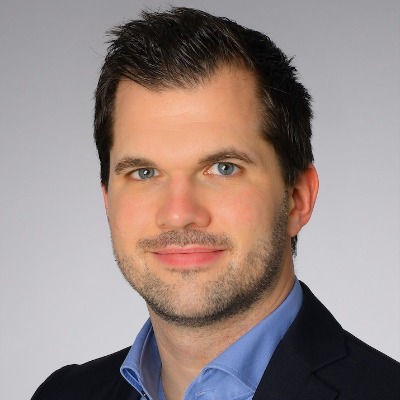
Philipp Lohmann
Physics of Medical Imaging
Contacts
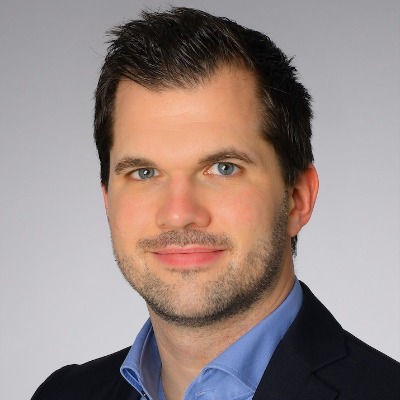
Short summary of your group's research: The research of the Brain Tumor Group within the Institute of Neuroscience and Medicine focuses on multimodal imaging in patients with brain tumors, with particular emphasis on amino acid PET in combination with advanced high-field and ultra-high-field MRI. In addition to the development and evaluation of novel PET tracers and PET/MRI methods, the potential of advanced image analysis such as radiomics and deep learning for patients with brain tumors is explored. A major goal of our research is the correlation of imaging findings and radiomics with local neuropathology and histomolecular markers, for which the close collaboration with the surrounding university hospitals in Aachen, Bonn, Cologne, Düsseldorf, and others, is extremely valuable and furthermore offers a high potential for verification and translation of research results into the clinic.
What infrastructure, programs and tools are used in your group? Amino acid PET, advanced MRI, ultra-high-field MRI, hybrid MR/PET, Python, Pyradiomics, PyTorch, LifeX, PMOD, FSL, HDBET, HDGLIO, ITK-SNAP, OsiriX, Matlab, High performance computing, etc.
What could a participant of the HIDA Trainee Network learn in your group? How could he or she support you in your group?
- Amino acid PET in combination with advanced, ultra-high-field MRI in patients with brain tumors
- Advanced image analysis including static and dynamic amino acid PET and MRI
- Feature-based as well as deep learning-based radiomics based on PET and MRI in patients with brain tumors
- Correlation of PET/MRI imaging findings and radiomics with local neuropathology and histomolecular markers
- Establishment of an imaging database of amino acid PET imaging in patients with brain tumors for advanced image analysis
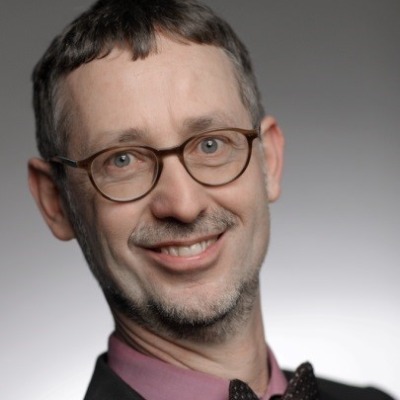
Wolfgang Wiechert
Biotechnology
Contacts
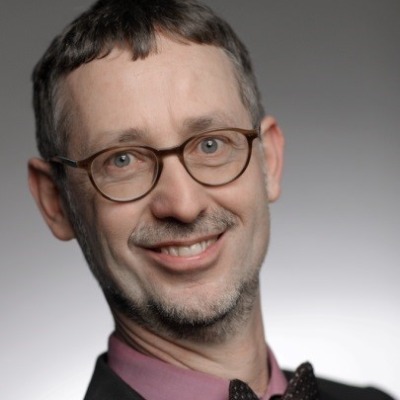
Short summary of your group's research: Modeling, Simulation and Data Analytics in the fields of systems metabolic engineering and bioprocess development: Cell and process modeling, Omics and bioprocess data processing, process parameter estimation, microbial image analysis, digitalization in lab automation
What infrastructure, programs and tools are used in your group? Hosting CADET and 13CFLUX2 software systems. Using and developing C++, python, Matlab toolboxes. High performance parameter estimation in complex systems, MCMC for parameter estimation and multi model analysis. Deep learning for image analysis. Supercomputing applications in process simulation.
What could a participant of the HIDA Trainee Network learn in your group? How could he or she support you in your group?
Wanted: Expertise in cutting edge Bayesian methods, image analysis and high performance computing
Offering: Challenging realistic problems from the above mentioned fields
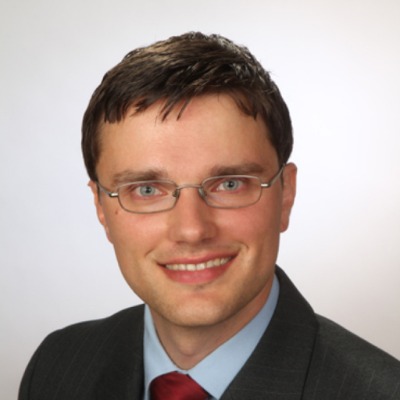
Dieter Weber
Microscopy and Spectroscopy with Electrons
Contacts
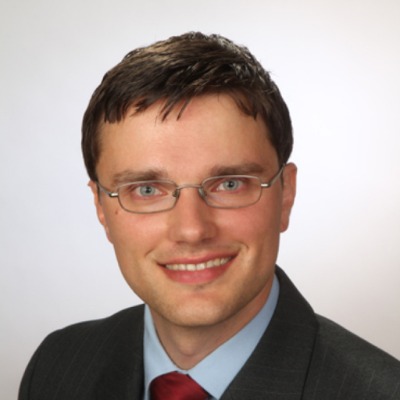
Short summary of your group's research: We are developing solutions for high-performance data processing, data management and automation in electron microscopy. This includes software like here as well as IT systems and infrastructure. Since modern detectors for electron microscopy can reach a data rate of 50 GB/s, datasets can contain terabytes of data, and electron microscopy is a visual and interactive method, these solutions can be characterized as interactive high performance computing.
What infrastructure, programs and tools are used in your group?
- MapReduce-like processing, "divide and conquer", linear algebra, inverse problem solving
- Python, NumPy, SciPy, Dask, Numba, CuPy, Torch, Jupyter, JupyterHub;
- Linux, Windows;
- Nextcloud, NAS, NFS, CIFS, SSH;
- GitHub, GitLab, Azure Pipelines, PyPI, Zenodo;
- Tox, Pytest, Sphinx;
- product management, project management, software development, test-driven development, Agile;
- PCIe 4.0, SSD RAIDs, 10 GBit Ethernet, AMD EPYC, GPGPU
What could a participant of the HIDA Trainee Network learn in your group? How could he or she support you in your group? You can learn high performance data processing with Python, in particular throughput-oriented processing of large-scale data. That can also involve finding suitable mathematical approaches and implementation strategies to transform an existing "proof of principle" reference implementation into a production-ready high-performance tool. Typically, we achieve speed-ups of 100x to 1000x from a "naive" NumPy or Matlab-based implementation.
Furthermore, you can learn software development methodologies, in particular the "GitHub flow", in combination with quality assurance through automated tests and continuous integration.
Contributions to scientific Open Source software are highly appreciated as a form of support. That can involve software which we actively maintain and develop, work on upstream dependencies, or all other forms of contributions to the Open Source software ecosystem. Such contributions can be in-kind by simply improving existing software and/or releasing new software under an Open Source license, or by joint applications for funding where parts of the resources are dedicated to software development and maintenance.
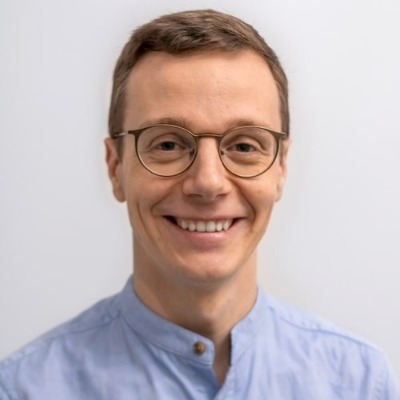
Gabriele Cavallaro
Simulation and Data Lab
Contacts
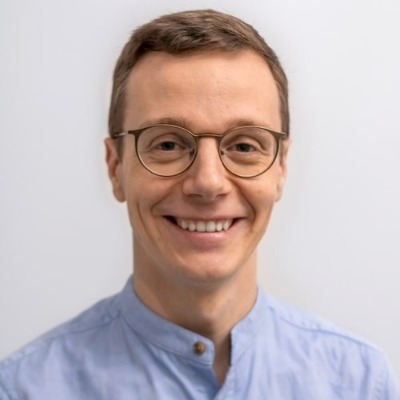
Short summary of your group's research: The joint research group ''High Productivity Data Processing (HPDP)'' at JSC and University of Iceland is highly active in developing parallel and scalable machine (deep) learning algorithms for remote sensing data processing and many other types of applications (i.e., medical research and retail sectors). The main backbone of the research group is the large number of PhD students that are jointly supervised with the University of Iceland. Furthermore, the HPDP works actively with the Cross-Sectional Team Deep Learning (CST DL) and the Helmholtz AI consultant team at JSC.
What infrastructure, programs and tools are used in your group? By being located at JSC, HPDP can rely on HPC technologies with MPI, OpenMP and CUDA (with TensorFlow, Keras, pyTorch, Chainer, Horovod) but also on innovative quantum computing systems.
What could a participant of the HIDA Trainee Network learn in your group? How could he or she support you in your group? Learn how data intensive computing approaches have become indispensable tools to deal with the challenges posed by applications from a diverse range of applications.
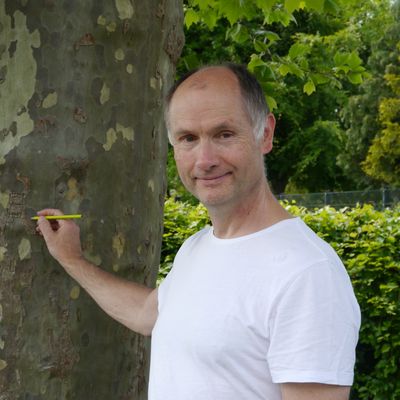
Martin Schultz
Earth System Data Exploration
Contacts
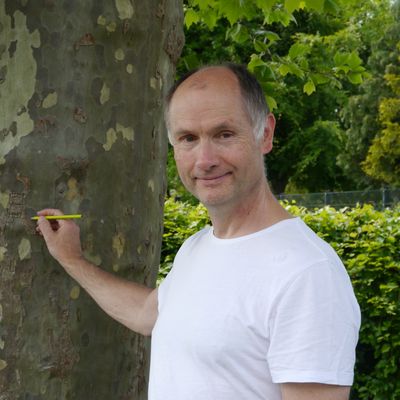
Short summary of your group's research: ESDE explores a wide range of machine learning methods for the analysis and forecasting of weather, climate and air quality data. We focus primarily on large, computationally intensive problems and adopt high-end deep learning approaches. Next to machine learning research we are also strongly interested in developing FAIR big data workflows. The 20+ group members have diverse educational backgrounds and build a lively international team of highly motivated researchers and software developers.
What infrastructure, programs and tools are used in your group? We make good use of JSC's high-end computing systems (https://go.fzj.de/juwels) and also operate on smaller GPU clusters. Tensorflow and Pytorch are our main (Python) software libraries. We also use containers and tools like Horovod to port ML codes to the supercomputer.
What could a participant of the HIDA Trainee Network learn in your group? How could he or she support you in your group? If you already have a good background in advanced machine learning techniques (i.e. beyond MLP) you may take it to the next level with a visit to our group and we will be happy to teach you the necessary basics of weather and air pollution science so that you can apply your skills to the problems we work on in our group. If you have a strong background in environmental science but only limited experience with machine learning (but you know how to program in Python) you may learn a lot about more sophisticated deep learning methods and we might be able to integrate your specific experience to better address our environmental research questions.
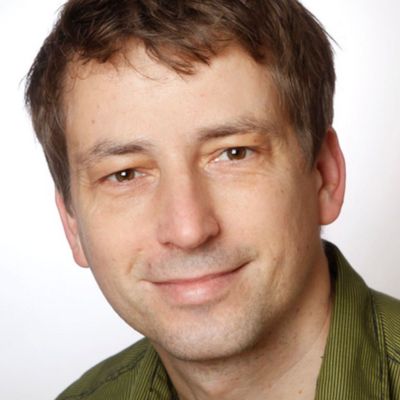
Christoph Lerche
Physics Group, Medical Imaging Physics
Contacts
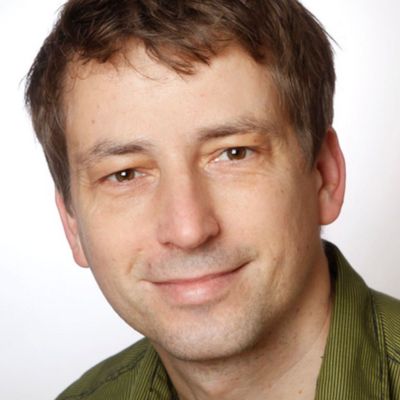
Short summary of your group's research: The PET physics group focusses on
- PET methodology development (image reconstruction and data corrections as scatter correction and attenuation correction)
- Multimodal Imaging (Applications and Methodology for combined PET/MR and PET/MR/EEG acquisitions) System development of an UHF MR compatible BrainPET insert
- Quantitative Methods for PET and PET/MR imaging
What infrastructure, programs and tools are used in your group?
- 3T MR-PET insert, consisting of a high resolution BrainPET, (a prototype by Siemens), and a commercial 3T MAGNETOM Trio MRI scanner with several phantoms
- Access to the Radiochemistry department for generation of Radio-tracer and Radionuclides
- Access to High Performance Computing Installations
- Access to PET and MR patient and volunteer data from other studies (neuroreceptor/neurotransmitter studies, neuroonkology)
- Monte Carlo Simulation tools Geant4/Gate, analysis tools: PMOD, SPM
What could a participant of the HIDA Trainee Network learn in your group? How could he or she support you in your group? Our group offers the following expertises
- Thorough understanding of PET systems and Image-formation from gamma photon detection to image reconstruction including all relevant corrections (scatter, attenuation, randoms, dead time)
- Unique access to a dedicated 3T MR BrainPET insert and a 7T human MRT in a research setting with all technical an league requirements to run studies involving human volunteers
- Starting in 2023: access to a in-house developed 7T MR BrainPET insert for humans
- We would highly benefit from guest researcher with expertises in Image post processing (PET and MR), Data science for information extraction from multi parametric and multimodal images, Monte Carlo Simulations
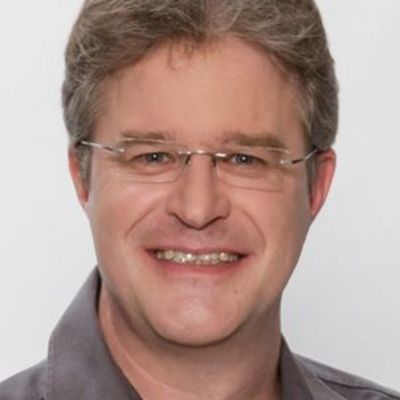
Maik Boltes
Civil Safety Research
Contacts
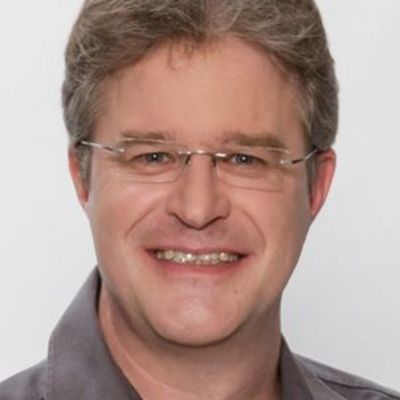
Short summary of your group's research: Our institute studies the dynamics of pedestrians to understand their behavior and thereupon develop reliable models for enhancing the safety of people inside crowds. Controlled experiments allow the quantitative description of the dynamics and enable the analysis of selected parameters under well-defined constant conditions. Data of these experiments is collected and analyzed by appropriately selected sensors and self-developed software.
What infrastructure, programs and tools are used in your group? During our experiments we are collecting a huge amount of data from (depth) cameras, motion capturing systems, IMU sensors, pressure sensors, heart rate sensors and electrodermal activity sensors sometimes combined with questionnaire data.
Our software is written in C++ or Python like our open-source tool PeTrack, which automatically extracts accurate pedestrian trajectories from videos.
Gathered data of all our experiments can be found in our open data archive.
What could a participant of the HIDA Trainee Network learn in your group? How could he or she support you in your group? We are a multidisciplinary young group of physicists, mathematicians, engineers, sociologists, psychologists, and computer scientists.
Our institute is one of the leading institutions in the field of experimental pedestrian research.
The dynamics and behavior of people in a crowd have many influences. The diversity of performed experiments and the amount of experimental data in our group is large. We learned in the past that a new view, method or discipline can give us another or extended insight to our data so that we would be happy to welcome you to our group.
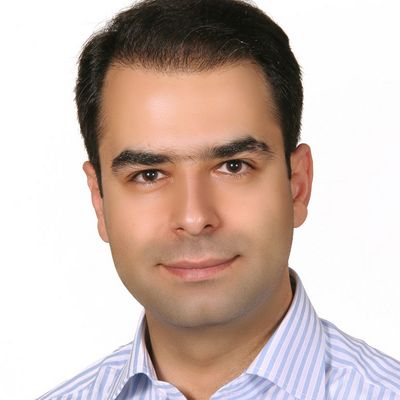
Masoud Tahmasian
Brain and Behaviour
Contacts
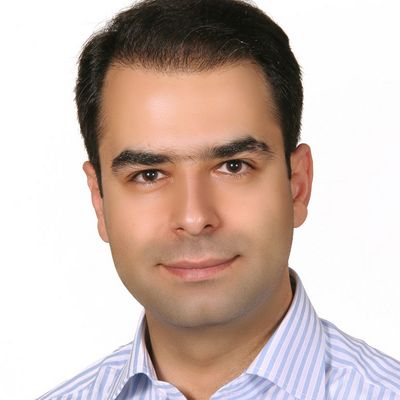
Short summary of your group's research: We use a multi-level approach utilizing computational tools and big data to gain an overall insight into neurological and psychiatric disorders to pave the way for personalized medicine. In particular, we focus on the interplay between sleep/sleep disorders and medical and mental conditions using phenotypic and multimodal neuroimaging data (e.g., sMRI, fMRI, PET).
Masoud Tahmasian is part of Prof. Dr. Simon Eickhoff's group on System Medicine.
What infrastructure, programs and tools are used in your group? We use state-of-art methodologies such as machine-learning to big clinical and population-based databases using high-performance computing tools.
What could a participant of the HIDA Trainee Network learn in your group? How could he or she support you in your group? The guest researcher will apply several analyses including machine-learning to identify the role of sleep disturbances and brain structures on predicting neuropsychiatric conditions such as depression using a large-scale general-population sample (e.g., UKBB).
He/She should perform the analyses independently and help us to build a nice analytic framework to find the neurobiological mechanisms behind the link between sleep and neuropsychiatric disorders.
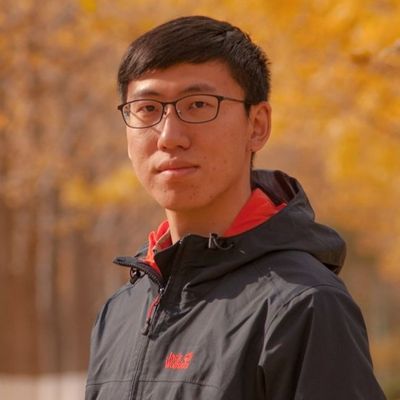
Yuankai Yang
Nuclear Waste Management
Contacts
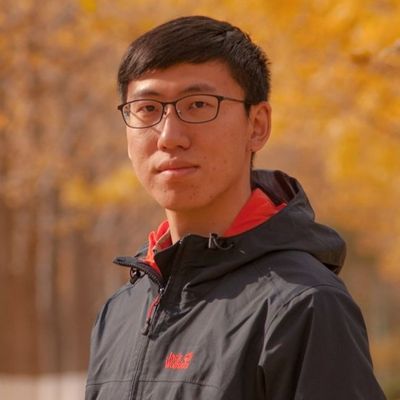
Short summary of your group's research: Analysing complex coupled thermo-hydraulic-mechanical-chemical-transport processes relevant to environmental systems on different scales.
My research at IEK-6 focuses on the development of complementary computational approaches to analyse and interpret the complex coupled THMC-processes in the near- and far-field of geological repositories for radioactive wastes on different scales, employing high-performance computing (HPC) resources provided by the Jülich Supercomputing Centre. This work aims at an enhanced process and system understanding across scales as well as at the reduction of uncertainties and conservatisms in performance assessments, contributing thus to the scientific basis for an in-depth comparison of different repository concepts and sites as required for the German site selection procedure.
What infrastructure, programs and tools are used in your group? We use the Lattice Boltzmann Method (LBM) for simulations. The High-Performance Computing (HPC) Cluster JURECA-DC is our main computational resource. Currently, we are using CUDA, Matlab, Python, Comsol, and Fortran for developing our numerical framework and data analysis.
What could a participant of the HIDA Trainee Network learn in your group? How could he or she support you in your group? The guest researcher could learn the Lattice Boltzmann method algorithm, multiscale modeling processes, and an understanding of coupled THMC-processes in geological porous media. Use of our techniques and software.
We will support the GPU High-Performance Computing Cluster in JURECA-DC. We will cooperate with the guest to develop their LBM codes. We also welcome guests with a basic background in machine learning, who want to perform joint research with us relevant to environmental systems.
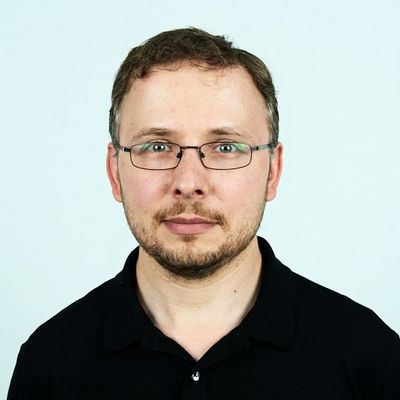
Johannes Laube
Stratosphere (ICE-4)
Contacts
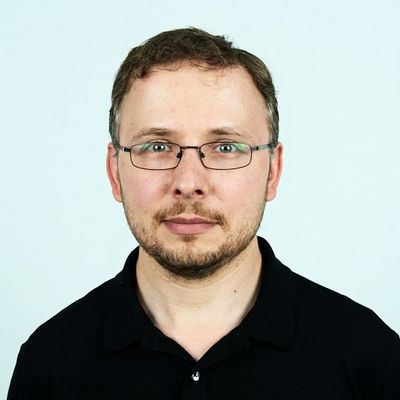
Short summary of your group's research: Our main focus is on flying sensors on balloons to stratospheric altitudes up to 35 km. For instance, we collect air samples and then subsequently analyse these for their content of trace gases important for stratospheric ozone (such as CFCs or halons) or climate (e.g., CO2 or HFCs). We can use the data to better understand the state of the ozone layer or circulation changes at altitudes well above the reach of aircraft.
What infrastructure, programs and tools are used in your group? Observation platforms are aircraft and balloons, measurements are mostly based on cavity-ringdown spectroscopy and mass spectrometry. Current project examples are the ERC Starting Grant EXC3ITE and we are also involved in Modular Observation Solutions for Earth Systems MOSES. We closely collaborate with the atmospheric modelling community, e.g. the Chemical Lagrangian Model of the Stratosphere CLaMS.
What could a participant of the HIDA Trainee Network learn in your group? How could he or she support you in your group? The learning experience would include the challenges of observations in as hostile an environment as the stratosphere, measurement and data retrieval techniques, and inferring key parameters such as average stratospheric transit times from the various trace gas data. Especially the latter part is where a guest researcher might be able to help us to improve our algorithms and procedures.
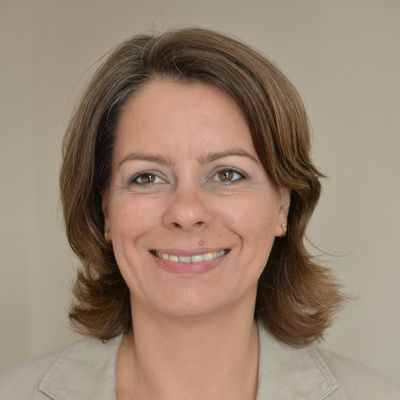
Simone Beer
Molecular Organization of the Brain
Contacts
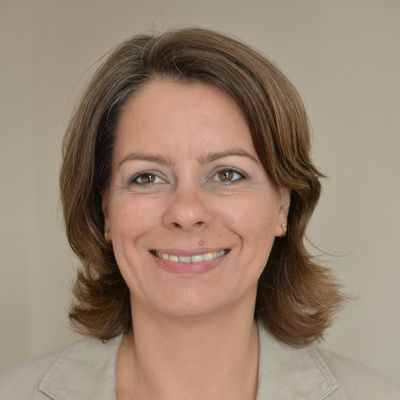
Short summary of your group's research: We use explainable AI on population-based studies to target neurodegenerative diseases, e.g. Alzheimer's disease. Machine learning algorithms like decision trees or tree ensembles can learn patterns in data, and the explanations are therefore promising tools to address interactions of genes, environment and lifestyle with respect to a certain trait or disease. We seek to evaluate and use different methods of explainable AI with respect to scientific discovery tasks.
What infrastructure, programs and tools are used in your group? Python, GitHub, access to various clinical and population-based databases like ADNI (the Alzheimer's Disease Neuroimaging Initiative), PET, hybrid MRI/PET and various laboratory techniques for preclinical and clinical research as well as research in basic neuroscience in a highly interdisciplinary environment.
What could a participant of the HIDA Trainee Network learn in your group? How could he or she support you in your group? A guest researcher will support in evaluating explainable AI for scientific discovery, especially with respect to the interaction of genetics, environment and lifestyle in neurodegenerative diseases.
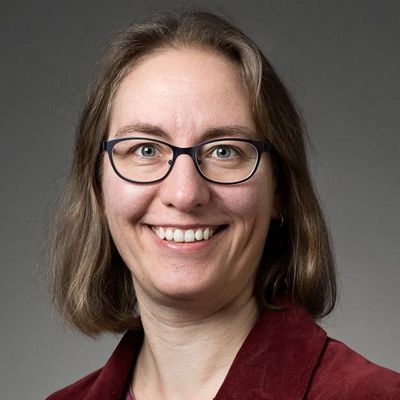
Ira Assent
Data Analytics and Machine Learning
Contacts
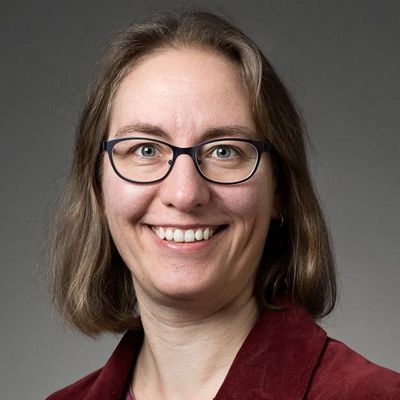
Short summary of your group's research: We aim at advancing theory and application of data sciences with a focus on image processing and computer vision for imaging as a scientific measurement tool. Our interests range from use-inspired, method-driven theory to application-driven research.
What infrastructure, programs and tools are used in your group? We work with state-of-the-art methods and algorithms in machine learning and data mining; most of our implementations are in python, and we make use of gpu-parallelization for efficiency and scalability; we devise methods that support scientists in other fields in analyzing and handling their data
What could a participant of the HIDA Trainee Network learn in your group? How could he or she support you in your group? You can learn more about how to train machine learning models, develop (variants of) such models, devise efficient algorithms, or use them to work with your data or with the data provided by our collaborators.
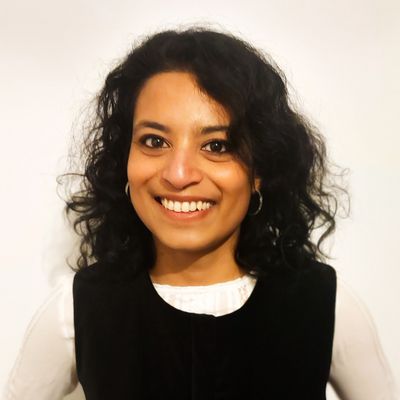
Jenna Poonoosamy
Reactive Transport
Contacts
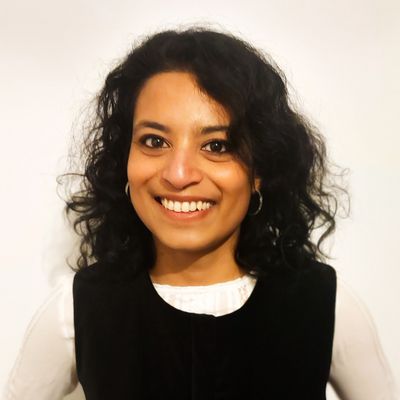
The institute performs cutting-edge research in the fields of nuclear waste management and safety, considering the process of “Energiewende”, the transition of the German energy system. This comprises fundamental as well as applied research and development for the safe management of nuclear wastes, covering issues from the atomic scale to the macroscopic scale of actual waste forms and waste packages or the engineered barrier system of deep geological repositories for nuclear wastes.
Short summary of your group's research: We apply innovative experimental and computational approaches across the scale to analyze and interpret complex coupled thermal-hydraulical-mechanical-chemical (THMC) processes in porous media related to energy applications. Recently, I have acquired an ERC project to investigate the gas water mineral interactions in confinement https://cordis.europa.eu/project/id/101040341. In this project, AI and Neural network tools will be used to (i) automate the analysis of microfluidics experiments and process experimental results (ii) derivation of constitutive equations to rationalize complex processes and (iii) accelerate geochemical processes.
What infrastructure, programs and tools are used in your group? Reactive transport tool software (Pflotran, Openegeosys-Gems, comsol multiphysics). Geochemical simulation. Image processing based on machine learning. In addition, we also have a microfluidic laboratory equipped with confocal Raman microscopy, IR and fluorescence microscopy.
What could a participant of the HIDA Trainee Network learn in your group? How could he or she support you in your group? Multiphase flow using Comsol multiphysics and geochemical modelling using phreeqc or GEMS. We have conducted novel experiments in the field of geochemistry; a lab on a chip approach to investigate radionuclide incorporation in mineral phases in a confined nano volume of (1-10nL) solution. The guest researcher would work on simulating droplet generation using comsol multiphysics and conduct geochemical modelling.
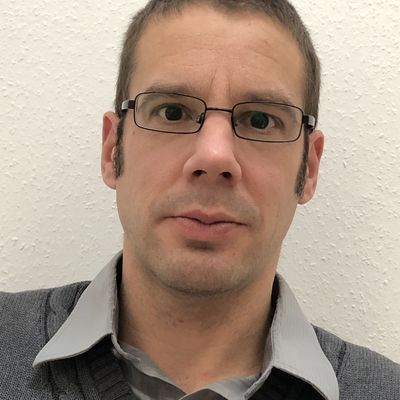
Andreas Kleefeld
Numerical and Statistical Methods
Contacts
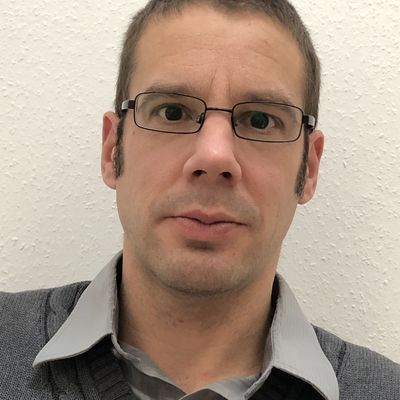
Three-sentence summary of your group's research: The ATML "Numerical and Statistical Methods" develops, implements, and tests new procedures/methods in the area of stochastic ordinary and partial differential equations that arise, for example, in image processing, acoustic, electromagnetic, and elastic scattering problems, as well as in reaction-diffusion-advection equations. In this context, the group also deals with algorithms for the solution of ill-posed inverse problems and the computation of eigenvalue problems taking real-world data into account. Furthermore, the group offers software support for supercomputers.
What infrastructure, programs and tools are used in your group? We develop software and tools in Fortran, Matlab, and Python depending on the requirements of the project.
For instance, solvers for acoustic, electromagnetic, and elastic scattering for time-harmonic waves in unbounded domains have been implemented and further used for related inverse problems. The high performance infrastructure of the Jülich Supercomputing Centre is at our disposal.
What could a guest researcher learn in your group? How could he or she support you in your group? A researcher in our group will learn how to efficiently solve partial differential equations in unbounded domains with boundary integral equations numerically. A recent development is mainly focusing on the efficient numerical calculation of interior transmission eigenvalues which can be used to visualize the interior of a given three dimensional object to uncover location, size, and shape of an inclusion for either given real-world or synthetic data which is the aim in non-destructive testing. A researcher can support our group with the development of this algorithm for HPC. Moreover, the mathematical theory still needs to be established. Finally note that a detailed description of our projects can be found at our homepage.
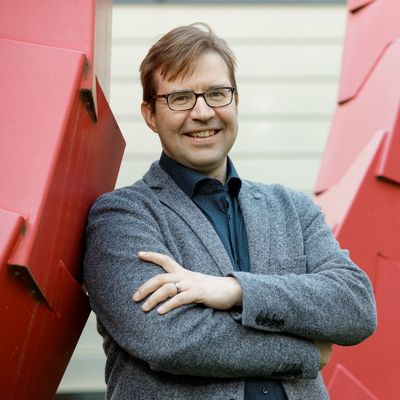
Stefan Sandfeld
Materials Data Science and Informatics
Contacts
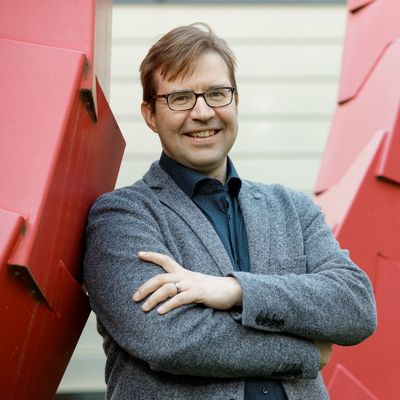
Three-sentence summary of your group's research: Research work at the IAS-9 is located at the intersection of data science and machine learning, research data management and semantic technology, and simulation in the field of computational materials science. In the data science department we investigate how predictive and generative machine learning models are able to replace or accelerate computationally expensive aspects of simulations; and as a second main topic we perform data mining of and method development for microscopy images and data. In the field of materials simulations we are particularly interested in predicting the structure-property relation in the context of solid-mechanical systems. In the field of RDM we are involved in software development and design ontologies and metadata schemas together with domain scientists.
What infrastructure, programs and tools are used in your group? Probably the main "tool" used in the IAS-9 is programming. 90% of the time, we use Python for writing code, but once in a while there is also some C/C++ or Java. Half of our activities are taking place on HPC hardware.
What could a guest researcher learn in your group? How could he or she support you in your group? A guest researcher can learn how (data) science is done in our team and how we sometimes also collaboratively work together on small projects -- sometimes with people from the field of simulation, research data management, and data science.
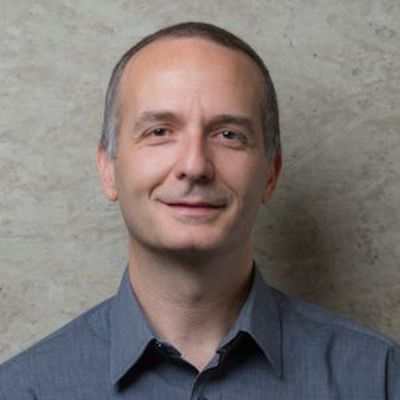
Eduardo di Napoli
Simulation and Data Laboratory Quantum Materials
Contacts
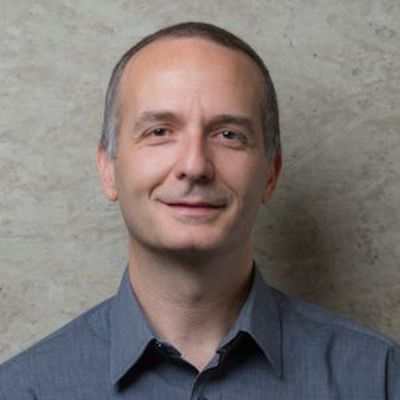
Short summary of your group's research
The Simulation and Data Laboratory Quantum Materials (SDLQM) serves as a dynamic hub for pioneering research at the intersection of simulation science and quantum materials. With a unique emphasis on leveraging High-Performance Computing and cutting-edge scientific Machine Learning, SDLQM offers a rich repository of expertise in quantum-based simulations within the realm of Materials Science. Our lab operates as a catalyst for innovation, actively engaging in dedicated projects and hosting research initiatives that delve into the essential domains of method refinement, algorithmic enhancement, and performance optimization. At the forefront of simulation science, SDLQM thrives on fostering cross-disciplinary collaborations spanning the global landscape. We take pride in our robust engagement within both the European and Japanese research arenas. Within the EU, our lab spearheads pioneering research and development initiatives, propelling the domain of Materials for Energy to new horizons. We are resolutely focused on facilitating exascale simulations of quantum transport, unraveling complexities that hold the key to transformative advancements. Our endeavors transcend borders, as we cultivate robust ties with esteemed partners in Japan, notably nurturing a dynamic synergy with the illustrious RIKEN supercomputing center in Kobe.
What infrastructure, programs and tools are used in your group?
Guided by an unwavering commitment to excellence, SDLQM not only facilitates extensive-scale simulations on state-of-the-art HPC platforms but also pioneers the training of intricate learning models driven by insights garnered from in-silico simulations. SDLQM operates at the heart of the Juelich supercomputing center, a pioneering institute that presently houses one of the largest supercomputers across the European Union. This convergence of minds and machines is set to elevate to unprecedented heights, with Europe's inaugural exascale supercomputer slated for 2024. Immerse yourself in a realm of unbounded possibilities, as the lab cultivates expertise encompassing high-performance computing, parallel programming, numerical linear algebra, quantum transport simulation, functional Renormalization Group methods and Tensor Networks. As a nexus at the frontier of knowledge, we seamlessly unite the realm of materials science simulation with the realm of massively parallel machines, thereby nurturing a community driven by cutting-edge innovation and collaborative excellence.
What could a guest researcher learn in your group? How could he or she support you in your group?
The doctoral researcher will be granted privileged access to JSC's cutting-edge supercomputing hardware, ushering them into the realm of computational supremacy. Working in close tandem with the group's accomplished experts, they will embark on a transformative journey of code development, adaptation, and optimization. Moreover, the doctoral researcher will gain firsthand exposure to the dynamic intersection of numerical linear algebra and materials science simulation. This immersive journey will encompass code integration, delving into the intricacies of parallel programming best practices, and honing indispensable software management skills. Join us in shaping the future of quantum-centric materials research, where innovative simulations and data-driven discoveries converge.