Helmholtz Munich is dedicated to researching widespread diseases such as diabetes, allergies, and similar conditions, investigating their development in the interplay of environmental factors, lifestyle, and genetic predisposition.
With the HIDA mobility program, data science talents can contribute to groundbreaking research projects on common diseases at Helmholtz Munich. The goal: to gain a deeper understanding of environmentally-related diseases in order to significantly advance diagnostics, therapy, and prevention. Apply now!
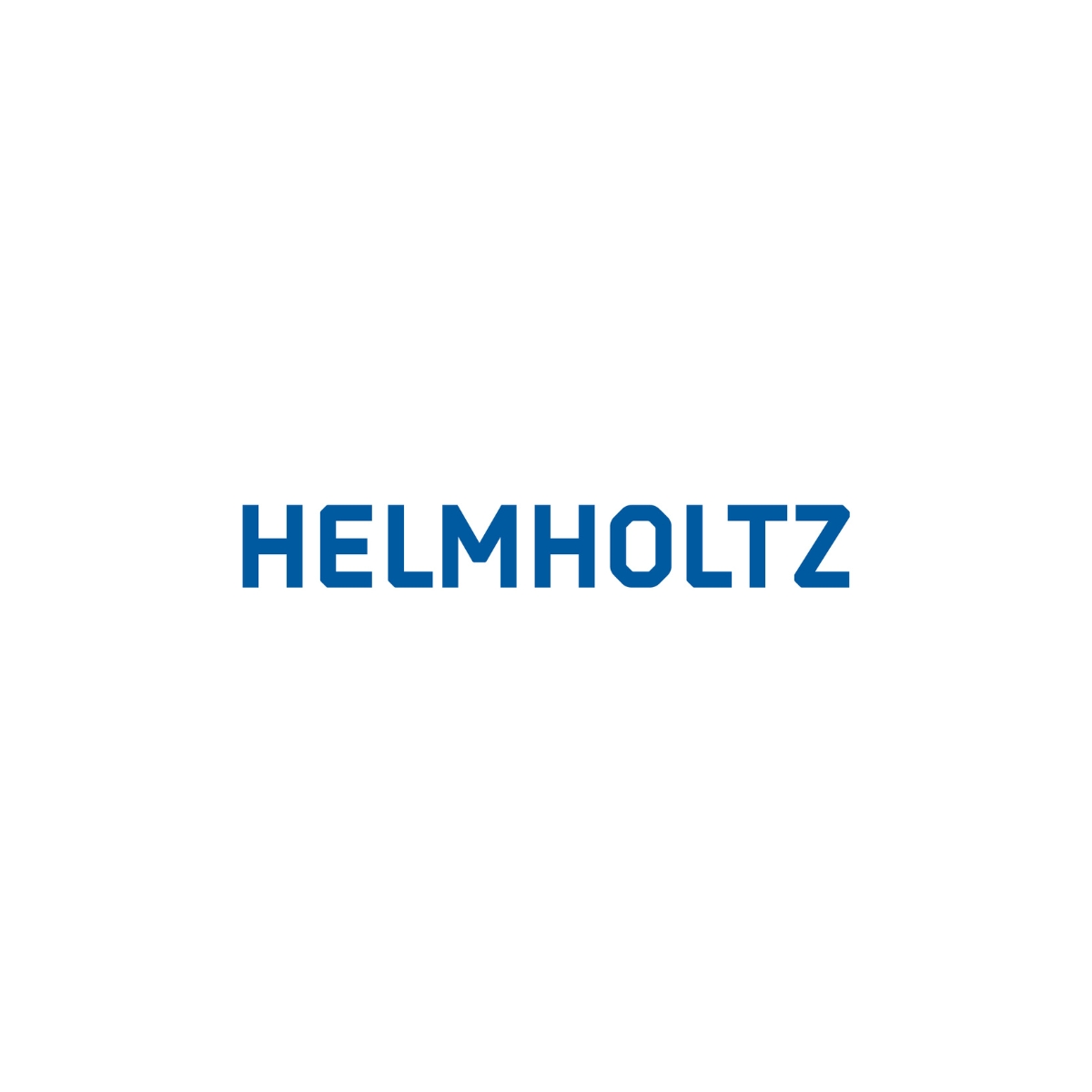
About the Helmholtz Association
The Helmholtz Association
The Helmholtz Association is Germany’s largest scientific organization. Our cross-cutting research programs connect the 18 Helmholtz research centers.
Each center has its own scientific focus areas and infrastructures. The research is thematically structured into six fields:
- Energy
- Earth & Enviroment
- Health
- Information
- Aeronautics, Space & Transport
- Matter
Helmholtz Munich is investigating how diabetes, allergies and chronic lung diseases are triggered by environmental influences. The scientists use the knowledge gained this way to develop innovative therapeutic approaches and innovative medicines. The goal of Helmholtz Munich is to achieve a better understanding of environmentally induced diseases in order to break new ground in diagnostics, therapy and prevention.
Research priorities:
- Research into widespread diseases (diabetes, allergies, chronic lung diseases)
- Analysis of the factors environment, lifestyle and genetic disposition
- Prevention and diagnostics
- Pharmaceutical research
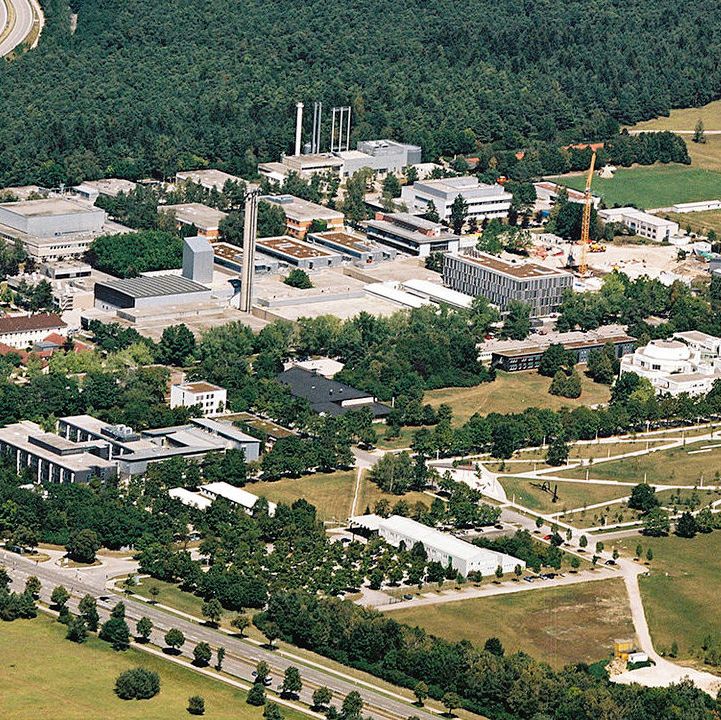
The sites
The sites
The sites of Helmholtz Munich
- Neuherberg (main site)
- Großhadern
- München (City)
- Augsburg
- Tübingen
- Hannover
- Dresden
- Leipzig
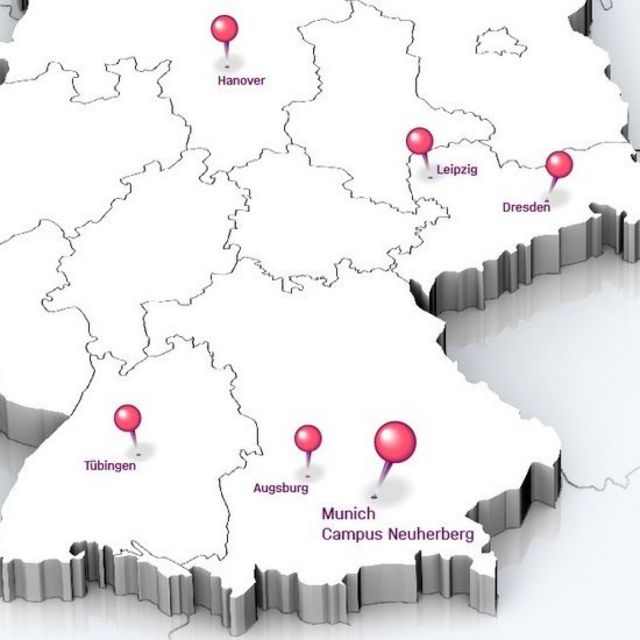
Helmholtz Munich's expertise in the field of Data Science and AI
In an interdisciplinary environment, data scientists at Helmholtz Munich develop advanced AI models and analytical tools that are crucial for solving complex health issues.
The research focus includes the integration of multi-omics data, AI-supported image processing, personalised medical applications and the investigation of environmental health interactions. Helmholtz Munich is committed to promoting health and quality of life on a broad basis through scalable data infrastructures and open science initiatives.
- Biomedical AI and machine learning
- Integration and analysis of multi-omics data
- AI-supported image processing and analysis
- Digital health and personalised medicine
- Scalable data infrastructure and open science initiatives
- AI for environmental and health interface
People from 88 nations work at Helmholtz Munich. A total of 2,515 employees work at the locations in Germany.
Application
Would you like to conduct research and work at Helmholtz Munich? Then apply now for the HIDA Mobility Program!
Please contact your potential supervisor by email before applying to propose and discuss a research project. Only submit your application after this has been clarified.
You can find more information about the application requirements here.
Note for external applicants:
If you have any questions about application formalities or organizational procedures, please contact your home institution directly.
The Hosts at Helmholtz Munich
Get to know some of the hosts at Helmholtz Munich and learn more about their respective research based on data science.
Please note: The listed hosts represent only a selection of possible supervisors.
You are also welcome to independently contact other potential hosts at the center and coordinate your participation in the HIDA Mobility Program directly with them.
If you have any questions, please send an email to: hida@helmholtz.de
Are you interested in becoming a Helmholtz host yourself and looking for support for your research project?
Then please also contact the above-mentioned email address.
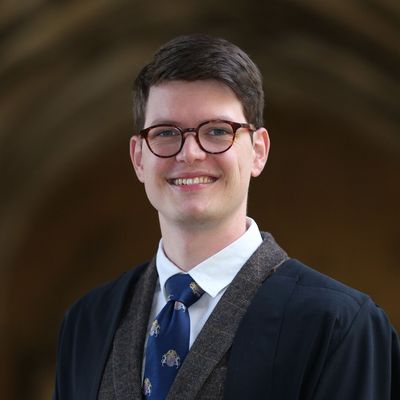
Vincent Fortuin
Efficient Learning and Probabilistic Inference for Science
Contacts
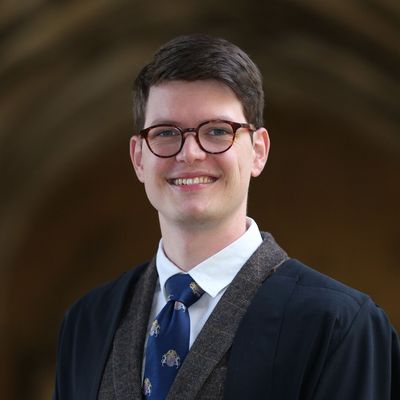
Three-sentence summary of your group's research: Our group focuses on the interface between Bayesian inference and deep learning with the goals of improving robustness, data-efficiency, and uncertainty estimation in these modern machine learning approaches. While deep learning often leads to impressive performance in many applications, it can be over-confident in its predictions and require large datasets to train. Especially in scientific applications, where training data is scarce and detailed prior knowledge is available, insights from Bayesian statistics can be used to drastically improve these models. Important research questions include how to effectively specify priors in deep Bayesian models, how to harness unlabeled data to learn re-usable representations, how to transfer knowledge between tasks using meta-learning, and how to guarantee generalization performance using PAC-Bayesian bounds.
What infrastructure, programs and tools are used in your group? We make use of high-performance CPU and GPU compute clusters (via Slurm) and regularly use deep learning frameworks (mostly PyTorch and JAX) together with the standard Python data analysis and visualization stack (scikit-learn, pandas, numpy, matplotlib, etc). On the theory side, we are interested Bayesian model selection, approximate Bayesian inference, and PAC-Bayesian generalization bounds.
What could a guest researcher learn in your group? How could he or she support you in your group? You can learn about the latest and greatest in Bayesian machine learning as well as how to train both small and (very) large deep learning models on one of the largest GPU clusters in Europe. You can apply cutting-edge ML models to a range of scientific data to push the boundaries of ML-powered scientific discovery, drug development, and understanding of health and disease. You can experience working directly at the intersection of theory and application with lots of freedom to explore either direction to suit your interests.
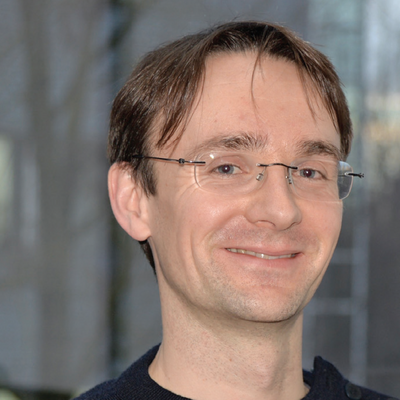
Julien Gagneur
Computational Molecular Medicine
Prof. Dr. Julien Gagneur
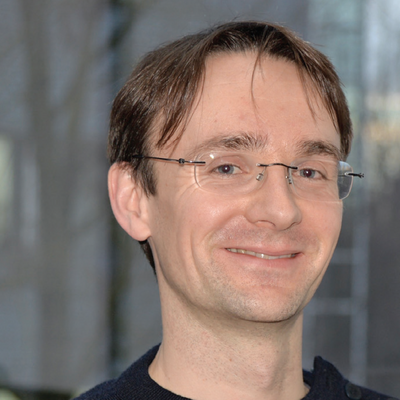
Three-sentence summary of your group's research: Our goal is an improved understanding of the genetic basis of gene regulation and its implication in diseases. To this end, we employ AI and statistical modeling of 'omic data and work in close collaboration with experimentalists. We are both based at the TUM and HMGU.
What infrastructure, programs and tools are used in your group? We work with python, ML libraries (pytorch, etc.) and R/Bioconductor.
We have developed multiple software for regulatory genomics and genetic diagnostics including :
- API and repos for trained ML models in genomics Kipoi
- sequence-based model of splicing MMSplice (GitHub)
- Base pair resolution modeling of genome-wide assays BPnet (Article regarding the topic)
- Omics based diagnosis of rare disorders: OUTRIDER, FRASER, DROP: (Protocol regarding the topic)
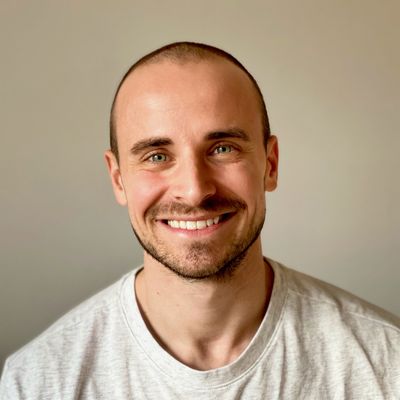
Niki Kilbertus
Ethics in Systems Design and Machine Learning
Contacts
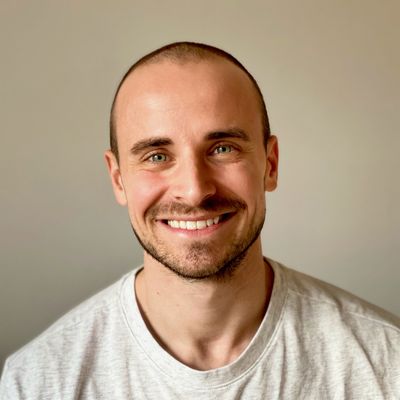
Three-sentence summary of your group's research: Our main research interests include causality, interpretable dynamical systems modeling, as well as machine learning systems that interact with humans, where we focus on reliable, fair and socially beneficial systems.
What infrastructure, programs and tools are used in your group? We make use of high performance CPU and GPU compute clusters (via Slurm) and regularly use ML frameworks (mostly jax and pytorch) together with the standard Python data analysis and visualization stack (scikit-learn, pandas, numpy, matplotlib, etc). On the theory side, we are interested in (partial) identifiability of causal effects in complex data modalities as well as in the identifiability and estimation of dynamical systems (ODEs, PDEs) from observational data.
What could a guest researcher learn in your group? How could he or she support you in your group? You can learn about the latest and greatest in causal learning and dynamic systems modeling as well as how to train both small and (very) large ML models on one of the largest GPU clusters in Europe. You can apply cutting-edge models to novel bio-medical data to push the boundaries of machine learning powered scientific discovery, drug development, and understanding of health and disease. You can experience working right at the intersection of theory and application with lots of freedom to explore either direction to whatever extent suits you.
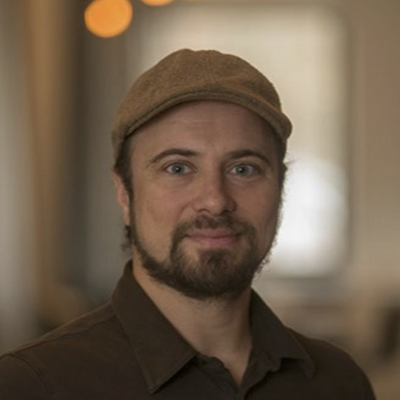
Christian L. Müller
Biomedical Statistics and Data Science
Contacts
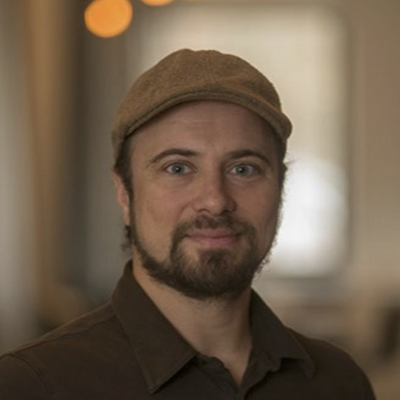
Three-sentence summary of your group's research: We do research in high-dimensional statistics, (non-)convex optimization, network inference, causal inference, and compositional data analysis with a special interest in microbiome research and microbial ecosystems. We care about sound statistical methodology and good software that is useful for answering broad statistical questions in computational biology and microbial ecology.
What infrastructure, programs and tools are used in your group? We use R, Python, (and MATLAB) for software development, and use GitHub for software deployment and versioning. Group communication is decentralized via Slack and Mattermost. We have (shared) office spaces at LMU Munich (City center) and Helmholtz Munich (in the North of Munich).
What could a guest researcher learn in your group? How could he or she support you in your group? High-dimensional statistics concepts, data analysis workflows for microbiome and microbial data, journal club covering state-of-the-art concepts ranging from experimental high-throughput biology to deep learning.
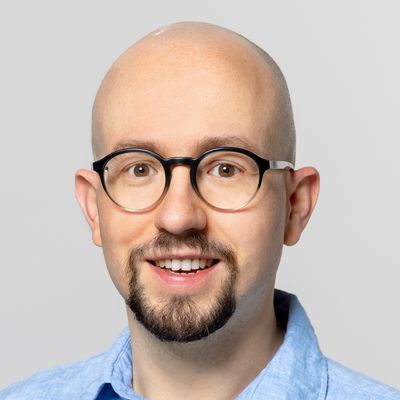
Bastian Rieck
AIDOS Lab at the Institute of AI for Health
Contacts
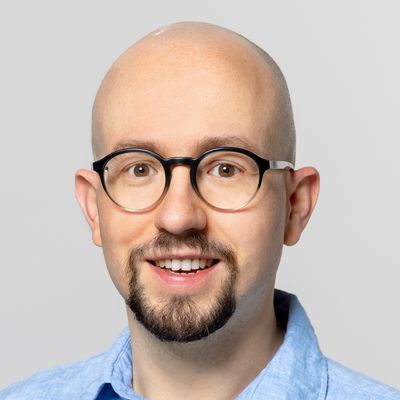
Short summary of your group's research: Our primary research interests are situated at the intersection of geometrical deep learning, topological machine learning, and representation learning. We want to make use of geometrical and topological information—also known as manifold learning—to imbue neural networks with more information in their respective tasks, leading to better and more robust outcomes. Following the dictum ‘theory without practice is empty,’ we also develop methods to address current challenges in biomedicine or healthcare applications.
What infrastructure, programs and tools are used in your group? HPC CPU/GPU cluster using Slurm, machine learning frameworks (mostly pytorch) and data analysis tools (scikit-learn, pandas, numpy) based on Python. On the theory side, we are world leaders in topological machine learning, as nascent branch of machine learning.
What could a participant of a HIDA Mobility Program learn in your group? How could he or she support you in your group? Getting to know cutting-edge AI research in geometrical deep learning or topological machine learning, including—but certainly not limited to—graph representation learning. At HMGU, we are sitting on a treasure trove of complex high-dimensional data sets, bearing the promise to substantially advance our understanding of disease-driving mechanisms, for instance. Visitors will benefit from being exposed to a unique combination of theory and practice, with the prospect of working on challenging, impactful applications. We need motivated doctoral researchers who can support our endeavour of developing the next generation of machine learning models to boldly tackle the challenges of today and tomorrow in healthcare!
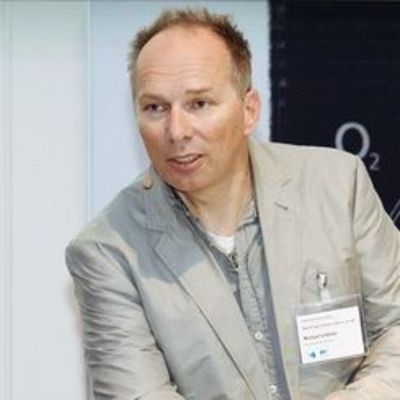
Michael Schloter
Comparative Microbiome Analysis
Contacts
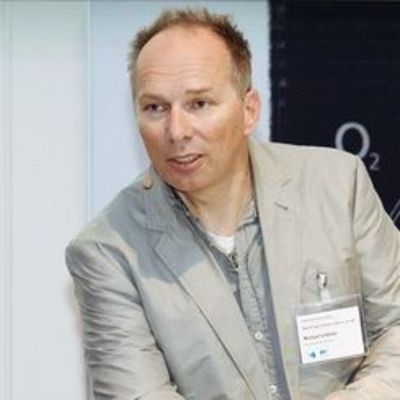
Three-sentence summary of your group's research: The human microbiome is a key component for our health. It is strongly influenced by environmental microbiota, which interact with the microbiome of barrier organs like skin or respiratory system. As a consequence, the reduced microbial diversity in the environment, resulting from climate- and global change, strongly impacts human – environment interactions, resulting in an increase in environmental diseases and infections. According to the planetary health concept the prevention of such diseases requires strategies which increase biodiversity in the environment.
We identify key microbiota from the environment, which trigger our health, develop strategies to promote the abundance of those microbiota in urban and indoor environments and analyze consequences for our health.
What infrastructure, programs and tools are used in your group?: We do next generation sequencing of microbiomes using metagneomics approaches from various sources and perform high throughput bioinformatics including KI based tools to reconstruct genomes of microborganisms.
What could a guest researcher learn in your group? How could he or she support you in your group?: In silco genome assembly of microbiota from metagenomics data and functional predications.
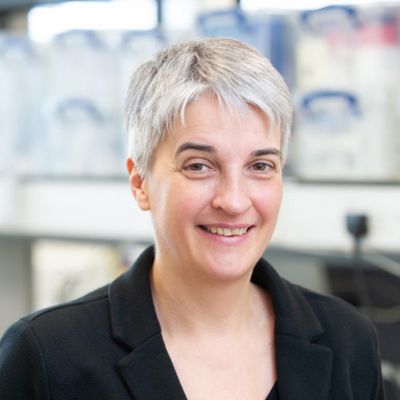
Julia Schnabel
Computational Imaging and AI in Medicine
Contacts
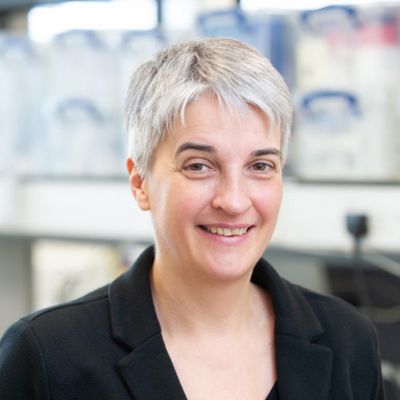
Short summary of your group's research: The Institute of Machine Learning in Biomedical Imaging (IML) focuses on research to leverage machine learning for the grand challenges in biomedical imaging in areas of unmet clinical need. Its goal is to fundamentally transform the use of imaging for diagnostics and prognostics. Novel and affordable solutions should empower clinics to make more accurate, fast and reliable decisions for early detection, treatment planning and improved patient outcome.
What infrastructure, programs and tools are used in your group? Novel and affordabel solutions should empower clinics to make more accurate, fast and reliablie decisions for early detection, treatment planning and improved patient outcome.
What could a participant of the HIDA Trainee Network learn in your group? How could he or she support you in your group? Deep learning and machine learning methods for intelligent imaging solutions, from imaging sensor to clinical applications
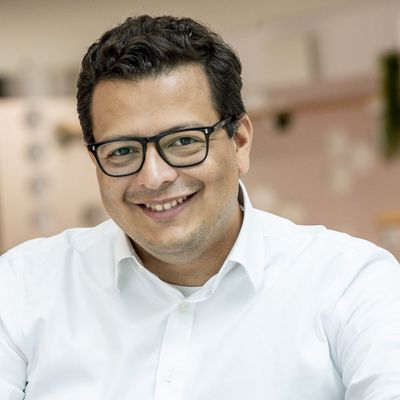
Carlos Talavera-López
Computational Immunobiology
Contacts
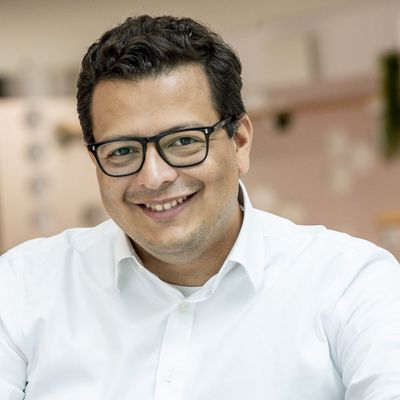
Short summary of your group's research: Our lab specialises in translational single cell biology with focus on infectious diseases. We develop integrative Carlos Talavera-LópezAI approaches to characterise cellular behaviour in health and disease with the aim of identifying diagnostic biomarkers that can be easily deployed in the clinic.
What could a participant of a HIDA Mobility Program learn in your group? How could he or she support you in your group? We are looking for a curious, dedicated scientist interested in learning to apply AI/ML methods to single cell multiome data, and to help us find ways to better communicate biological insights using novel data visualisations. Together, we will better understand the cellular social networks of inflammatory processes, and how these interactions could be potentially be used as diagnostic biomarkers.