AI-HERO Hackathon for energy-efficient AI
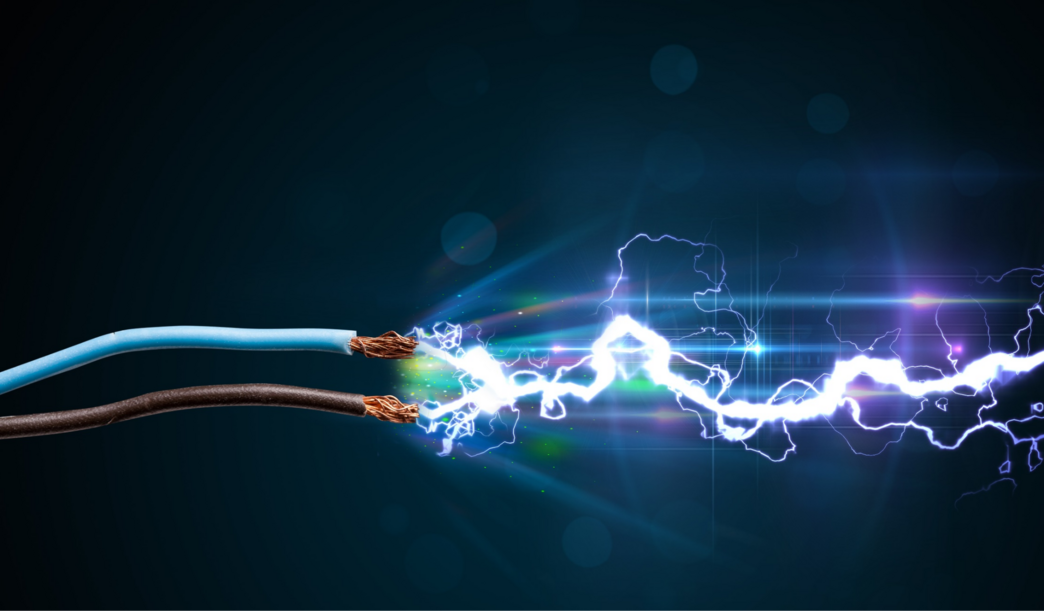
The enormous energy requirements of large IT infrastructures are an ecological challenge. The first AI-HERO Hackathon has raised awareness for the energy consumption of modern AI research and development and contributed to the wider use of "Green IT" approaches.
Artificial intelligence (AI) methods have yielded great advances in the past decade in many areas of science and technology. However, growing complexity in problems addressed is followed by an equally growing complexity in the AI models and by extension of the required resources for their computation. While modern accelerator hardware and large-scale computer clusters allow AI researchers to implement such models, the extraordinary need for electricity of these IT-infrastructures poses an increasing challenge, especially with regards to climate change and the transition to renewable energy sources.
AI-HERO is the Helmholtz Energy- and Resource-awareness Operation for Artificial Intelligence, a joint effort of members of four Helmholtz Incubator platforms from two different Helmholtz Centers. Together, the members from Helmholtz AI, Helmholtz Imaging, Helmholtz Metadata Collaboration HMC and the Helmholtz graduate school HIDSS4Health intend to raise awareness on the energy-consumption of modern day AI research and development, taking a step towards green IT.
Save Energy in AI Models
For that purpose, the team of AI-researchers from the Karlsruhe Institute of Technology KIT and the German Cancer Research Center DKFZ conducted a three-day virtual hackathon in the beginning of February 2022. The goal of the hackathon was to develop energy efficient AI models, i.e. models that use as little electricity as possible during development, training and inference.
19 Participants from ten different Helmholtz centers and affiliated universities or research institutions participated in two use-cases from the domains Energy and Health. For the health challenge, participants were faced with the problem of predicting a COVID-19 infection based on chest x-ray images. The data originated from different hospitals, collected in the context of the COVID-Net Open Initiative. The energy use case constituted an electric load forecasting task: based on three years of hourly electricity consumption from different cities the goal was to predict future electric load on a week-long forecast horizon.
Important for Evaluation: Open Science Criteria
Models were run on the Helmholtz AI Computing Resources (HAICORE) partition of KIT's supercomputer HoreKa. Utilizing Lenovo’s “XClarity Controller” and a novel SLURM plugin, HoreKa enables accurate measurement of per-node electricity consumption at a very fine resolution. The electricity consumption of all submitted processing jobs were tracked to measure the total development consumption. With the aim of propagating open-science principles, all models developed by the participants were submitted according to the FAIR (Findable, Accessible, Interoperable, Reproducible) principles for final evaluation and winner determination. These FAIR submissions were then used by the AI-HERO team to run inference on the hidden test set, allowing to independently evaluate their reproducibility as well as measure the methods performance and inference energy consumption.
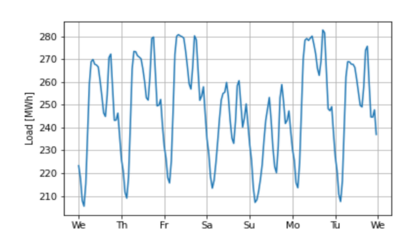
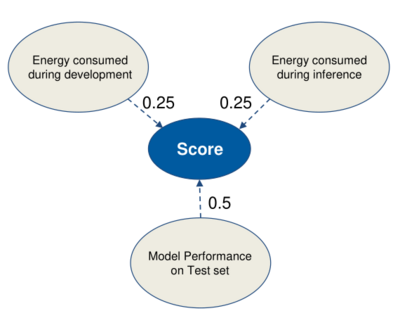
The team „Dynamic Ants“ from the Helmholtz Center Hereon won the energy use case. Using a DeepAR approach, participants were able to develop a model outperforming all others, while using only 1.74 MJ in their development process, one third of the energy consumption of the runner up team „Red Warriors“, and 36.39 kJ for the inference run. In the health use case, the team from DKFZ „Skeleton Suns“ made the race for first place. Their model based on a ResNet-18, whilst only second best in development energy consumption (22.10 MJ), provided the highest accuracy (66.43%) at only 106.64 kJ of electricity consumption for inference.
All models and scores can be found at https://ai-hero-hackathon.de/. Participants received prizes generously awarded by Lenovo, who also held a keynote presentation on the industry approach for energy efficient IT and compute hardware. We also would like to highlight the fantastic support we received from HIDA and NHR@KIT.