Why smokers, men, and the elderly tend to be more severely affected by COVID-19
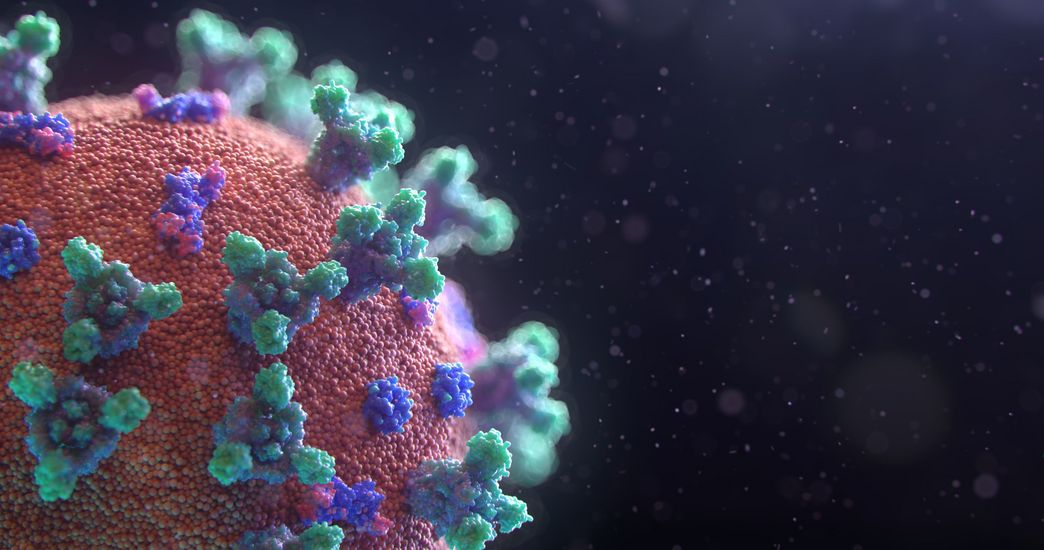
Helmholtz researchers have used single-cell analytics to find a potential molecular explanation as to why some people are at greater risk of being more severely affected by COVID-19.
It wasn’t easy to arrange an interview with you. Has the Corona virus changed your day-to-day work?
Definitely, partly because we at the Institute of Computational Biology—like everywhere else now—have been working entirely online for around four weeks. There are 150 of us here, and we conduct research on data science and biological systems in 15 different working groups. Over the past few weeks, we’ve had seminars where some 100 people held virtual discussions with each other—and it has worked amazingly well. On top of our high workload, we recently began research on a wide range of COVID-19 projects, and we’re working flat out on this. Many researchers around the world are hardly sleeping at all right now due to the critical relevance of this research, and it’s the same for us.
What is your research contributing to the fight against the Corona virus?
Epidemiological analyses in recent weeks have shown that the SARS-CoV-2 coronavirus doesn’t affect everyone equally — we’re seeing a high degree of heterogeneity in infections among the population. But why are men more vulnerable than women; why do more smokers get sick but almost no children? Basically, we’re getting to the bottom of this phenomenon in molecular biological terms. Our current work on COVID-19 in the Human Cell Atlas Lung Biological Network has found that various groups exhibit molecular differences in the expression of individual genes of relevance to the infection. This suggests that these differences influence the course of the disease and the likelihood of being infected, because they correspond to clinical observations. But it’s not easy to test these correlations, because you can’t just upregulate genes in people to see what happens.
What exactly have you found out?
So far, studies have shown that SARS-CoV-2 enters human cells by attaching to what are called ACE2 receptors. Proteases such as TMPRSS2 facilitate the attachment process. The role of ACE2 receptors is normally to modify hormones so they regulate the constriction of blood vessels. In the case of an infection, however, they can also cause tissue damage or pulmonary edema. We looked at datasets for various people based on these results. And our initial work, which was published in two recent papers, succeeded in identifying the human cells in which the ACE2 receptor and associated TMPRSS2 protease are expressed in the first place. These primarily include certain types of cells in the alveoli.
To what extent are these results relevant to the pandemic?
The results form the basis for an important study we’re currently working on; the manuscript will be published on a preprint server next week. In the study, we used the data to conduct cell-specific analyses as to how the expression of these receptors and the proteases is influenced by age, gender, and whether an individual is a smoker or not. To do this, we examined which tissue expresses ACE2 and the protease at the same time — and which cells therefore have a higher risk of infection than others. We found that the ACE2 receptor is more strongly expressed, for example, in epithelial cells in the respiratory tracts of older people, men, and smokers. We’re also seeing that, in certain types of cells, the proteases needed to infect a cell are also expressed more strongly in older people and men. These results correspond to clinical observations of COVID-19 patients. In other words, they could explain the molecular basis behind the differences in the way the disease progresses between age groups, genders, and smokers: The heterogeneous nature of coronavirus infections in the population is likely due to the fact that gene activity for these two proteins is higher among those affected — in addition to factors such as differences in the strength of their immune systems, weight, and underlying medical conditions. This makes it easier for SARS-CoV-2 to enter the cells, and these individuals are more likely to become infected.
How can these results be used to fight the Corona virus?
Coronavirus is continuing to spread, but it isn’t spreading consistently. The fact that coronavirus enters cells via the ACE2 receptor and TMPRSS2 protease was known before our study. But we didn’t know why the virus doesn’t spread on a uniform basis. We’ve found a potential molecular explanation for the uneven way it’s spreading, but this is based on the mechanism that had already been identified. Our work shows that the expression of the receptor and two proteases is the only factor that can explain the variation in the distribution of infections. This provides further indication that these genes represent interesting targets for treatment approaches, because they also seem to play an important role in predicting how the disease will progress.
Which specific datasets did you use?
We’ve been working as part of what’s known as the Human Lung Atlas for a number of years. The Lung Atlas is part of the Human Cell Atlas (HCA), an international consortium aiming to create a sort of Google Maps for the human body. Its working groups are collecting datasets from a broad spectrum of test subjects for this purpose. The consortium wants to characterize each cell, pinpoint its location, and understand how it interacts with other cells. If the consortium succeeds in mapping all cells and tissues at all points in time, this will make it possible to answer a vast number of complex questions relating to human health as well as diagnosing, monitoring, and treating diseases. There are already huge datasets for all organs and tissues from test subjects who are both healthy and diseased. The Human Lung Atlas on its own currently has the largest existing dataset of healthy lung cells; it comprises cells from 164 donors. We can use these to analyze millions of gene sequences, from infants to 80-year-olds.
What information do data from healthy people provide with respect to coronavirus infections?
We create profiles of the molecular state of all lung cells in order to identify the mechanisms involved when people are healthy or sick. In this context, data from just a few healthy people offer us millions of new pieces of information — including how diseases develop. Just think about the fact that a transcriptome — that’s the complete set of genes transcribed in a cell at a certain point in time — describes around 25,000 activated genes on its own. This makes it very challenging to find a pattern or a deviation in lung cells from only five test subjects. Prior to the SARS-CoV-2 outbreak, we worked with “small” datasets like these from the Lung Atlas. But the pandemic has given this work a new sense of urgency. All 18 working groups in the Lung Atlas have shared their datasets from a total of 250 subjects to achieve rapid and conclusive results regarding heterogeneity, for example. This has resulted in what’s likely the largest dataset of lung cells anywhere in the world right now. Analyses of these vast volumes of data have indicated that the genes of men, smokers, and older people exhibit a higher level of activity for the receptor and the proteases — and that these individuals are therefore more likely to become sick if they come into contact with the virus.
What data science methods are used to help analyze the data?
We use single-cell analytics as the basis for the data science. This term covers various methods that deliver comprehensive, precise data on the molecular character of individual cells and the way they work. These methods make it possible, for example, to read the activity of genes in the individual lung cells via gene sequencers and classify the changes in the proteins. Single-cell analytics have revolutionized the field of molecular biology. They make it possible to record complex biological processes, events, and developments precisely for single cells — and they’re capable of doing this for thousands or even millions of individual cells at a time. Data science also allows us, firstly, to deliver detailed statistical descriptions of high-dimensional states cells can assume. Secondly, it enables us to calculate how these cell states change, for example, due to a virus or taking a drug.
But your work goes far beyond single-cell analytics.
Data sequencing is just the beginning, of course. The vast volumes of data from people can’t be evaluated anymore; furthermore, we work with millions of different cells from different cell types and various labs. This results in an incredible degree of variation. So, what we need first of all is a uniform definition of what exactly is a cell from the upper or lower respiratory tract. We would then need to place the data in a context where they’re linked together in a meaningful way. Methods like machine learning and artificial intelligence would be helpful here. Developing algorithms allows us to identify structures in the data and the underlying biological mechanisms.
What is your take on the past few weeks?
Given the incredible challenges science is facing due to the pandemic, I’ve been happy to see the innovative collaboration that’s taking place. The best people from around the world are working on this. In the Lung Atlas, for example, all of the groups were previously focused on their own work. Now we’re sharing data, even between groups that are competing with each other. Our own interests have taken a back seat, and our shared goal is to understand the pandemic and get a handle on it. I never expected to see such a positive dynamic develop in the group or such a rapid exchange of information. There’s no question that this is one positive outcome of the coronavirus crisis.
Interview: Beate Wagner