Nach einer intensiven Ausbildung an der Schnittstelle zwischen Information & Data Science und einer der sechs Helmholtz Forschungsdomänen sind die Absolventen unserer Research Schools bereit für die Herausforderungen von morgen. Lernen Sie die Graduierten hier kennen.
DASHH
Think Big: Mit dem stärksten Röntgenstrahl der Welt und Data Science werden im hohen Norden die Geheimnisse der Materie entschlüsselt. Die Data Science in Hamburg Helmholtz Graduate School for the Structure of Matter (DASHH) bringt dafür neun Partnerinstitutionen zusammen.
HEIBRiDS
In die Tiefen des Universums blicken oder Erdbeben vorhersagen: An der Helmholtz Einstein International Berlin Research School in Data Science (HEIBRiDS) hat die Beschäftigung mit Data Science einen weiten Horizont.
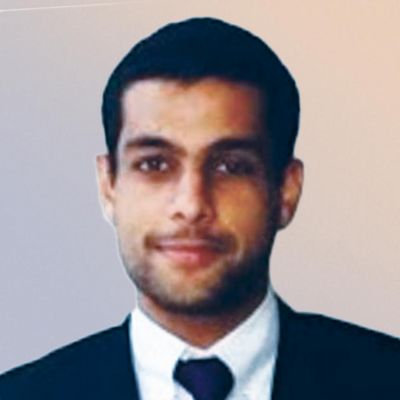
Siddhant Agarwal
Alumnus HEIBRiDS
Kontakt
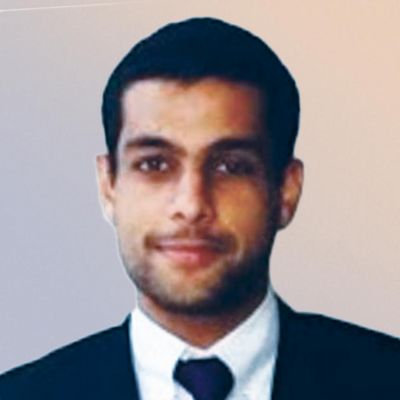
Supervisors:
Studying how rocky planets like Mercury, Venus, the Earth and Mars evolve over billions of years requires detailed modelling of mantle convection, the main driver of planetary evolution. The mantle - sandwiched between the crust and the core - behaves like a highly viscous fluid over geological time scales and hence can be quantified through equations describing conservation of mass, momentum and energy. These non-linear partial differential equations are typically solved numerically using fluid dynamics codes. However, the parameters and initial conditions to these equations are poorly known. Whereas certain outputs of the simulations (numerically solved equations) can be "observed'' via spacecraft missions and used to constrain key parameters and initial conditions, thus elucidating the basic physics and evolution of planets. Since each simulation can take from several hours to weeks to run, varying parameters extensively and repeatedly is often impractical. We aim to overcome this computational bottleneck by learning the mapping between parameters and observables through a combination of state-of-the-art geodynamic modelling, machine learning and high-performance computing.
Full-length publications
- S. Agarwal, N. Tosi, D. Breuer, S. Padovan, P. Kessel, and G. Montavon (2020). A machine-learning-based surrogate model of Mars’ thermal evolution. Geophysical Journal International, 222(3), 1656-1670. https://doi.org/10.1093/gji/ggaa234
- S. Agarwal, N. Tosi, P. Kessel, S. Padovan, D. Breuer, and G. Montavon (2021). Towards constraining Mars’ thermal evolution using Machine Learning. Earth and Space Science.https://doi.org/10.1029/2020EA001484
- S. Agarwal, N. Tosi, P. Kessel, D. Breuer, and G. Montavon (2021). Deep learning for surrogate modeling of two-dimensional mantle convection. Physical Review Fluids, 6, 113801. https://doi.org/10.1103/PhysRevFluids.6.113801
Conference presentations
- S. Agarwal, N. Tosi, D. Breuer, S. Padovan, P. Kessel, and G. Montavon. Unravelling interior evolution of terrestrial planets using Machine Learning. (Oral presentation), Artificial Intelligence in Astronomy at ESO, Garching, Germany, 22-26 July 2019.
- S. Agarwal, N. Tosi, D. Breuer, P. Kessel, and G. Montavon. Using machine learning to predict 1D steady-state temperature profiles from compressible mantle convection simulations. (Oral presentation), 72nd Annual Meeting of the APS Division of Fluid Dynamics, Seattle, USA, 23-26 November 2019.
- S. Agarwal, N. Tosi, P. Kessel, D. Breuer, S. Padovan, and G. Montavon. Mars’ thermal evolution from machine-learning-based 1D surrogate modelling. (Oral presentation), EGU General Assembly, Online, 7 May 2020.
- S. Agarwal, N. Tosi, P. Kessel, D. Breuer, S. Padovan, and G. Montavon. Learning high dimensional surrogates from mantle convection simulations. (Oral presentation), 73rd Annual Meeting of the APS Division of Fluid Dynamics, Online, 23 November 2020.
- S. Agarwal, N. Tosi, P. Kessel, S. Padovan, D. Breuer, and G. Montavon. Towards constraining Mars' thermal evolution using machine learning. (PICO presentation), EGU General Assembly, Online, 19-30 Apr 2021. https://doi.org/10.5194/egusphere-egu21-4044
- S. Agarwal, N. Tosi, P. Kessel, D. Breuer, and G. Montavon. Deep learning for surrogate modelling of 2D mantle convection, oral presentation. German-Swiss Geodynamics Workshop 2021, Bad Belzig, 29 Aug–1 Sep 2021.
- S. Agarwal, N. Tosi, P. Kessel, D. Breuer, and G. Montavon. Deep learning for surrogate modelling of 2D mantle convection. (Oral presentation), European Planetary Science Congress 2021, Online, 13–24 Sep 2021. https://doi.org/10.5194/epsc2021-218
- S. Agarwal, N. Tosi, P. Kessel, D. Breuer, and G. Montavon. Deep learning for surrogate modelling of 2D mantle convection. (Oral presentation), The 74th Annual Meeting of the Division of Fluid Dynamics, Online, 21-23 Nov 2021.
- S. Agarwal, N. Tosi, P. Kessel, D. Breuer, and G. Montavon. A machine learning framework for constraining mantle convection parameters. (Oral presentation), American Geophysical Union Fall Meeting, New Orleans, 13-17 Dec 2021.
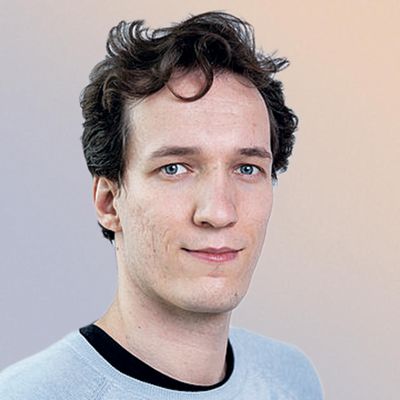
Ivo Daniel
Alumnus HEIBRiDS
Kontakt
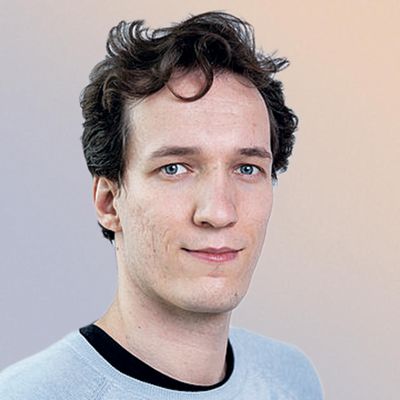
Supervisors:
Water losses are one of the main consequences of infrastructure failures in water distribution networks. While background leakages and pipe bursts in well maintained systems generally amount to only 3-7% of the total water supplied, they can account for more than 50% for poorly maintained networks worldwide. Methods that support prompt detection and accurate localization of leakages are crucial to help water utilities implement timely mitigation measures and avoid unnecessary loss of water.
Leakages can be classified as one type of anomaly occurring in water distribution networks. Broadly speaking, methods for their detection are referred to as anomaly detection methods. Anomaly detection methods have been studied extensively in the context of intrusion into information networks, and applied to water distribution networks in the similar context of cyber-attacks on SCADA systems. However, most current approaches for leakage detection rely on in-situ, engineering-based technology, while the development and application of data-driven approaches still poses several research challenges.
The goal of this project is to develop data-driven methods that are capable of detecting leakages in water distribution networks in real-time. As this research originated in an international competition, the BattLeDIM - Battle on Leakage Detection and Isolation Methods (http://battledim.ucy.ac.cy), its foundation is built upon the BattLeDIM dataset, inferring that the focus is put on the analysis of high resolution pressure data provided by a network of sensors located throughout the system. Data Mining and Machine Learning frameworks offer a wide range of opportunities for the analysis of this data and are comparatively utilized to identify and localize leakages as the primary type of anomaly.
The development of a data-driven methodology for leakage detection opens up the possibility to be extended to other applications in water distribution systems, including real-world systems, and assess their transferability to other problems where anomaly detection may be beneficial. The development of such an effective, decentralized framework implies the opportunity for additional research on IoT sensors, their communication interface, and their placement. Further research may be targeting wastewater systems to evaluate whether the developed methods may be cost-effectively transferred or adapted.
Full-length publications
- I. Daniel, J. Pesantez, S. Letzgus, M.A. Khaksar Fasee, F. Alghamdi, E. Berglund, G. Mahinthakumar, and A. Cominola, (2022). A sequential pressure-based algorithm for data-driven leakage identification and model-based localization in water distribution networks. Journal of Water Resources Planning and Management, 148, 6. DOI:10.1061/(ASCE)WR.1943-5452.0001535
- I. Daniel, J. Pesantez, S. Letzgus, M.A.K. Fasaee, F. Alghamdi, K. Mahinthakumar, E. Berglund, and A. Cominola (2020). A high-resolution pressure-driven method for leakage identification and localization in water distribution networks. Zenodo. http://doi.org/10.5281/zenodo.3924632
Conference presentations
- I. Daniel, N. Ajami, A. Castelletti, D. Savic, R. Stewart, M. Becker, and A. Cominola. How is digital transformation impacting the water utility sector? - Insights from a worldwide online utility survey. (Oral presentation), EGU General Assembly 2021, Online, 19–30 Apr 2021. https://doi.org/10.5194/egusphere-egu21-12540
- I. Daniel, J. Pesantez, S. Letzgus, M.A. Khaksar Fasee, F. Alghamdi, E. Berglund, G. Mahinthakumar, and A. Cominola. Leakage identification and localization on the BattLeDIM dataset: testing and performance evaluation of a high-resolution pressure-driven method. (Oral presentation), World Environmental & Water Resources Congress, Online, 7-11 Jun 2021.
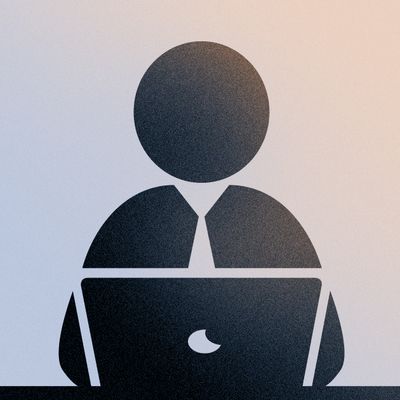
Peter Hirsch
Alumnus HEIBRiDS
Kontakt
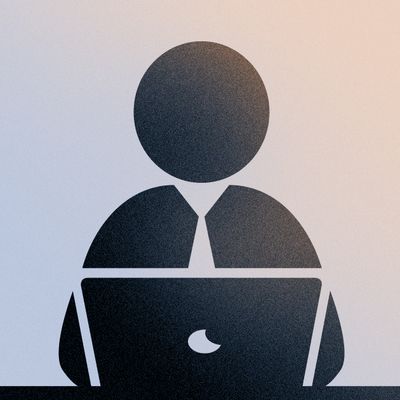
Supervisors:
Many experiments in biology require a large number of samples to allow for conclusive statements. It is thus of utmost importance to reduce the cost per sample as much as possible. This cost can be both in terms of money and time. If a single sample takes multiple hours and one needs hundreds or thousands of samples, the undertaking quickly becomes infeasible. Every significant reduction in manual work can thus make an infeasible project feasible.
In this project we want to study the effect of changes (mutations) in the genome - some of them lethal - on the development of C. elegans embryos and its cell lineage. Yet this requires the tracking of all their cells over time and through cell divisions. While some automatic methods to do this exist, all require several hours of manual curation per sample to get an error-free result.
To overcome this, we are developing new tracking algorithms employing modern machine learning methods applied to volumetric time series data (3d+time).
C. elegans provides us with a prime example. Its development is stereotypical, each wild type (without mutations) organism exhibits the identical number of cells and division pattern. This makes it possible to automatically pin-point both errors in the tracking algorithm and true changes in the development due to mutations.
Analyzing these changes will help us to expand our understanding of the gene regulatory networks induced by the genome, and how they are affected by mutations, a key challenge of developmental biology.
Full-length publications
- A. Krull*, P. Hirsch*, C. Rother, A. Schiffrin, and C. Krull (2020). Artificial-intelligence-driven scanning probe microscopy. (*shared first) Commun Phys 3, 54. https://doi.org/10.1038/s42005-020-0317-3
- P. Hirsch, and D. Kainmueller (2020). An auxiliary task for learning nuclei segmentation in 3D microscopy images. Proceedings of Machine Learning Research 121(304), 318.
- L. Mais*, P. Hirsch*, and D. Kainmueller (2020). PatchPerPix for instance segmentation. (*shared first) In: Vedaldi A., Bischof H., Brox T., Frahm JM. (eds) Computer Vision – ECCV 2020. ECCV 2020. Lecture Notes in Computer Science, vol 12370. Springer, Cham. https://doi.org/10.1007/978-3-030-58595-2_18
- J.L. Rumberger*, X. Yu*, P. Hirsch*, M. Dohmen*, V.E. Guarino*, A. Mokarian, L. Mais, J. Funke, and D. Kainmueller (2021). How Shift equivariance impacts metric learning for instance segmentation. (*shared first) In Proceedings of the IEEE/CVF International Conference on Computer Vision (ICCV).
-
L. Mais, P. Hirsch, C. Managan, K. Wang, K. Rokicki, R.R. Svirskas, B.J. Dickson, W. Korff, G.M. Rubin, G. Ihrke, G.W. Meissner, and D. Kainmueller (2021). PatchPerPixMatch for Automated 3d Search of Neuronal Morphologies in Light Microscopy. bioRxiv. https://doi.org/10.1101/2021.07.23.453511
- P. Hirsch, C. Malin-Mayor, A. Santella, S. Preibisch, D. Kainmueller, and J. Funke (2022). Tracking by Weakly-Supervised Learning and Graph Optimization for Whole-Embryo C. elegans lineages. In: Wang, L., Dou, Q., Fletcher, P.T., Speidel, S., Li, S. (eds) Medical Image Computing and Computer Assisted Intervention – MICCAI 2022. Lecture Notes in Computer Science, vol 13434. Springer, Cham. https://doi.org/10.1007/978-3-031-16440-8_3
- J.L. Rumberger, E. Baumann, P. Hirsch, A. Janowczyk, I. Zlobec and D. Kainmueller (2022). Panoptic segmentation with highly imbalanced semantic labels. 2022 IEEE International Symposium on Biomedical Imaging Challenges (ISBIC), p. 1-4. https://doi.org/10.1109/ISBIC56247.2022.9854551
- P. Hirsch, L. Epstein, and L. Guignard (2020). Chapter 20 - Mathematical and bioinformatic tools for cell tracking. In: M. Schnoor, L-M. Yin, S.X. Sun (eds) Cell Movement in Health and Disease, Academic Press, 2022, p. 341-361, ISBN 9780323901956. https://doi.org/10.1016/B978-0-323-90195-6.00013-9
- C. Malin-Mayor, P. Hirsch, L. Guignard, K. McDole, Y. Wan, W.C. Lemon, D. Kainmueller, P.J. Keller, S. Preibisch, and J. Funke (2023). Automated reconstruction of whole-embryo cell lineages by learning from sparse annotations. Nat Biotechnol 41, 44–49. https://doi.org/10.1038/s41587-022-01427-7
Conference presentations
- P. Hirsch and D. Kainmueller. An Auxiliary Loss for Learning Nuclei Segmentation in 3D Microscopy Images. (Poster presentation), Frontiers in Imaging Science II, Janelia Research Campus, 1-4 May 2019.
- P. Hirsch, J.L. Rumberger, X. Yu, M. Dohmen, V.E. Guarino, A. Mokarian, L. Mais, J. Funke, and D. Kainmueller. What can go wrong with tile&stitch? (Poster presentation), Crick Bioimage Analysis Symposiym, London, U.K., 22-23 November 2021.
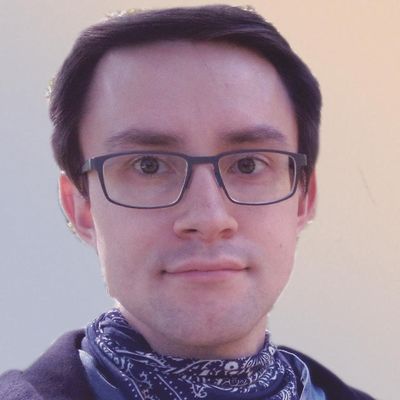
Thorren Kirschbaum (né Gimm)
Alumnus HEIBRiDS
Kontakt
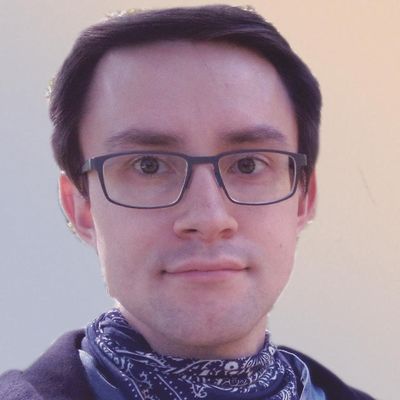
Supervisors:
The world is facing an ever-increasing demand for energy and resources as the scarcity of resources and the pollution of the environment are forcing us to redesign the foundations of global economies. New methods of producing „green“ energy and chemical base materials are in heavy demand. Hydrogen generated from environmentally neutral processes has the potential to provide both: a zero-emission energy carrier and chemical feedstock. However, the processes needed for the „clean“ production of hydrogen are not yet economically viable on a large scale. This project explores a novel way to generate hydrogen by splitting water into its elements, H2 and O2.
A key goal of modern energy research is to find efficient ways to achieve this splitting. The process relies on the efficient reduction of water hydrogen and oxidation of water oxygen. It has long been known that electrons solvated in water are the ideal, most direct agents to induce this reduction, but typically generating them has required harsh reaction conditions that have limited this approach. Very recently, however, a relatively mild production process was experimentally achieved using hydrogen-covered nanodiamonds illuminated by light. The process is conceived as follows: (1) The nanodiamond is excited and an electron moves towards the particle‘s surface, which permits (2) the electron to transfer into the interfacial water and (3) to move into the solution, where (4) it eventually reacts. Still we are far from understanding the precise mechanism underlying this effect, which is a key to improving and scaling-up its performance.
To learn more about the electron generating processes, we plan to model the electron transfer and solvation dynamics (described in (1)-(3) above) using coupled multi-scale electron and nuclear dynamics methods. Additionally, we will optimize the reaction paramters through a combination of quantum chemistry and machine learning. Steps (1-2) require intricate quantum electron dynamics (ED) calculations, which can be done only for a small number of molecular conformations. Steps (2-3) rely on electron hopping/transfer rates in conjunction with statistical interface physics and simulations of the molecular dynamics (MD) of the diamond/water interface. Deep learning will be used to approximate results from ED to parametrize MD simulations and create a time-dependent multi-physics description of the full process. This should give us a significantly better understanding of the system. Subsequently, we will use methods of optimal control to find the most efficient electron solvation process, in which the optimal control parameters are surface decoration, UV pulse (intensity, duration, shape), and temperature. Furthermore, the nanodiamonds‘ electronic properties will be optimized for excitation by sunlight through an approach that combines density functional theory (DFT) and supervised machine learning.
Full-length publications
- J. Ren, L. Lin, K. Lieutenant, C. Schulz, D. Wong, T. Gimm, A. Bande, X. Wang, and T. Petit (2020). Role of dopants on the local electronic structure of polymeric carbon nitride photocatalysts. Small Methods 2000707. https://doi.org/10.1002/smtd.202000707
- T. Kirschbaum, T. Petit, J. Dzubiella, and A. Bande (2022). Effects of oxidative adsorbates and cluster formation on the electronic structure of nanodiamonds. J. Comput. Chem., 43,13, 923-929. https://doi.org/10.1002/jcc.26849
- F. Buchner, T. Kirschbaum, A. Venerosy, H. Girard, J-C. Arnault, B. Kiendl, A. Krueger, K. Larsson, A. Bande, T. Petit, and C. Merschjann (2022). Early dynamics of the emission of solvated electrons from nanodiamonds in water. Nanoscale, 14,17188-17195. https://doi.org/10.1039/D2NR03919B
- K. Palczynski, T. Kirschbaum, A. Bande, and J. Dzubiella (2023). Hydration Structure of Diamondoids from Reactive Force Fields. J. Phys. Chem. C, 127, 6, 3217–3227. https://doi.org/10.1021/acs.jpcc.2c07777
- T. Kirschbaum, B. von Seggern, J. Dzubiella, A. Bande, and F. Noé (2023). Machine Learning Frontier Orbital Energies of Nanodiamonds. J. Chem. Theory Comput. https://doi.org/10.1021/acs.jctc.2c01275
Conference presentations
- T. Gimm, X. Wang, K. Palczynski, A. Bande, and J. Dzubiella. Nanodiamond-adsorbate interactions studied by DFT. (Poster presentation), Bunsen-Tagung 2021 - Multi-scale modelling & physical chemistry of colloids, Online, 10-12 May 2021.
- T. Gimm, X. Wang, K. Palczynski, A. Bande, and J. Dzubiella. Nanodiamond-adsorbate interactions studied by DFT. (Poster presentation), 57th Symposium of Theoretical Chemistry, Online, 20-24 September 2021.
- T. Kirschbaum, B. von Seggern, J. Dzubiella, A. Bande and F. Noé. Machine Learning Frontier Orbital Energies of Nanodiamonds. (Poster presentation), 58th Symposium of Theoretical Chemistry, Heidelberg, Germany, 18-22 September 2022.
- T. Kirschbaum, B. von Seggern, J. Dzubiella, A. Bande, and F. Noé. Machine Learning Frontier Orbital Energies of Nanodiamonds. (Oral presentation), Asia Pacific Conference of Theoretical and Computational Chemistry, Quy Nhon, Vietnam, 19-23 February 2023.
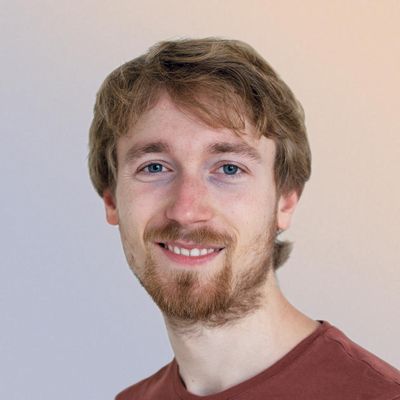
Jannes Münchmeyer
Alumnus HEIBRiDS
Kontakt
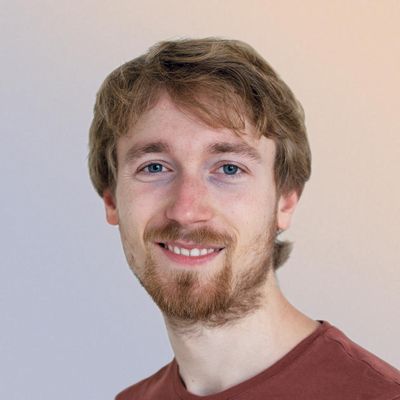
Supervisors:
Earthquakes count among the largest natural threats to humans. The current state of research suggests that it will not be possible to predict earthquakes reliably anytime in the near future. What is possible, on the other hand, is to provide reliable early warning in the context of ongoing earthquakes. The goal is to provide warnings a few seconds before strong shaking occurs. Such warnings can trigger automatic reactions, like stopping trains, or alert humans early enough to allow them to seek cover.
Usually, early warnings are based on recording the early, relatively weak shaking caused by an earthquake, inferring its size, and then predicting the level of shaking to follow. However, a look at the physics behind earthquakes reveals a crucial issue in attempting to make such predictions. An earthquake emits seismic waves from a rapidly growing rupture between two tectonic plates. These ruptures can traverse distances of tens or even hundreds of kilometers, and consequently, even when a rupture grows quickly, it might take tens of seconds or even minutes for the full rupture to occur.
There is no scientific consensus on how accurately the size of an earthquake can be assessed at what time during an ongoing rupture. There are two basic positions among experts: one holds that the size of an earthquake can be accurately predicted from its onset or during the first few seconds, and the other that accurate assessment is impossible until the rupture is largely finished. Which of these positions is correct will have a profound impact on the potential of early warning methods: if the earthquake's size can only be determined at the end of the rupture, then only short warning times – if any at all – will be possible.
In this PhD project, I am taking a novel, data-driven approach to the question of predictability. Using machine learning, I will build real-time assessment systems to predict the size of an event during an ongoing rupture. If we can design a model that can accurately assess the size of an earthquake from its first seconds, this will be a demonstration that ruptures can be feasibly predicted. A further step will be to integrate our real-time assessment model into earthquake early warning systems, to improve their performance with our state-of-the-art estimation methodology.
Full-length publications
- L. Weber, J. Münchmeyer, T. Rocktäschel, M. Habibi, and U. Leser (2019). HUNER: Improving biomedical NER with pretraining. Bioinformatics, 36(1), 295-302. 10.1093/bioinformatics/btz528
-
L. Weber, P. Minervini, J. Münchmeyer, U. Leser, and T. Rocktäschel (2019). NLProlog: Reasoning with weak unification for question answering in natural language. In Proceedings of the 57th Annual Meeting of the Association for Computational Linguistics, 6151-6161. 10.18653/v1/P19-1618
-
J. Münchmeyer, D. Bindi, C. Sippl, U. Leser, and F. Tilmann (2019). Low uncertainty multi-feature magnitude estimation with 3D corrections and boosting tree regression: Application to North Chile. Geophysical Journal International, 220(1), 142-159. doi.org/10.1093/gji/ggz416
-
J. Münchmeyer, D. Bindi, U. Leser, and F. Tilmann (2020). The transformer earthquake alerting model: A new versatile approach to earthquake early warning. Geophysical Journal International, ggaa609. doi.org/10.1093/gji/ggaa609
-
L. Weber, M. Sänger, J. Münchmeyer, M. Habibi, U. Leser, and A. Akbik (2021). HunFlair: An easy-to-use tool for state-of-the-art biomedical Named Entity Recognition. Bioinformatics, btab042, https://doi.org/10.1093/bioinformatics/btab042
-
J. Münchmeyer, D. Bindi, U. Leser, and F. Tilmann (2021). Earthquake magnitude and location estimation from real time seismic waveforms with a Transformer Network. Geophysical Journal International, 226(2), 1086-1104. https://doi.org/10.1093/gji/ggab139
-
W.J. Foster, G. Ayzel, J. Münchmeyer, T. Rettelbach, N. Kitzmann, T.T. Isson, M. Mutti, and M. Aberhan (2021). Machine learning identifies ecological selectivity patterns across the end-Permian mass extinction. Paleobiology, 1-15. https://doi.org/10.1017/pab.2022.1
-
L. Weber, S. Garda, J. Münchmeyer, and U. Leser (2021). Extend, don’t rebuild: Phrasing conditional graph modification as autoregressive sequence labelling. In Proceedings of the 2021 Conference on Empirical Methods in Natural Language Processing, 1213–1224.
-
J.* Münchmeyer, J.* Woollam, F. Tilmann, A. Rietbrock, D. Lange, ..., and H. Soto (2021). Which picker fits my data? A quantitative evaluation of deep learning based seismic pickers. Journal of Geophysical Research: Solid Earth, 127, 1, e2021JB023499. https://doi.org/10.1029/2021JB023499 *Equal contribution
-
K. Singh, J. Münchmeyer, L. Weber, U. Leser and A. Bande (2022). Graph Neural Networks for Learning Molecular Excitation Spectra. J. Chem. Theory Comp., 18, 7, 4408-4417. DOI: 10.1021/acs.jctc.2c00255
-
J.* Woollam, J.* Münchmeyer, F. Tilmann, A. Rietbrock, D. Lange, ..., and H. Soto (2022). SeisBench - A Toolbox for Machine Learning in Seismology. Seismological Research Letters, 93(3), 1695–1709. https://doi.org/10.1785/0220210324 *Equal contribution
-
J. Münchmeyer, U. Lesera and F. Tilmann (2022). A probabilistic view on rupture predictability: All earthquakes evolve similarly. Geophysical Research Letters, 49, 13, e2022GL098344. https://doi.org/10.1029/2022GL098344
Conference presentations
- J. Münchmeyer, D. Bindi, C. Sippl, and F. Tilmann. Increasing magnitude scale consistency by combining multiple waveform features through machine learning. (Oral presentation), EGU General Assembly, Vienna, 7-12 April 2019.
- J. Münchmeyer, D. Bindi, U. Leser, and F. Tilmann. Convolutional event embeddings for fast probabilistic earthquake assessment. (Poster presentation), AGU Fall Meeting, San Francisco, USA, 9-13 December 2019.
- J. Münchmeyer, D. Bindi, U. Leser, and F. Tilmann. End-to-end PGA estimation for earthquake early warning using transformer networks. (Oral presentation), EGU General Assembly, Online, 4-8 May 2020.
- J. Münchmeyer, D. Bindi, U. Leser, and F. Tilmann. The Transformer Earthquake Alerting Model: Improving Earthquake Early Warning with Deep Learning. (Oral presentation), AGU Fall Meeting, Online, 13-17 December 2020.
- J. Münchmeyer, D. Bindi, U. Leser, and F. Tilmann. Insights into deep learning for earthquake magnitude and location estimation. (PICO presentation), EGU General Assembly, Online, 19-30 April 2021. https://doi.org/10.5194/egusphere-egu21-4718
- J. Münchmeyer, D. Bindi, U. Leser, and F. Tilmann. The Transformer Earthquake Alerting Model: A Data Driven Approach to Early Warning. (Oral presentation), Seismological Society of America (SSA) Annual Meeting, Online, 19-23 April 2021.
- J. Münchmeyer, J. Woollam, ..., D. Lange, A. Rietbrock, and F. Tilmann. SeisBench: A framework for machine learning in seismology. (Oral presentation), 37th General Assembly of the European Seismological Commission, Online, 19-24 September 2021.
- J. Münchmeyer, U. Leser, and F. Tilmann. A probabilistic view of earthquake rupture predictability. (Oral presentation), AGU Fall Meeting, Online & New Orleans, USA, 13-17 December 2021.
- J. Münchmeyer, J. Woollam, F. Tilmann, A. Rietbrock, D. Lange, ..., and H. Soto. Which picker fits my data? A quantitative evaluation of deep learning based seismic pickers. EGU General Assembly 2022, Vienna, Austria, 23–27 May 2022. https://meetingorganizer.copernicus.org/EGU22/EGU22-4071.html
- J. Münchmeyer, J. Woollam, F. Tilmann, A. Rietbrock, D. Lange, ..., and H. Soto. (2022). SeisBench: A toolbox for machine learning in seismology. Helmholtz AI Conference, Dresden, Germany, 2-3 June 2022.
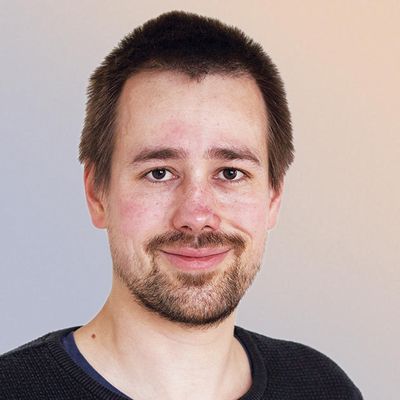
Peter Tillmann
Alumnus HEIBRiDS
Kontakt
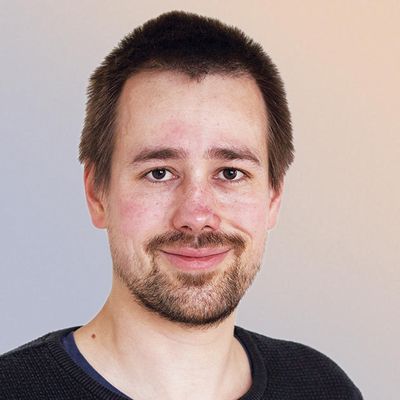
Supervisors:
Currently, perovskite-silicon (pero-Si) tandem solar cells are the most investigated concept to overcome the theoretical limit for the power conversion efficiency of single-junction silicon solar cells, with is 29.4%. Optical simulations are extremely valuable to study the distribution of light within the solar cells, and allow to minimize losses from reflection and parasitic absorption. For monolithic perovskite-silicon solar cells, it is vital that the available light is equally distributed between the two subcells, which is known as current matching. Nanotextures have proven to strongly reduce reflective losses. In this project we investigate, how realistic weather conditions affect the performance of pero-Si modules. We study, how different light management approaches, such as pyramidal texturing or (sinusoidal) nanotexturing influence the sensitivity of the solar module to the illumination condition. In contrast to single-junction silicon solar cells, (two-terminal) tandem solar cells are more sensitive to the spectral distribution of the incident light.
Full-length publications
- P. Tillmann, K. Jäger, and C. Becker (2020). Minimising the levelised cost of electricity for bifacial solar panel arrays using Bayesian optimization. Sustainable Energy Fuels, 4, 254-264. https://doi.org/10.1039/C9SE00750D
- K. Jäger, P. Tillmann, E.A. Katz, and C. Becker (2020). Perovskite/silicon tandem solar cells: Effect of luminescent coupling and bifaciality. Sol. RRL. https://doi.org/10.1002/solr.202000628
- K. Jäger, P. Tillmann, and C. Becker (2020). Detailed illumination model for bifacial solar cells. Opt. Express, 28, 4, 4751-4762. https://doi.org/10.1364/OE.383570
- P. Tillmann, B. Bläsi, S. Burger, M. Hammerschmidt, O. Höhn, C. Becker, and K. Jäger (2021). Optimizing metal grating back reflectors for III-V-on-silicon multijunction solar cells. Opt. Express, 29, 22517. https//doi.org/10.1364/OE.426761
- P. Tillmann, K. Jäger, A. Karsenti, L. Kreinin, and C. Becker (2022). Model-Chain Validation for Estimating the Energy Yield of Bifacial Perovskite/Silicon Tandem Solar Cells. Sol. RRL, 202200079. https://doi.org/10.1002/solr.202200079
Conference presentations
- P. Tillmann, C. Becker, and K. Jäger. Analysing the angular reflection losses of bifacial solar cells. (Poster presentation), European Photovoltaic Solar Energy Conference and Exhibition (EU PVSEC), Online, 7-11 September 2020.
- P. Tillmann, K. Jäger, E.A. Katz, and C. Becker. Relaxed current-matching constraints in perovskite/silicon tandem solar cell by bifacial operation and luminescent coupling. (Oral presentation), IEEE Photovoltaic Specialists Conference (PVSC), Online, 20-25 June 2021.
- P. Tillmann, K. Jäger, A. Karsenti, L. Kreinin, and C. Becker. Validation of Energy Yield Model for Bifacial Solar Cells and Prediction of Perovskite/silicon Tandem Solar Cell Performance. (Poster presentation), TandemPV, Freiburg, Germany, 30 May - 1 June 2022.
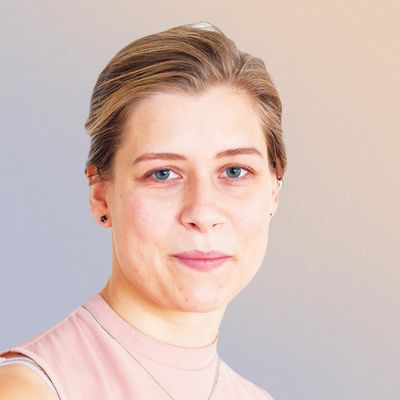
Anna Vlot
Alumnus HEIBRiDS
Kontakt
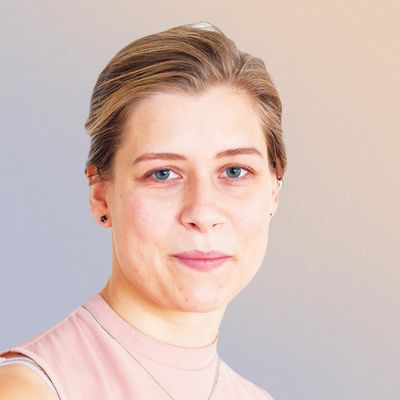
Supervisors:
Setareh Maghsudi (Uni Tübingen)
Cells are the building blocks of all multicellular organisms. Generally speaking, the DNA in each cell in a single organism is identical. Yet each different type of cell has its specialized function. These functional differences occur because cells of a particular identity transcribe a distinct set of genes into RNA molecules, many of which the cell then translates into proteins that determine cell structure, function, and identity. We do not yet fully understand the mechanisms that determine which genes and proteins a given cell produces. What we do know, however, is that the packing of DNA into a structure called chromatin plays a role. It is this packing that permits a 2-meter-long strand of DNA to fit into a cell nucleus with a diameter of no more than roughly 6 micrometres. If a gene lies in a region of the DNA that is tightly packed, the gene is not accessible for binding by the molecules that govern its transcription into RNA molecules. Thus, genes in inaccessible chromatin regions are not transcribed into RNA. However, protein-encoding regions make up just 2% of the human genome, and the accessibility of genomic regions alone does not explain cell-to-cell differences. Namely, non-protein-coding regions of the DNA, e.g. cis-regulatory regions, regulate gene expression. These regions, too, cannot exert their function if they are not accessible. Ultimately, the abundance of particular RNAs and the accessibility of chromatin together provide a starting point for unravelling the processes underlying cell identity acquisition and cell function.
Recently, researchers have begun measuring RNA abundance, chormatin accessibility, and more, in individual cells using so called single-cell omics assys. Analysis of the data obtained from these single-cell omics assays may provide novel insights into how cells aquire their identity. However, analysis of this data is complicated by its high-dimensional, sparse, and noisy nature. High dimensionality refers to the fact that tens of thousands of genes or hundreds of thousands of DNA region are measured in thousands to millions of cells. Sparsity occurs because most genes are not expressed in any given cell, and most regions of chromatin are not accessible. Besides, due to technical limitations, not all genes that are expressed or chromatin regions that are accessible in a given cell are captured. The combination of inherent sparsity and futher technical limitations results in noisy data with a poor signal-to-noise ratio. Taken together, these data characteristics complicate the identifcation of biologically meaningful patterns from the data, especially for genes that expressed at very low levels, or in only a few cells. This is of particular concern when considering cells at different stages of development since differences between cells may be restricted to the expression of only a few genes or subtle changes in chromatin accessibility.
In this project, we aim to develop methods to identify RNA molecules and cis-regulatory regions that characterize cell types and regulate the acquisition of cell identity. For this, we will adapt existing analytical approaches for the analysis of data representing continuous differentiation processes, without discretizing cells indetities into distinct cell states. This criterion is essential if we hope to identify genes and cis-regulatory regions that govern the development of cells in health and disease, where disease occurs due to abberent cell functions induced by disregulation of gene expression.
Full-length publications
- P. Rautenstrauch, A.H.C. Vlot, S. Saran, and U. Ohler (2021). Intricacies of single-cell multi-omics data integration. Trends in Genetics. https://doi.org/10.1016/j.tig.2021.08.012
- R. Shahan, C.W. Hsu, T.M. Nolan, B.J. Cole, I.W. Taylor, A.H.C. Vlot, P.N. Benfey, and U. Ohler (2022). A single cell Arabidopsis root atlas reveals developmental trajectories in wild type and cell identity mutants. Developmental Cell 57(4), 543-560.e9. https://doi.org/10.1016/j.devcel.2022.01.008
- A.H.C. Vlot, S. Maghsudi, and U. Ohler (2022). Cluster-independent marker feature identification from single-cell omics data using SEMITONES. Nucleic Acids Research, gkac639. https://doi.org/10.1093/nar/gkac639
Conference presentations
- R. Shahan, C.W. Hsu, T.M. Nolan, B.J. Cole, I.W. Taylor, A.H.C. Vlot, P.N. Benfey, and U. Ohler (2020). A single cell Arabidopsis root atlas reveals developmental trajectories in wild type and cell identity mutants. bioRxiv 2020.06.29.178863. https://doi.org/10.1101/2020.06.29.178863
-
A.H.C. Vlot, S. Maghsudi, and U. Ohler. Identification of marker genes and cis-regulatory regions using Single-cEll Marker IdentificaTiON by Enrichment Scoring (SEMITONES). (Poster presentation), 13th annual RECOMB/ISCB Conference on Regulatory & Systems Genomics with DREAM Challenges, Online, 16-19 November 2020.
-
A.H.C. Vlot, S. Maghsudi, and U. Ohler. Single-cEll Marker IdentificaTiON by Enrichment Scoring. (Poster and oral presentation), ISMB/ECCB 2021, Online, 25-30 July 2021.
- A.H.C. Vlot, S. Maghsudi, and U. Ohler. Identification of cis-regulatory regions using Single-cEll Marker IdentificaTiON by Enrichment Scoring (SEMITONES). (Poster presentation), EMBO Workshop Enhanceropathies: Understanding enhancer function to understand human disease, 6-9 October 2021.
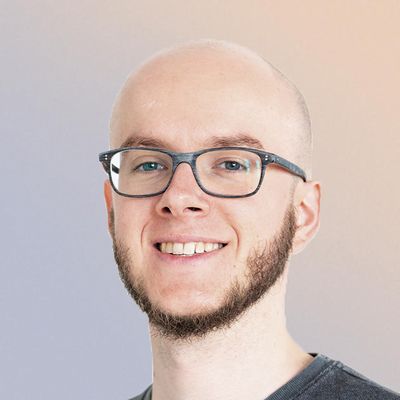
Leon Weber
Alumnus HEIBRiDS
Kontakt
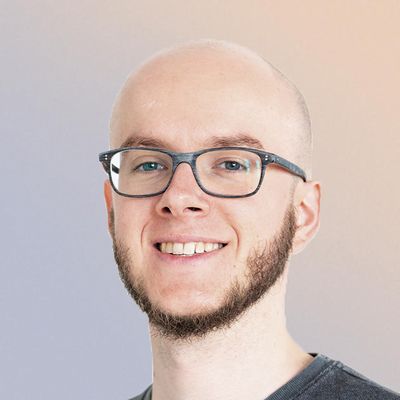
Supervisors:
Current attempts to decipher the molecular basis of cellular processes and human diseases are based on quantitative or qualitative models of the complex interplay between molecules in the cell, for instance in gene regulation, cellular signaling, or the metabolism. Obtaining such models in sufficient quality and breadth is a laborious task which today is predominantly based on human experts manually searching and reading the scientific literature with the aim to collect the many dispersed pieces of knowledge necessary to derive at a comprehensive picture. This work can be supported by using Text Mining, however, current research in this area focuses on extracting information from isolated sentences, which often produces unsatisfactory results as important contextual information is ignored (such as the experimental evidence of a reported fact, the precise species in which a finding was experimentally observed, the strength of the observed effects, possible previous treatments (with certain drugs) of the experimental system etc.). In this PhD project, we follow a radically different approach. We use the entire corpus of available scientific publications (roughly 30 Million abstracts, 1.5 Million full texts, possibly patents) as the source of inference for single relationships. To this end, a machine learning setup will be designed, where models of valid relationships are learned from all mentions of their constituents trained on a set of proven relationships. We use that approach to significantly expand the molecular network of several clinically relevant molecular pathways of which the PIs have comprehensive background knowledge, such as NF-kB signaling pathway, a pathway that is critically involved in cell faith decisions and perturbed in a number of diseases including cancer and inflammatory diseases, and the p53 pathway, which is strongly perturbed in cancer. The central aim of the PhD project is the extension of the currently available restricted pathway models, however, additional directions of expansion will also be investigated, such as development of cell-type -specific models, or elucidation of cross-talk to other pathways. We also envision using the new method to study connections between signaling pathways and existing targeted cancer therapies, for which patent texts would be extremely useful. Results from such text mining algorithms will be rigorously assessed in terms of their quality and relevance for biomedical research by (a) qualitatively checking the results at the literature level, and (b) quantitatively evaluating the performance of the expanded or improved pathways in typical analysis settings using OMICS data, such as pathways enrichment analysis and predictive power for selected phenotypes. The approach would allow a new way of predicting treatments that ideally can be adapted and specified for subgroups harboring individual combinations of perturbations in the disease-relevant pathways.
Full-length publications
- L. Weber, J. Münchmeyer, T. Rocktäschel, M. Habibi, and U. Leser (2019). HUNER: Improving biomedical NER with pretraining. Bioinformatics, 36(1), 295-302. 10.1093/bioinformatics/btz528
- L. Weber, P. Minervini, J. Münchmeyer, U. Leser, and T. Rocktäschel (2019). NLProlog: Reasoning with weak unification for question answering in Natural Language. In Proceedings of the 57th Annual Meeting of the Association for Computational Linguistics, 6151-6161. 10.18653/v1/P19-1618
- L. Weber, K. Thobe, O.A.M. Lozano, J. Wolf, and U. Leser (2020). PEDL: Extracting protein-protein associations using deep language models and distant supervision. Bioinformatics, 36(1), i490–i498. https://doi.org/10.1093/bioinformatics/btaa430
- W.D. Xing, L. Weber, and U. Leser (2020). Biomedical event extraction as multi-turn question answering. In Proceedings of the 11th Int. Workshop on Health Text Mining and Information Analysis, 88-96. 10.18653/v1/2020.louhi-1.10
- L. Weber, M. Sänger, J. Münchmeyer, M. Habibi, U. Leser, and A. Akbik (2021). HunFlair: An easy-to-use tool for state-of-the-art biomedical named entity recognition. Bioinformatics, btab042. https://doi.org/10.1093/bioinformatics/btab042
- L. Weber, M. Sänger, S. Garda, F. Barth, C. Alt, and U. Leser (2021). Humboldt @ DrugProt: Chemical-protein relation extraction with pretrained transformers and entity descriptions. In Proceedings of the 7th BioCreative Challenge Evaluation Workshop.
- L. Weber, S. Garda, J. Münchmeyer, and U. Leser (2021). Extend, don’t rebuild: Phrasing conditional graph modification as autoregressive sequence labelling. In Proceedings of the 2021 Conference on Empirical Methods in Natural Language Processing, 1213–1224.
- K. Singh, J. Münchmeyer, L. Weber, U. Leser, and A. Bande (2022). Graph Neural Networks for Learning Molecular Excitation Spectra.J. Chem. Theory Comp., 18, 7, 4408-4417. DOI: 10.1021/acs.jctc.2c00255
- J.A. Fries, N. Seelam, G. Altay, L. Weber, M. Kang, D. Datta, R. Su, S. Garda, B. Wang, S. Ott, M. Samwald, and W. Kusa (2022). Dataset Debt in Biomedical Language Modeling. In Proceedings of the Workshop on Challenges & Perspectives in Creating Large Language Models, 137-145. https://doi.org/10.18653/v1/2022.bigscience-1.10
- X. Wang, U. Leser, and L. Weber (2022). BEEDS: Large-Scale Biomedical Event Extraction using Distant Supervision and Question Answering. In Proceedings of BioNLP, 298-309. 10.18653/v1/2022.bionlp-1.28
- L. Weber, M. Sänger, S. Garda, F. Barth, C. Alt and U. Leser (2022). Chemical-Protein Relation Extraction with Ensembles of Carefully Tuned Pretrained Language Models. Database, 2022, baac098. https://doi.org/10.1093/database/baac098
- J.A. Fries, L. Weber, N. Seelam, G. Altay, et al. (2022). BigBIO: A Framework for Data-Centric Biomedical Natural Language Processing. https://arxiv.org/abs/2206.15076 [Preprint]
- H. Laurençon, L. Saulnier, T. Wang, C. Akik, A. V. del Moral, T. Le Scao, ... L. Weber, ... et al. (2022). The BigScience Corpus A 1.6 TB Composite Multilingual Dataset. https://openreview.net/forum?id=UoEw6KigkUn [Preprint]
- L. Weber, F. Barth, L. Lorenz, F. Konrath, K. Huska, J. Wolf, and U. Leser (2023). PEDL+: Protein-centered relation extraction from PubMed at your fingertip. Bioinformatics, 39, 11. doi:10.1093/bioinformatics/btad603
Conference presentations
- L. Weber, P. Minervini, J. Münchmeyer, U. Leser, and T. Rocktäschel. NLProlog: Reasoning with weak unification for question answering in Natural Language. (Poster presentation) 57th Annual Meeting of the Association for Computational Linguistics, Florence, Italy, 28 July - 2 August, 2019.
- M. Saenger, L. Weber, and U. Leser. WBI at MEDIQA 2021: Summarizing Consumer Health Questions with Generative Transformers. BioNLP Workshop - MEDIQA, 11 June 2021. https://www.aclweb.org/anthology/2021.bionlp-1.9.pdf
HDS-LEE
Mit Data Science die eigene Forschung vorantreiben, von führenden Data Scientists lernen und sich interdisziplinär austauschen – das ist möglich an der Helmholtz School for Data Science in Life, Earth and Energy (HDS-LEE) im nordrhein-westfälischen ABCD-Dreieck (Aachen-Bonn-Cologne-Düsseldorf).
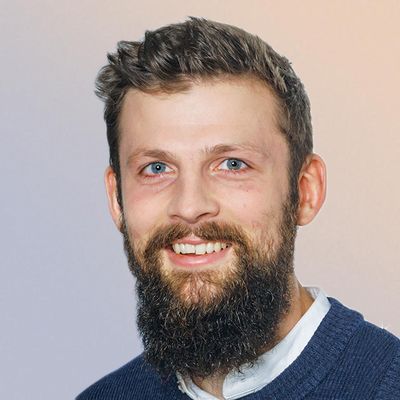
Eike Cramer
Alumnus HDS-LEE
Kontakt
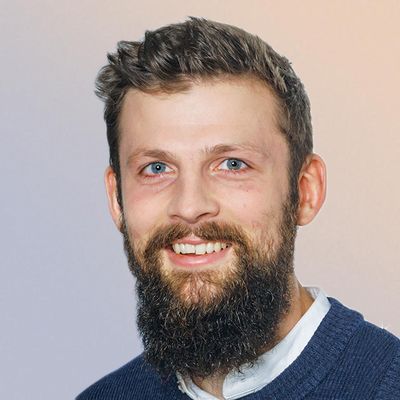
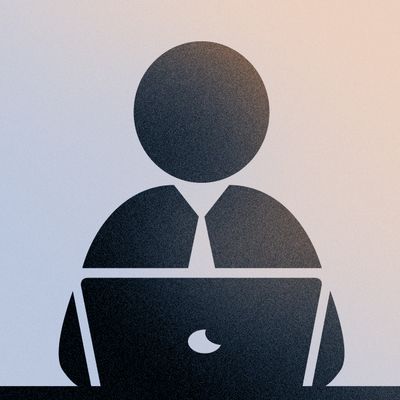
Viktor Grimm
Alumnus HDS-LEE
Kontakt
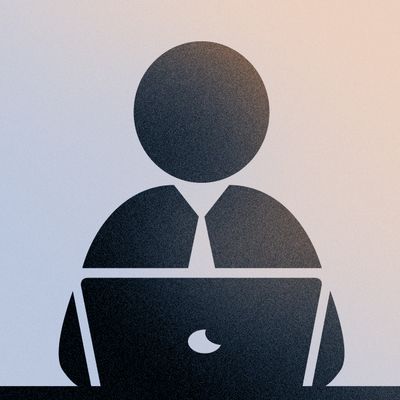
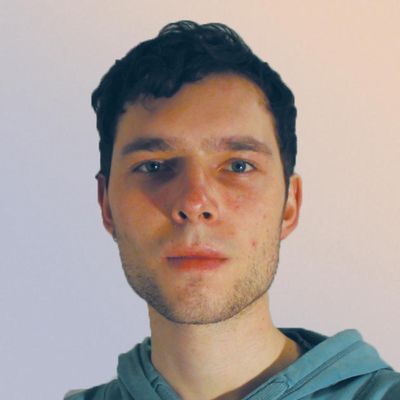
Johannes Kruse
Alumnus HDS-LEE
Kontakt
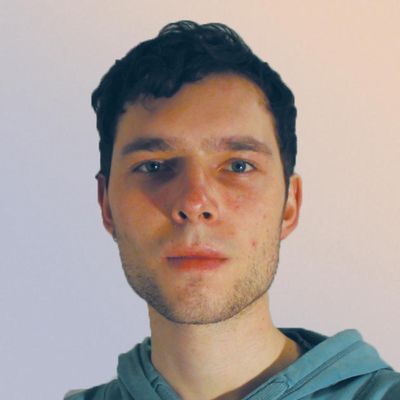
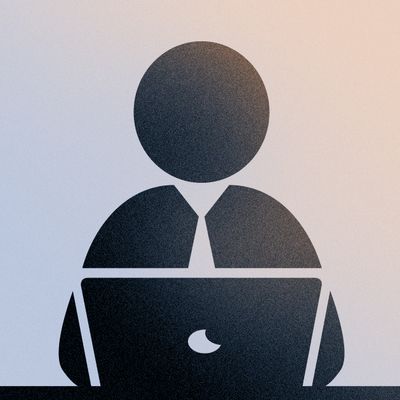
Leonardo Rydin Gorjão
Alumnus HDS-LEE
Kontakt
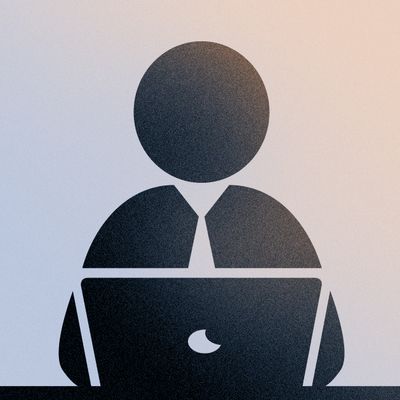
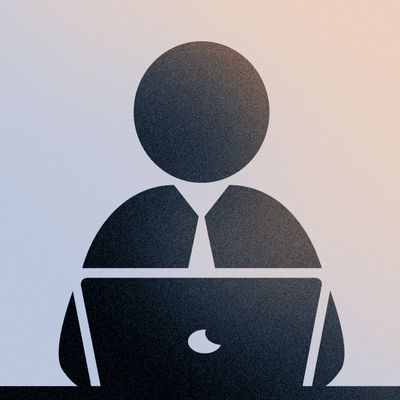
Giuliano Santarpia
Alumnus HDS-LEE
Kontakt
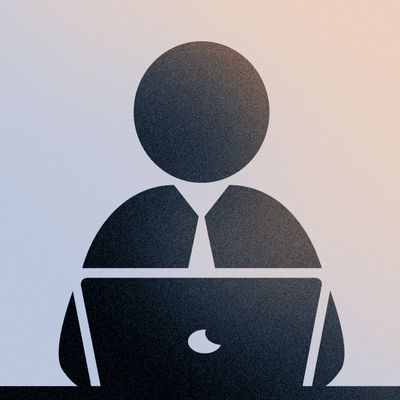
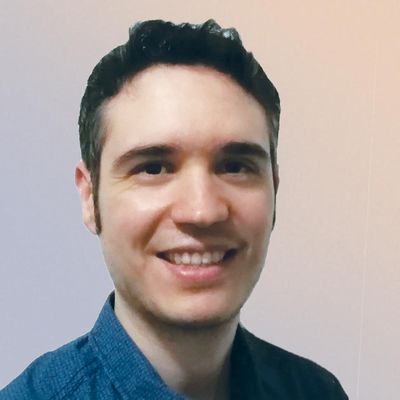
Alper Yegenoglu
Alumnus HDS-LEE
Kontakt
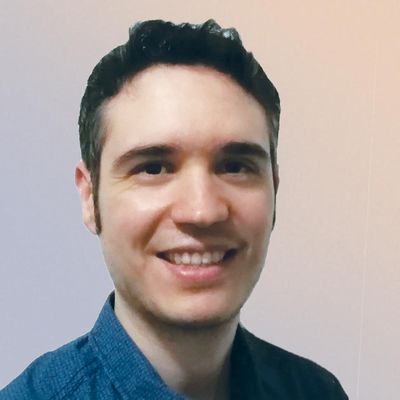
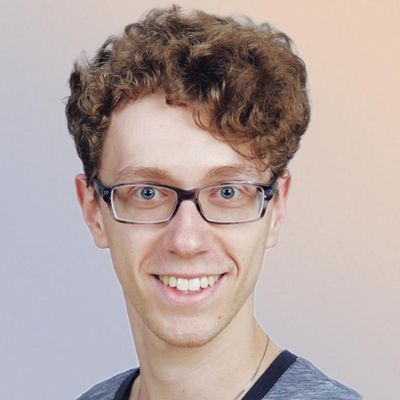
Daniel Wolff
Alumnus HDS-LEE
Kontakt
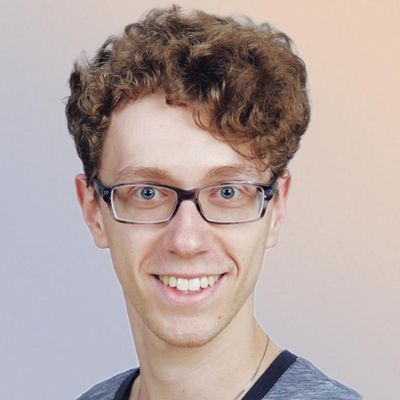
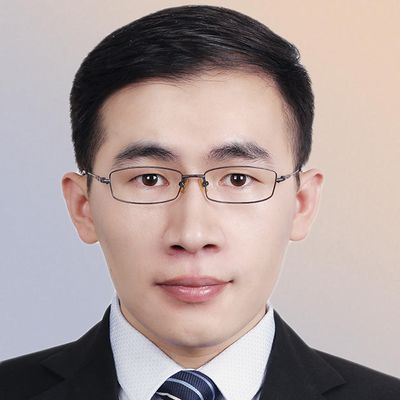
Hu Zhao
Alumnus HDS-LEE
Kontakt
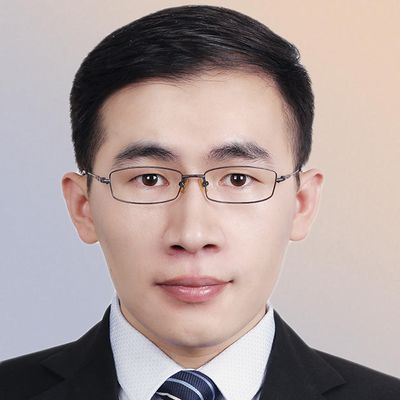
MarDATA
Mit Data Science in die Tiefe gehen, um den größten Lebensraum auf unserer Erde besser zu verstehen – und zu schützen. Die Helmholtz School for Marine Data Science (MarDATA) bündelt wissenschaftliche Meereskompetenz im hohen Norden.
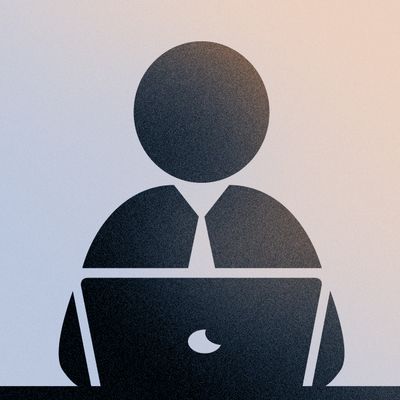
Justus Contzen
Alumnus MarDATA
Kontakt
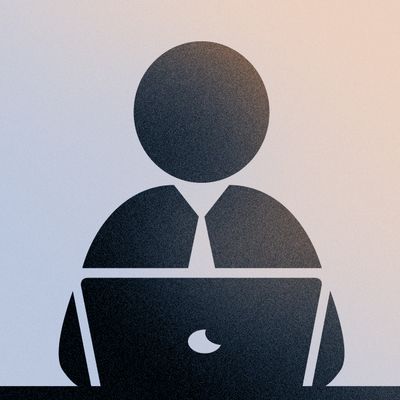
Supervisors:
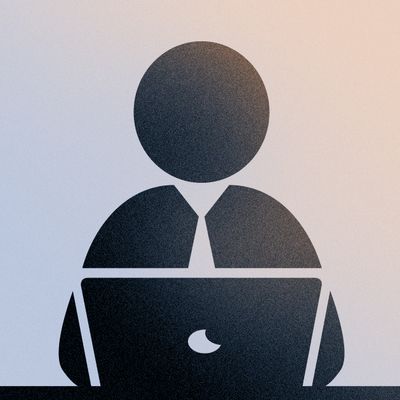
Marco Landt-Hayen
Alumnus Mar-Data
Kontakt
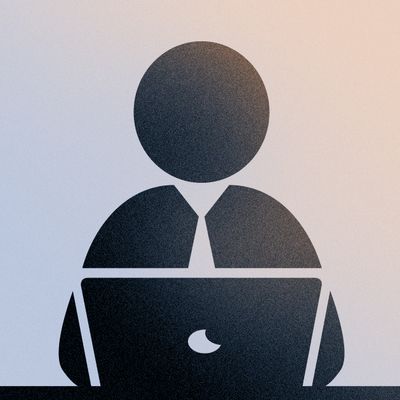
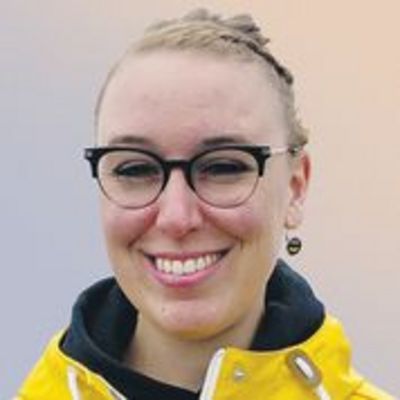
Maria-Theresia Pelz
Alumna MarDATA
Kontakt
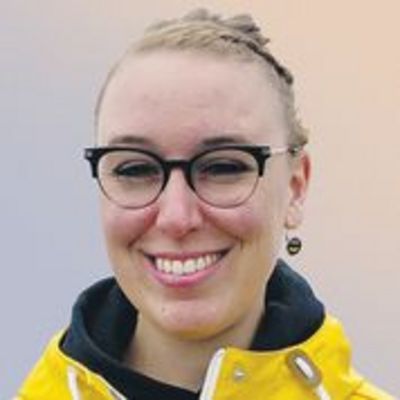
Supervisors:
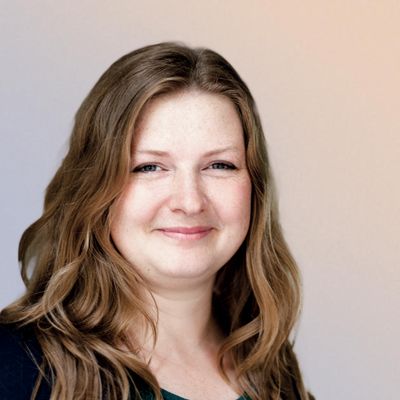
Carola Trahms
Alumna MarDATA
Kontakt
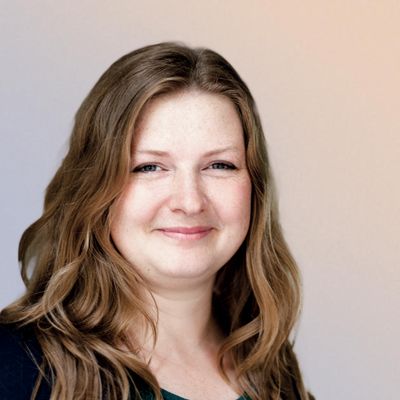
HIDSS4Health
Drei renommierte Institutionen sind an der Helmholtz Information & Data Science School for Health (HIDSS4Health) beteiligt: das Karlsruher Institut für Technologie (KIT), das Deutsche Krebsforschungszentrum (DKFZ) und die Universität Heidelberg. Hier bieten insgesamt mehr als 40 Forschungsgruppen mit einzigartigem Forschungsspektrum einen optimalen Ausgangspunkt, um zentrale Herausforderungen im Bereich Gesundheit zu lösen.
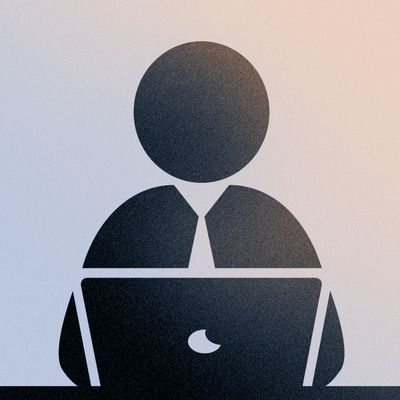
Vojtech Kumpost
Alumnius HIDSS4Health
Kontakt
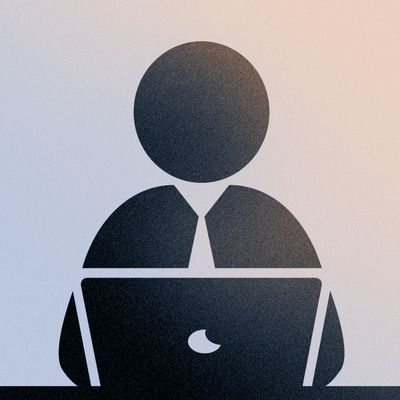
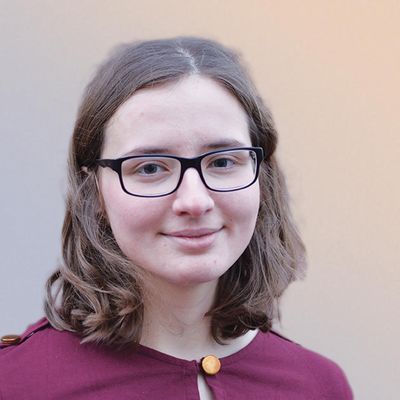
Katharina Löffler
Alumna HIDSS4Health
Kontakt
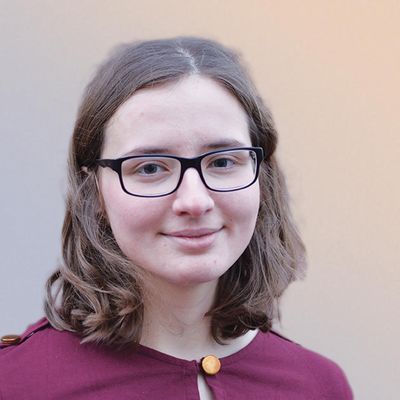
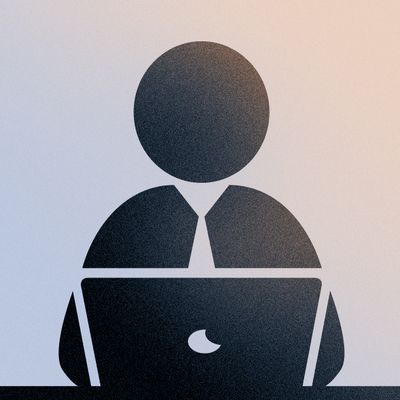
Constantin Seibold
Alumnus HIDSS4Health
Kontakt
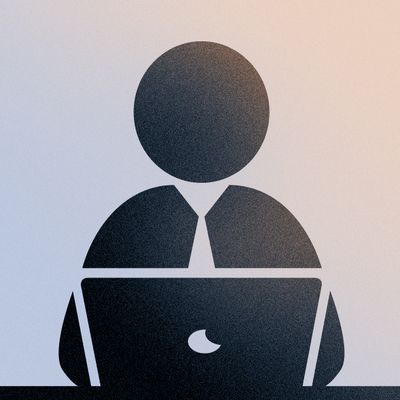
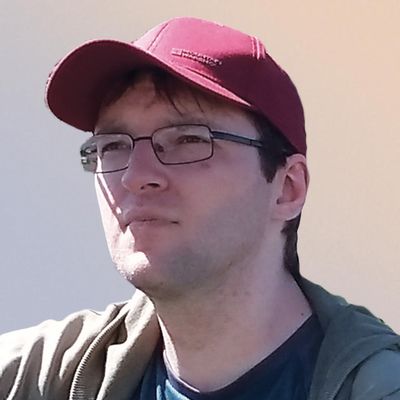
Rene Snajder
Alumnus HIDSS4Health
Kontakt
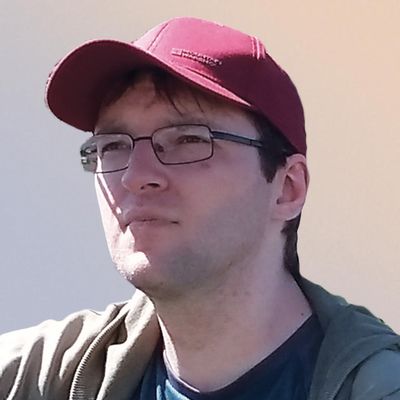
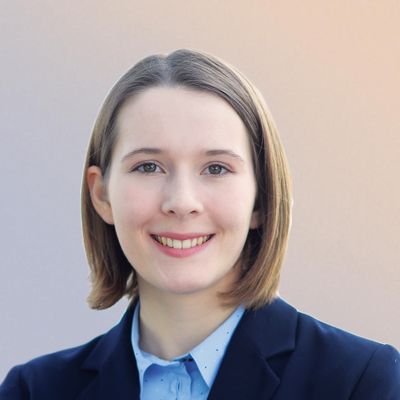
Pia Stammer
Alumna HIDSS4Health
Kontakt
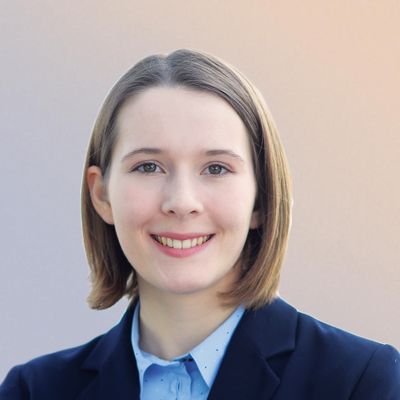