Lernen Sie Helmholtz-Wissenschaftlerinnen und -Wissenschaftler kennen, die einen Gastforscher oder eine Gastforscherin für einen kurzfristigen Forschungsaufenthalt in ihrer Gruppe willkommen heißen möchten. Hier erfahren Sie, an welchen Projekten die Forschungsgruppen derzeit arbeiten, wie Ihr Beitrag dazu aussehen kann und was Sie von den spezifischen Forschungsansätzen lernen können.
Helmholtz-weite Kooperationen möglich
Wenn Sie einen passenden Host Supervisor suchen, beachten Sie bitte: Sie können zu diesem Zweck auch andere Wissenschaftlerinnen und Wissenschaftler (in der Regel PIs oder Head of Divisions) an den 18 Helmholtz-Zentren kontaktieren und mit Ihnen eine Teilnahme am Helmholtz Visiting Researcher Grant oder am HIDA Trainee Network vereinbaren. Die hier aufgeführte Liste stellt lediglich eine Auswahl von möglichen Hosts dar.
Eine Übersicht der Helmholtz-Zentren und der jeweiligen Forschungsbereiche finden Sie hier:
Helmholtz-Betreuerinnen und -Betreuer
Lernen Sie hier einige potentielle Gastgeberinnen und Gastgeber an verschiedenen Helmholtz-Zentren kennen und erfahren sie mehr über deren jeweilige Data Science-Forschung durch einen Klick auf die Karten.
Bitte beachten Sie: Kontaktieren Sie Ihren potenziellen Betreuer oder Ihre potenzielle Betreuerin bitte vorab per E-Mail, um ein Forschungsprojekt vorzuschlagen und zu besprechen. Reichen Sie erst nach dieser Klärung Ihre Bewerbung ein.
Wenn Sie Fragen haben, senden Sie bitte eine E-Mail an: hida@helmholtz.de
Sie möchten selbst gerne Helmholtz-Gastgeber werden und suchen nach Unterstützung für Ihr Forschungsprojekt? Dann wenden Sie sich ebenfalls an die oben genannte E-Mail Adresse.
GFZ Helmholtz-Zentrum für Geoforschung
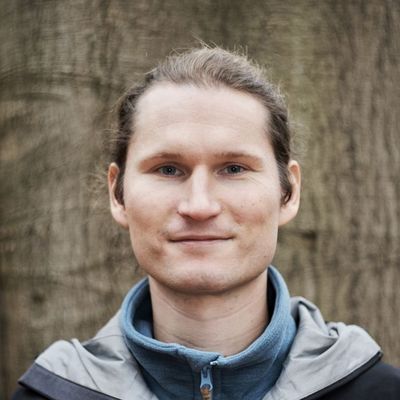
Benjamin Brede
Remote Sensing and Geoinformatics
Ansprechpartner
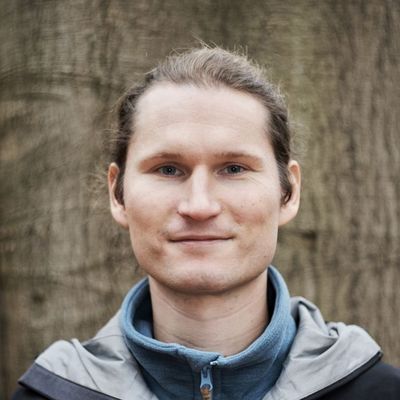
Three-sentence summary of your group's research: We link fine scale observations of vegetation to global satellite observations in order to calibrate and validate EO products. For this we use proximal remote sensing like Terrestrial and UAV laser scanning, radiative transfer models and machine learning.
What infrastructure, programs and tools are used in your group? UAV and terrestrial laser scanning, hyperspectral imaging.
What could a guest researcher learn in your group? How could he or she support you in your group? A researcher can learn to design field experiments that aim to link local to global observations of vegetation. A researcher can support the group with novel ideas on using AI and machine learning, in particular to apply on point cloud data.
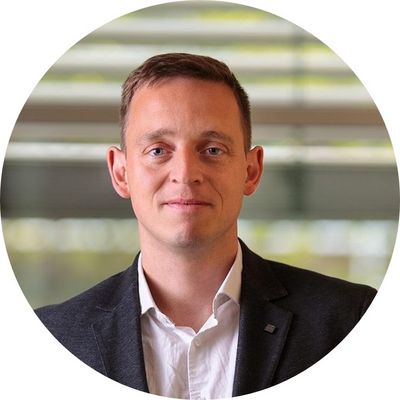
Sven Fuchs
Hydrothermal Energy
Ansprechpartner
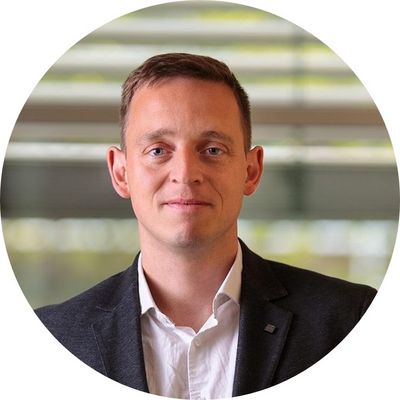
Short summary of your group's research: Our working group explores the Earth’s thermal field and geothermal resources, studies involved processes, quantifies their relevance, and provides knowledge on its behavior over time and across scales. The group is aimed at identifying priority targets for different geoenergy utilizations. With our work, we contribute to the transformation of the conventional energy system and to the reduction of CO2 emission.
With an applied focus, we elaborate exploration strategies to advance the successful development of geoenergy utilization in urban areas, where conventional surface exploration methods are often not applicable. We develop advanced methodologies for cross-scale characterization and for a better scale-dependent parameterization of the subsurface for risk reduction. A key concept is to combine geological expertise with a multi-methodological approach to establish adequate and reliable conceptual subsurface models. Fields of application include the sustainable provision of geothermal energy or the successful application of (thermal) storage systems.
With a more fundamental perspective, we investigate thermal processes in the crust and analyze the thermal field (including heat flow), and provide boundary conditions for multi-process integrated geodynamic models. With the integration of multidisciplinary observation data, we improve the understanding of the present thermal state and involved processes in subsurface thermal geosystems: from local to global views, from rock to lithospheric scales and across time domains.
What could a participant of the HIDA Trainee Network learn in your group? How could he or she support you in your group? We welcome all researchers with interest in geothermal field exploration, numerical methods and related data science. A guest researcher could learn about thermal field modeling approaches and their applications. Possible joint projects could involve processing and mapping of regional to global heat flow data, conducting analysis of observational data for mapping applications.
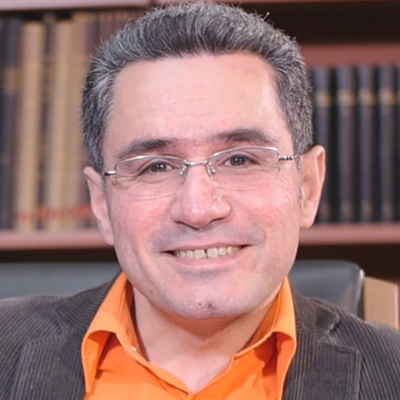
Mahdi Motagh
Radar Remote Sensing and Geohazards
Ansprechpartner
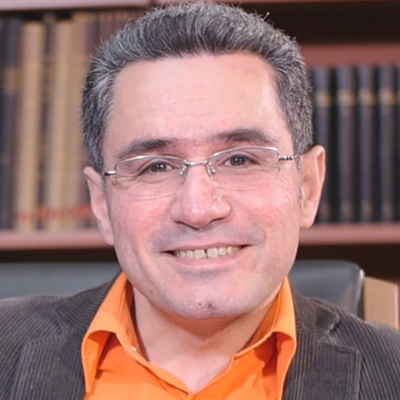
Three-sentence summary of your group's research: My research interests focus on the use of radar remote sensing data to investigate processes related to various types of geological phenomena and engineering applications, such as active tectonics, landslides, floods, groundwater extraction/injection, underground mining, glacier motion and ice mass change, dam stability, and anthropogenic activities in urban areas.
What could a guest researcher learn in your group? How could he or she support you in your group? Integration of SAR data with machine learning approaches to address natural hazards
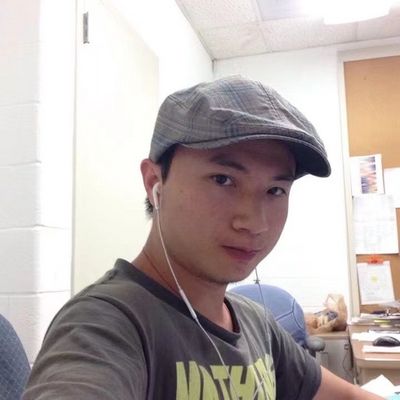
Hui Tang
Hazards and Surface Processes Research
Ansprechpartner
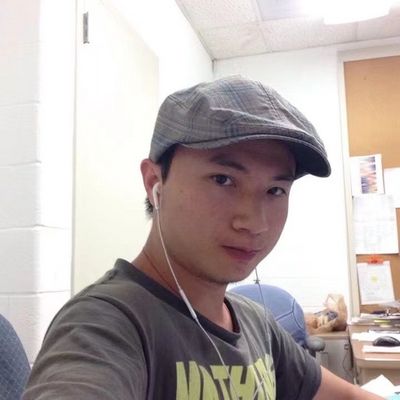
Short summary of your group's research: Our research group studies hazards and related surface processes across a wide range of environments, from mountain regions to coastal areas and even deep oceans, over different time scales. Our work has wide topics ranging from earthquake and tsunami, storm and hurricane, landslide and debris flow, to flood and paleo-flood. We use various tools and methods, including field surveys, remote sensing, environmental seismology methods, processes-based modelling, and data science methods, including machine learning to understand the physical processes behind all these natural hazards.
What infrastructure, programs and tools are used in your group? We use the High-Performance Computing infrastructure, including GPU and CPU clusters from our section, GFZ or Helmholtz association, as our main computation resource. For programming languages, we have used different mainstream languages, including Fortran, C, C++, Python, R, Matlab, and Julia, depending on the project. Regarding the data science model, we develop and use open-source platforms such as Scikit-learn, Tensorflow, and PyTorch.
What could a participant of the HIDA Trainee Network learn in your group? How could he or she support you in your group? As an interdisciplinary and diverse research group, we can offer many opportunities for HIDA Trainee Network participants with various interesting projects. There are two main types of projects: method-development-oriented projects and application-oriented projects. For a method-oriented, the participant could learn and develop physics-based machine learning methods for solving partial differential equations or investigating physics. An application-oriented participant could work on earth science or hazard-related research in the project using methods borrowing from computer vision, natural language processing, or signal processing. The participant will involve and support us in developing open-source data science model toolkits for the earth science and hazard science communities. Detailed project descriptions can be found on our group homepage. Feel free to contact us if you have any questions about the projects or bring your own ideas. We specifically welcome applicants from under-presented groups and third-world countries.
Forschungszentrum Jülich - FZJ
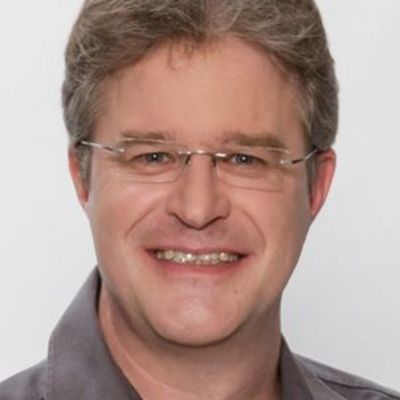
Maik Boltes
Civil Safety Research
Ansprechpartner
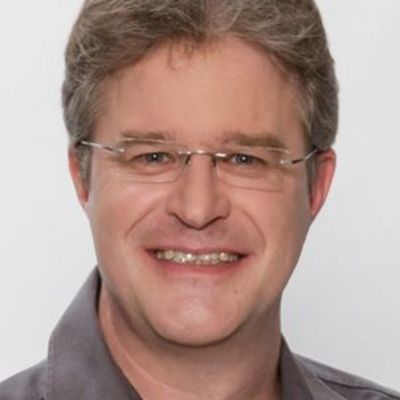
Short summary of your group's research: Our institute studies the dynamics of pedestrians to understand their behavior and thereupon develop reliable models for enhancing the safety of people inside crowds. Controlled experiments allow the quantitative description of the dynamics and enable the analysis of selected parameters under well-defined constant conditions. Data of these experiments is collected and analyzed by appropriately selected sensors and self-developed software.
What infrastructure, programs and tools are used in your group? During our experiments we are collecting a huge amount of data from (depth) cameras, motion capturing systems, IMU sensors, pressure sensors, heart rate sensors and electrodermal activity sensors sometimes combined with questionnaire data.
Our software is written in C++ or Python like our open-source tool PeTrack, which automatically extracts accurate pedestrian trajectories from videos.
Gathered data of all our experiments can be found in our open data archive.
What could a participant of the HIDA Trainee Network learn in your group? How could he or she support you in your group? We are a multidisciplinary young group of physicists, mathematicians, engineers, sociologists, psychologists, and computer scientists.
Our institute is one of the leading institutions in the field of experimental pedestrian research.
The dynamics and behavior of people in a crowd have many influences. The diversity of performed experiments and the amount of experimental data in our group is large. We learned in the past that a new view, method or discipline can give us another or extended insight to our data so that we would be happy to welcome you to our group.
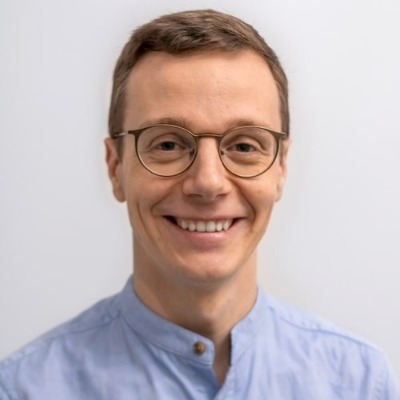
Gabriele Cavallaro
Simulation and Data Lab
Ansprechpartner
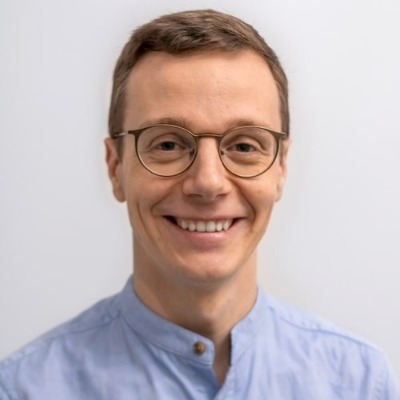
Short summary of your group's research: The joint research group ''High Productivity Data Processing (HPDP)'' at JSC and University of Iceland is highly active in developing parallel and scalable machine (deep) learning algorithms for remote sensing data processing and many other types of applications (i.e., medical research and retail sectors). The main backbone of the research group is the large number of PhD students that are jointly supervised with the University of Iceland. Furthermore, the HPDP works actively with the Cross-Sectional Team Deep Learning (CST DL) and the Helmholtz AI consultant team at JSC.
What infrastructure, programs and tools are used in your group? By being located at JSC, HPDP can rely on HPC technologies with MPI, OpenMP and CUDA (with TensorFlow, Keras, pyTorch, Chainer, Horovod) but also on innovative quantum computing systems.
What could a participant of the HIDA Trainee Network learn in your group? How could he or she support you in your group? Learn how data intensive computing approaches have become indispensable tools to deal with the challenges posed by applications from a diverse range of applications.
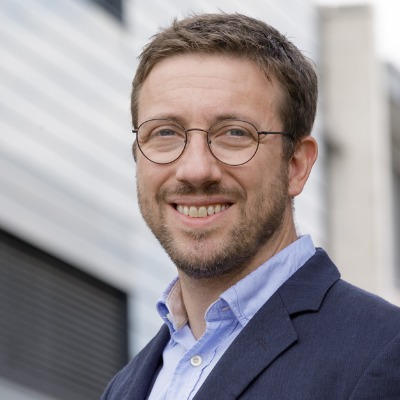
Timo Dickscheid
Structural and functional organisation of the brain
Ansprechpartner
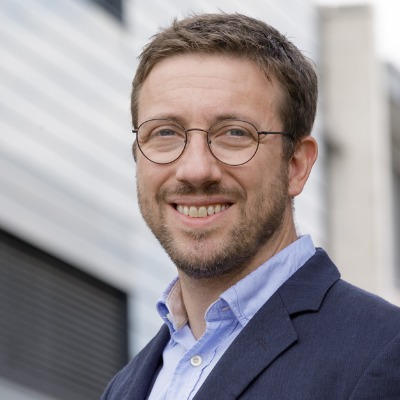
Short summary of your group's research: The Big Data Analytics group in Jülich develops software and methodology to build a microscopic resolution 3D model of the human brain, which includes detailed information about distributions of neuronal cells and nerve fibers, and microstructurally defined 3D maps of brain areas. This requires microscopic imaging and analysis of large quantities of histological brain sections at high throughput, leading to image datasets at the Petabyte scale.
At the intersection of neuroinformatics, computer vision and artificial intelligence (AI), our research addresses
- Data and workflow management for high throughput microscopic imaging,
- Machine learning and computer vision algorithms for biomedical image analysis on high performance computers,
- Software development for structured access to large image datasets and interactive 3D exploration of high-resolution brain atlases over the web.
What infrastructure, programs and tools are used in your group? The trainee will be part of the local Helmholtz AI unit in Jülich. Our data management and image analysis workflows run on a distributed environment which includes Jülich’s modular high-performance computing systems. Image acquisition of whole-brain sections at micrometer resolution is performed on a high throughput microscopy facility. Outputs of our research are typically integrated with the human brain atlas hosted in the European research infrastructure EBRAINS, to which we contribute key developments. Most of our software is implemented in Python. Image analysis and machine learning approaches use common Deep Learning frameworks, typically PyTorch or Tensorflow.
What could a participant of the HIDA Trainee Network learn in your group? How could he or she support you in your group?
A trainee in our group can learn about
- biomedical computer vision, especially processing and analysis of high resolution image data using state of the art AI methods
- distributed workflow management and parallel processing of big image data on supercomputers
- neuroinformatics.
A trainee can support our group with practical experience in machine learning approaches for image analysis from the point of view of another application domain. A trainee could also contribute with experience in inverse modelling, simulation, or computer graphics.
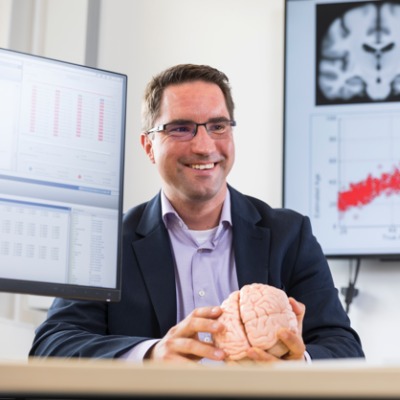
Simon Eickhoff
Brain and Behaviour
Ansprechpartner
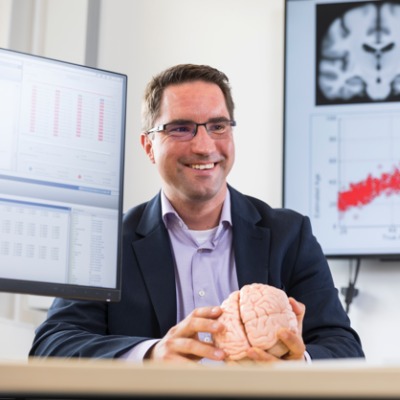
Short summary of your group's research: We develop and apply novel methods to better understand the organization of the human brain and to relate it to behavioral phenotypes in healthy and as biomarkers in clinical populations. To do so, we capitalize on machine learning approaches. In addition to these scientific objectives, we also develop open access methods and tools for data management and reproducible research.
What infrastructure, programs and tools are used in your group? Python, R, MATLAB, HPC, machine learning, neuroimaging
What could a participant of the HIDA Trainee Network learn in your group? How could he or she support you in your group? A HIDA trainee can choose from diverse topics from machine learning, data management to biomarker discovery. For technically oriented trainees we offer opportunities to contribute to our growing set of tools. For application-oriented trainees we offer participation in ongoing projects to uncover brain-behavior relationships.
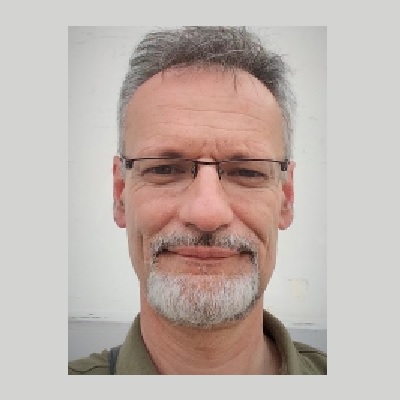
Fabio Fiorani
Plant Sciences
Ansprechpartner
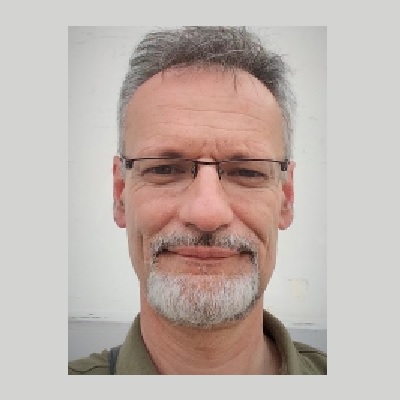
Short summary of your group's research: We focus on the application and development of high-throughput, non-invasive phenotyping methods for screening of plant shoot and root productivity traits. In our group teams of plant biologists, engineers and image processing specialists cooperate to realize screening methodologies for shoot and root growth and architecture combined with physiological measurements of plant performance and plasticity to environmental challenges. Interpretation of results requires the evaluation of multi-dimensional data structures which include dynamic changes of plant phenotypes over time, environmental and imaging sensors data.
What infrastructure, programs and tools are used in your group? We have developed and operate a unique set of automated experimental platforms to analyze plant phenotypes in a minimally-invasive fashion, please click here to learn more.
What could a participant of the HIDA Trainee Network learn in your group? How could he or she support you in your group? Participants to the HIDA Trainee Network would benefit from learning: a) methodologies for high-throughput screening in plants; b) data analyses and visualization of complex data sets; c) experimental designs for plant phenotyping. HIDA Trainee Network participants would support our continued efforts in improving and automating data analyses using existing data stored in several institutional databases.
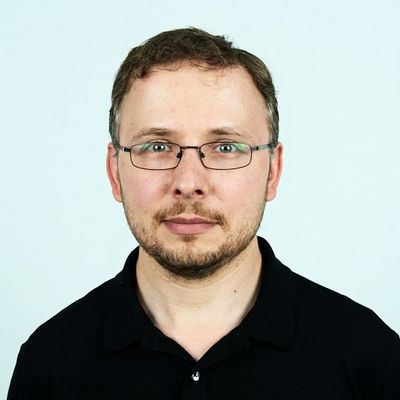
Johannes Laube
Stratosphere (ICE-4)
Ansprechpartner
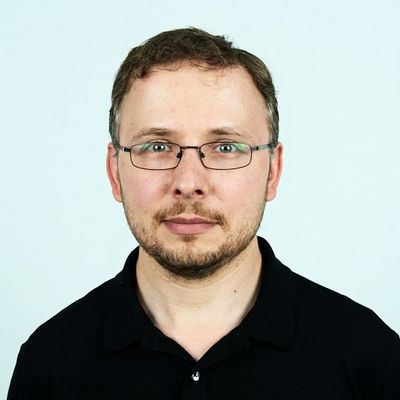
Short summary of your group's research: Our main focus is on flying sensors on balloons to stratospheric altitudes up to 35 km. For instance, we collect air samples and then subsequently analyse these for their content of trace gases important for stratospheric ozone (such as CFCs or halons) or climate (e.g., CO2 or HFCs). We can use the data to better understand the state of the ozone layer or circulation changes at altitudes well above the reach of aircraft.
What infrastructure, programs and tools are used in your group? Observation platforms are aircraft and balloons, measurements are mostly based on cavity-ringdown spectroscopy and mass spectrometry. Current project examples are the ERC Starting Grant EXC3ITE and we are also involved in Modular Observation Solutions for Earth Systems MOSES. We closely collaborate with the atmospheric modelling community, e.g. the Chemical Lagrangian Model of the Stratosphere CLaMS.
What could a participant of the HIDA Trainee Network learn in your group? How could he or she support you in your group? The learning experience would include the challenges of observations in as hostile an environment as the stratosphere, measurement and data retrieval techniques, and inferring key parameters such as average stratospheric transit times from the various trace gas data. Especially the latter part is where a guest researcher might be able to help us to improve our algorithms and procedures.
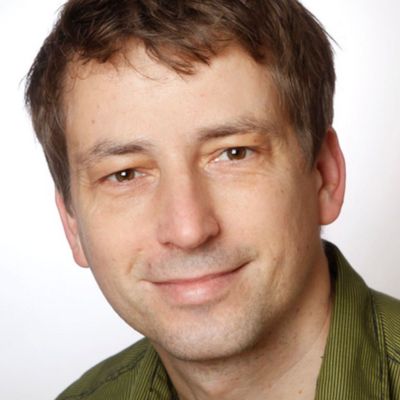
Christoph Lerche
Physics Group, Medical Imaging Physics
Ansprechpartner
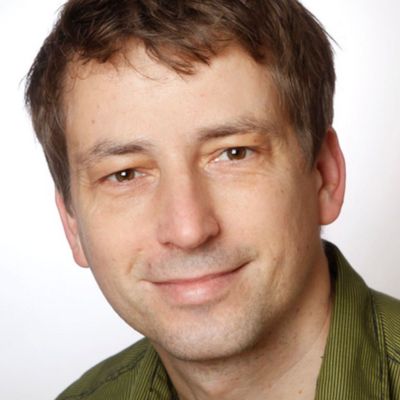
Short summary of your group's research: The PET physics group focusses on
- PET methodology development (image reconstruction and data corrections as scatter correction and attenuation correction)
- Multimodal Imaging (Applications and Methodology for combined PET/MR and PET/MR/EEG acquisitions) System development of an UHF MR compatible BrainPET insert
- Quantitative Methods for PET and PET/MR imaging
What infrastructure, programs and tools are used in your group?
- 3T MR-PET insert, consisting of a high resolution BrainPET, (a prototype by Siemens), and a commercial 3T MAGNETOM Trio MRI scanner with several phantoms
- Access to the Radiochemistry department for generation of Radio-tracer and Radionuclides
- Access to High Performance Computing Installations
- Access to PET and MR patient and volunteer data from other studies (neuroreceptor/neurotransmitter studies, neuroonkology)
- Monte Carlo Simulation tools Geant4/Gate, analysis tools: PMOD, SPM
What could a participant of the HIDA Trainee Network learn in your group? How could he or she support you in your group? Our group offers the following expertises
- Thorough understanding of PET systems and Image-formation from gamma photon detection to image reconstruction including all relevant corrections (scatter, attenuation, randoms, dead time)
- Unique access to a dedicated 3T MR BrainPET insert and a 7T human MRT in a research setting with all technical an league requirements to run studies involving human volunteers
- Starting in 2023: access to a in-house developed 7T MR BrainPET insert for humans
- We would highly benefit from guest researcher with expertises in Image post processing (PET and MR), Data science for information extraction from multi parametric and multimodal images, Monte Carlo Simulations
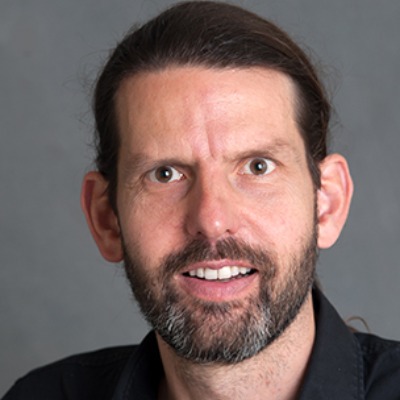
Eric von Lieres
Modeling and Simulation
Ansprechpartner
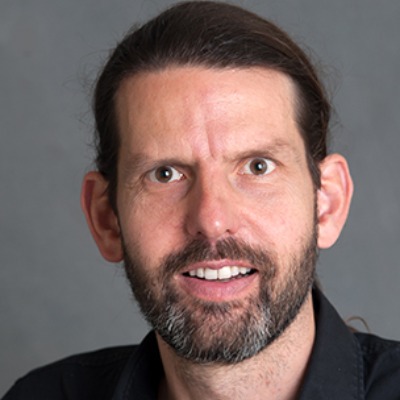
Short summary of your group's research: We provide our local colleagues and the scientific community with state-of-the-art models, algorithms and software. Current challenges in strain selection and optimization as well as process analysis and development are addressed in a strongly data-driven fashion in close collaboration between theory, simulation and experiment. Typical projects involve data analysis, model calibration or training, uncertainty analysis, experimental design. Model predictions are applied for testing hypotheses and focusing experimental work.
What could a participant of the HIDA Trainee Network learn in your group? How could he or she support you in your group? Many projects require advanced numerical methods and high-performance computing, in particular when machine learning/ artificial intelligence, Monte Carlo simulations, global optimization or CFD simulations are applied. We develop and maintain dedicated software packages, most of which are published as open source code https://github.com/modsim.
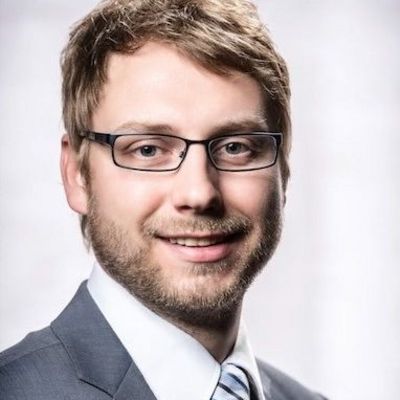
Andreas Lintermann
Fluids & Solids Engineering
Ansprechpartner
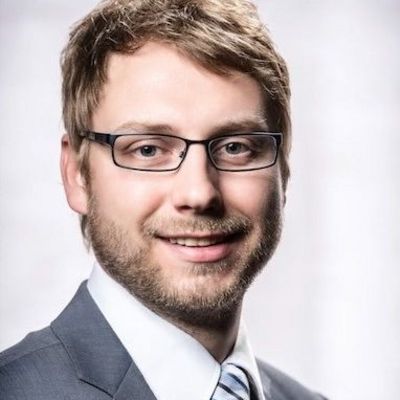
Short summary of your group's research: The SDL FSE's research focuses, amongst others, on lattice-Boltzmann methods, artificial intelligence, high-performance computing, heterogeneous computing on modular supercomputing architectures, high-scaling meshing methods, efficient multi-physics coupling strategies, and bio-fluidmechanical analyses of respiratory diseases. Furthermore, the SDL FSE aims at supporting users from engineering sciences who have already developed parallel codes but need support for the use of massively parallel systems regarding high scalability, memory optimization, programming of hierarchic computer architectures, and performance optimization on compute nodes.
What infrastructure, programs and tools are used in your group? The group mainly uses the high-performance computing (HPC) systems available at JSC for its various simulation applications. As a simulation code, a massively parallel multi-physics framework, jointly developed with the Institute of Aerodynamics and Chair of Fluid Mechanics, RWTH Aachen University, is employed. The big data outputs generated by the simulation tools are post-processed, e.g., by in-house developed tools, ParaView, machine-learning algorithms, or by Jupyter-based scripts.
What could a participant of the HIDA Trainee Network learn in your group? How could he or she support you in your group? The doctoral researcher will have access to the latest supercomputing hardware installed at JSC and will learn from the experts in the group to develop, adapt, and optimize simulation codes. He or she will support the member of the SDL FSE in running large-scale simulations on the production HPC machines at JSC and post-process the data to gain new insights to physical phenomena in the realm of fluid mechanics. Furthermore, the doctoral researcher will be able to learn how research in a European Center of Excellence in Exascale Computing is performed and has the opportunity to contribute to the corresponding cutting-edge research in bringing AI technologies along various use-cases to exascale.
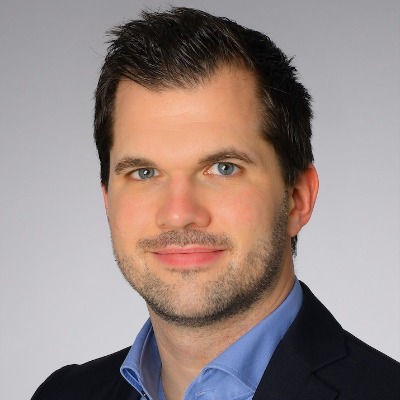
Philipp Lohmann
Physics of Medical Imaging
Ansprechpartner
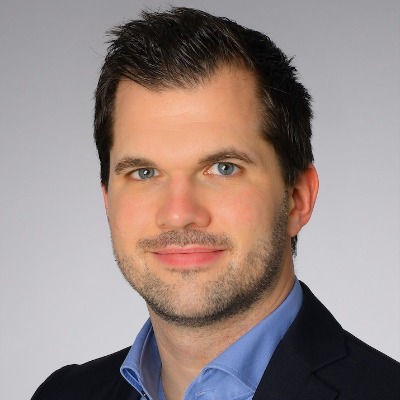
Short summary of your group's research: The research of the Brain Tumor Group within the Institute of Neuroscience and Medicine focuses on multimodal imaging in patients with brain tumors, with particular emphasis on amino acid PET in combination with advanced high-field and ultra-high-field MRI. In addition to the development and evaluation of novel PET tracers and PET/MRI methods, the potential of advanced image analysis such as radiomics and deep learning for patients with brain tumors is explored. A major goal of our research is the correlation of imaging findings and radiomics with local neuropathology and histomolecular markers, for which the close collaboration with the surrounding university hospitals in Aachen, Bonn, Cologne, Düsseldorf, and others, is extremely valuable and furthermore offers a high potential for verification and translation of research results into the clinic.
What infrastructure, programs and tools are used in your group? Amino acid PET, advanced MRI, ultra-high-field MRI, hybrid MR/PET, Python, Pyradiomics, PyTorch, LifeX, PMOD, FSL, HDBET, HDGLIO, ITK-SNAP, OsiriX, Matlab, High performance computing, etc.
What could a participant of the HIDA Trainee Network learn in your group? How could he or she support you in your group?
- Amino acid PET in combination with advanced, ultra-high-field MRI in patients with brain tumors
- Advanced image analysis including static and dynamic amino acid PET and MRI
- Feature-based as well as deep learning-based radiomics based on PET and MRI in patients with brain tumors
- Correlation of PET/MRI imaging findings and radiomics with local neuropathology and histomolecular markers
- Establishment of an imaging database of amino acid PET imaging in patients with brain tumors for advanced image analysis
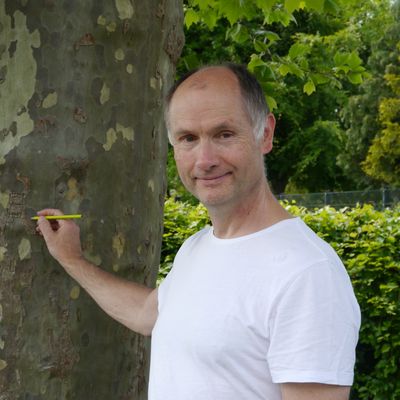
Martin Schultz
Earth System Data Exploration
Ansprechpartner
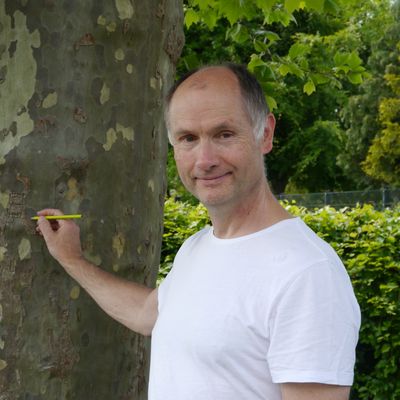
Short summary of your group's research: ESDE explores a wide range of machine learning methods for the analysis and forecasting of weather, climate and air quality data. We focus primarily on large, computationally intensive problems and adopt high-end deep learning approaches. Next to machine learning research we are also strongly interested in developing FAIR big data workflows. The 20+ group members have diverse educational backgrounds and build a lively international team of highly motivated researchers and software developers.
What infrastructure, programs and tools are used in your group? We make good use of JSC's high-end computing systems (https://go.fzj.de/juwels) and also operate on smaller GPU clusters. Tensorflow and Pytorch are our main (Python) software libraries. We also use containers and tools like Horovod to port ML codes to the supercomputer.
What could a participant of the HIDA Trainee Network learn in your group? How could he or she support you in your group? If you already have a good background in advanced machine learning techniques (i.e. beyond MLP) you may take it to the next level with a visit to our group and we will be happy to teach you the necessary basics of weather and air pollution science so that you can apply your skills to the problems we work on in our group. If you have a strong background in environmental science but only limited experience with machine learning (but you know how to program in Python) you may learn a lot about more sophisticated deep learning methods and we might be able to integrate your specific experience to better address our environmental research questions.
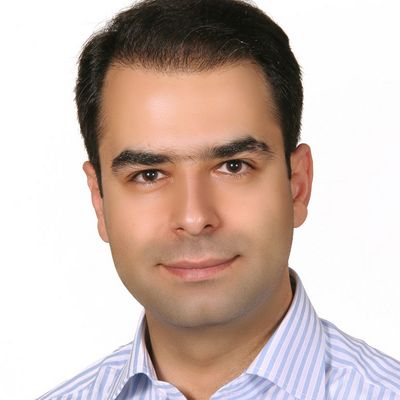
Masoud Tahmasian
Brain and Behaviour
Ansprechpartner
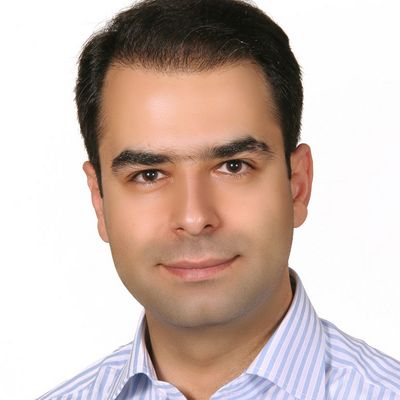
Short summary of your group's research: We use a multi-level approach utilizing computational tools and big data to gain an overall insight into neurological and psychiatric disorders to pave the way for personalized medicine. In particular, we focus on the interplay between sleep/sleep disorders and medical and mental conditions using phenotypic and multimodal neuroimaging data (e.g., sMRI, fMRI, PET).
Masoud Tahmasian is part of Prof. Dr. Simon Eickhoff's group on System Medicine.
What infrastructure, programs and tools are used in your group? We use state-of-art methodologies such as machine-learning to big clinical and population-based databases using high-performance computing tools.
What could a participant of the HIDA Trainee Network learn in your group? How could he or she support you in your group? The guest researcher will apply several analyses including machine-learning to identify the role of sleep disturbances and brain structures on predicting neuropsychiatric conditions such as depression using a large-scale general-population sample (e.g., UKBB).
He/She should perform the analyses independently and help us to build a nice analytic framework to find the neurobiological mechanisms behind the link between sleep and neuropsychiatric disorders.
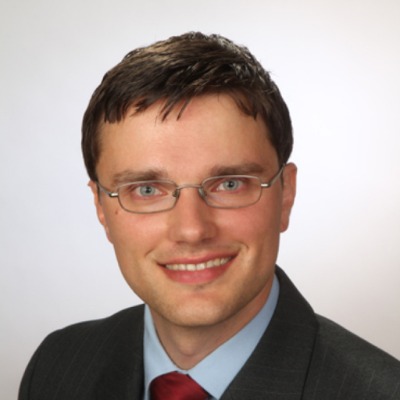
Dieter Weber
Microscopy and Spectroscopy with Electrons
Ansprechpartner
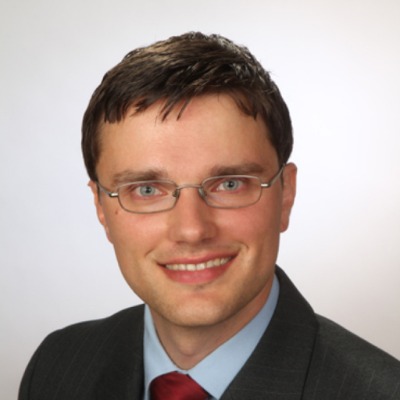
Short summary of your group's research: We are developing solutions for high-performance data processing, data management and automation in electron microscopy. This includes software like here as well as IT systems and infrastructure. Since modern detectors for electron microscopy can reach a data rate of 50 GB/s, datasets can contain terabytes of data, and electron microscopy is a visual and interactive method, these solutions can be characterized as interactive high performance computing.
What infrastructure, programs and tools are used in your group?
- MapReduce-like processing, "divide and conquer", linear algebra, inverse problem solving
- Python, NumPy, SciPy, Dask, Numba, CuPy, Torch, Jupyter, JupyterHub;
- Linux, Windows;
- Nextcloud, NAS, NFS, CIFS, SSH;
- GitHub, GitLab, Azure Pipelines, PyPI, Zenodo;
- Tox, Pytest, Sphinx;
- product management, project management, software development, test-driven development, Agile;
- PCIe 4.0, SSD RAIDs, 10 GBit Ethernet, AMD EPYC, GPGPU
What could a participant of the HIDA Trainee Network learn in your group? How could he or she support you in your group? You can learn high performance data processing with Python, in particular throughput-oriented processing of large-scale data. That can also involve finding suitable mathematical approaches and implementation strategies to transform an existing "proof of principle" reference implementation into a production-ready high-performance tool. Typically, we achieve speed-ups of 100x to 1000x from a "naive" NumPy or Matlab-based implementation.
Furthermore, you can learn software development methodologies, in particular the "GitHub flow", in combination with quality assurance through automated tests and continuous integration.
Contributions to scientific Open Source software are highly appreciated as a form of support. That can involve software which we actively maintain and develop, work on upstream dependencies, or all other forms of contributions to the Open Source software ecosystem. Such contributions can be in-kind by simply improving existing software and/or releasing new software under an Open Source license, or by joint applications for funding where parts of the resources are dedicated to software development and maintenance.
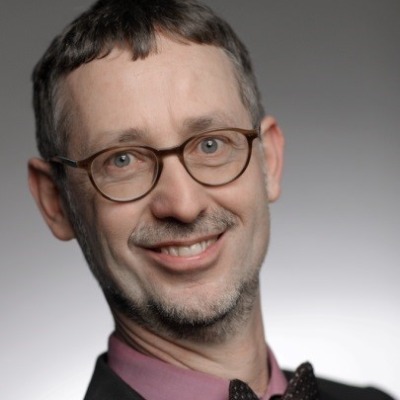
Wolfgang Wiechert
Biotechnology
Ansprechpartner
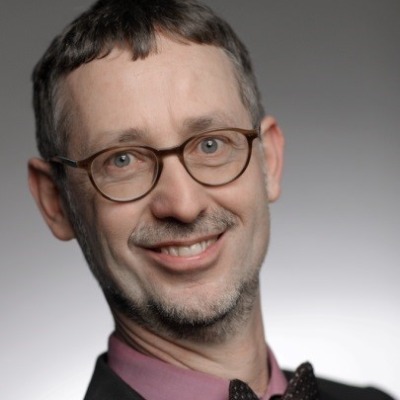
Short summary of your group's research: Modeling, Simulation and Data Analytics in the fields of systems metabolic engineering and bioprocess development: Cell and process modeling, Omics and bioprocess data processing, process parameter estimation, microbial image analysis, digitalization in lab automation
What infrastructure, programs and tools are used in your group? Hosting CADET and 13CFLUX2 software systems. Using and developing C++, python, Matlab toolboxes. High performance parameter estimation in complex systems, MCMC for parameter estimation and multi model analysis. Deep learning for image analysis. Supercomputing applications in process simulation.
What could a participant of the HIDA Trainee Network learn in your group? How could he or she support you in your group?
Wanted: Expertise in cutting edge Bayesian methods, image analysis and high performance computing
Offering: Challenging realistic problems from the above mentioned fields
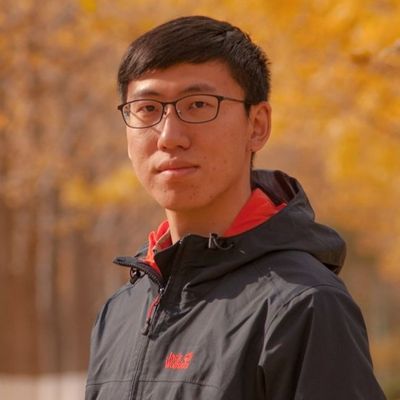
Yuankai Yang
Nuclear Waste Management
Ansprechpartner
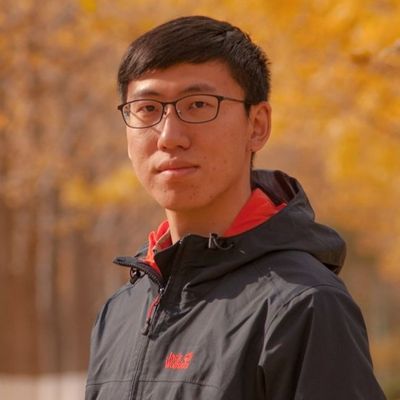
Short summary of your group's research: Analysing complex coupled thermo-hydraulic-mechanical-chemical-transport processes relevant to environmental systems on different scales.
My research at IEK-6 focuses on the development of complementary computational approaches to analyse and interpret the complex coupled THMC-processes in the near- and far-field of geological repositories for radioactive wastes on different scales, employing high-performance computing (HPC) resources provided by the Jülich Supercomputing Centre. This work aims at an enhanced process and system understanding across scales as well as at the reduction of uncertainties and conservatisms in performance assessments, contributing thus to the scientific basis for an in-depth comparison of different repository concepts and sites as required for the German site selection procedure.
What infrastructure, programs and tools are used in your group? We use the Lattice Boltzmann Method (LBM) for simulations. The High-Performance Computing (HPC) Cluster JURECA-DC is our main computational resource. Currently, we are using CUDA, Matlab, Python, Comsol, and Fortran for developing our numerical framework and data analysis.
What could a participant of the HIDA Trainee Network learn in your group? How could he or she support you in your group? The guest researcher could learn the Lattice Boltzmann method algorithm, multiscale modeling processes, and an understanding of coupled THMC-processes in geological porous media. Use of our techniques and software.
We will support the GPU High-Performance Computing Cluster in JURECA-DC. We will cooperate with the guest to develop their LBM codes. We also welcome guests with a basic background in machine learning, who want to perform joint research with us relevant to environmental systems.