Lernen Sie Helmholtz-Wissenschaftlerinnen und -Wissenschaftler kennen, die einen Gastforscher oder eine Gastforscherin für einen kurzfristigen Forschungsaufenthalt in ihrer Gruppe willkommen heißen möchten. Hier erfahren Sie, an welchen Projekten die Forschungsgruppen derzeit arbeiten, wie Ihr Beitrag dazu aussehen kann und was Sie von den spezifischen Forschungsansätzen lernen können.
Helmholtz-weite Kooperationen möglich
Wenn Sie einen passenden Host Supervisor suchen, beachten Sie bitte: Sie können zu diesem Zweck auch andere Wissenschaftlerinnen und Wissenschaftler (in der Regel PIs oder Head of Divisions) an den 18 Helmholtz-Zentren kontaktieren und mit Ihnen eine Teilnahme am Helmholtz Visiting Researcher Grant oder am HIDA Trainee Network vereinbaren. Die hier aufgeführte Liste stellt lediglich eine Auswahl von möglichen Hosts dar.
Eine Übersicht der Helmholtz-Zentren und der jeweiligen Forschungsbereiche finden Sie hier:
Helmholtz-Betreuerinnen und -Betreuer
Lernen Sie hier einige potentielle Gastgeberinnen und Gastgeber an verschiedenen Helmholtz-Zentren kennen und erfahren sie mehr über deren jeweilige Data Science-Forschung durch einen Klick auf die Karten.
Bitte beachten Sie: Kontaktieren Sie Ihren potenziellen Betreuer oder Ihre potenzielle Betreuerin bitte vorab per E-Mail, um ein Forschungsprojekt vorzuschlagen und zu besprechen. Reichen Sie erst nach dieser Klärung Ihre Bewerbung ein.
Wenn Sie Fragen haben, senden Sie bitte eine E-Mail an: hida@helmholtz.de
Sie möchten selbst gerne Helmholtz-Gastgeber werden und suchen nach Unterstützung für Ihr Forschungsprojekt? Dann wenden Sie sich ebenfalls an die oben genannte E-Mail Adresse.
Forschungszentrum Jülich - FZJ
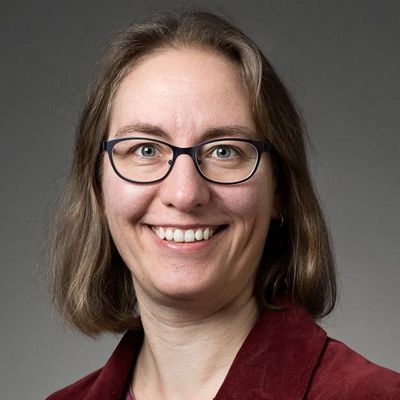
Ira Assent
Data Analytics and Machine Learning
Ansprechpartner
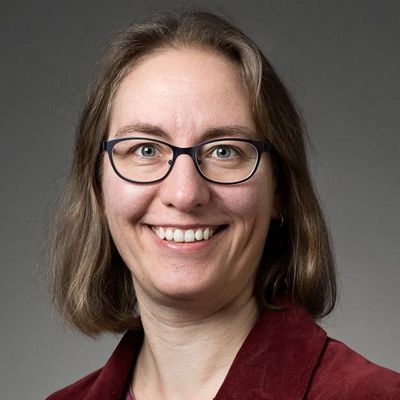
Short summary of your group's research: We aim at advancing theory and application of data sciences with a focus on image processing and computer vision for imaging as a scientific measurement tool. Our interests range from use-inspired, method-driven theory to application-driven research.
What infrastructure, programs and tools are used in your group? We work with state-of-the-art methods and algorithms in machine learning and data mining; most of our implementations are in python, and we make use of gpu-parallelization for efficiency and scalability; we devise methods that support scientists in other fields in analyzing and handling their data
What could a participant of the HIDA Trainee Network learn in your group? How could he or she support you in your group? You can learn more about how to train machine learning models, develop (variants of) such models, devise efficient algorithms, or use them to work with your data or with the data provided by our collaborators.
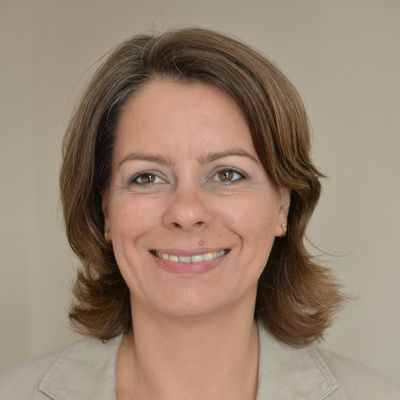
Simone Beer
Molecular Organization of the Brain
Ansprechpartner
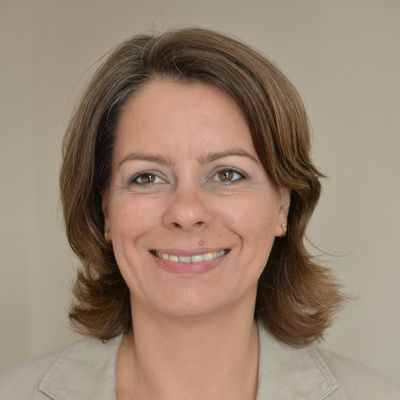
Short summary of your group's research: We use explainable AI on population-based studies to target neurodegenerative diseases, e.g. Alzheimer's disease. Machine learning algorithms like decision trees or tree ensembles can learn patterns in data, and the explanations are therefore promising tools to address interactions of genes, environment and lifestyle with respect to a certain trait or disease. We seek to evaluate and use different methods of explainable AI with respect to scientific discovery tasks.
What infrastructure, programs and tools are used in your group? Python, GitHub, access to various clinical and population-based databases like ADNI (the Alzheimer's Disease Neuroimaging Initiative), PET, hybrid MRI/PET and various laboratory techniques for preclinical and clinical research as well as research in basic neuroscience in a highly interdisciplinary environment.
What could a participant of the HIDA Trainee Network learn in your group? How could he or she support you in your group? A guest researcher will support in evaluating explainable AI for scientific discovery, especially with respect to the interaction of genetics, environment and lifestyle in neurodegenerative diseases.
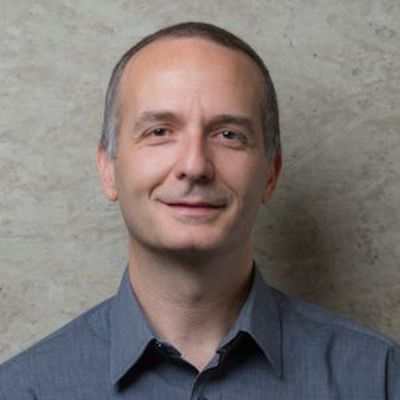
Eduardo di Napoli
Simulation and Data Laboratory Quantum Materials
Ansprechpartner
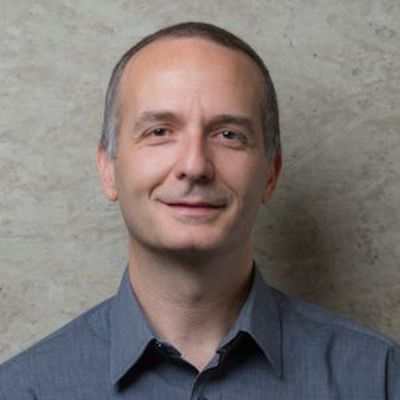
Short summary of your group's research
The Simulation and Data Laboratory Quantum Materials (SDLQM) serves as a dynamic hub for pioneering research at the intersection of simulation science and quantum materials. With a unique emphasis on leveraging High-Performance Computing and cutting-edge scientific Machine Learning, SDLQM offers a rich repository of expertise in quantum-based simulations within the realm of Materials Science. Our lab operates as a catalyst for innovation, actively engaging in dedicated projects and hosting research initiatives that delve into the essential domains of method refinement, algorithmic enhancement, and performance optimization. At the forefront of simulation science, SDLQM thrives on fostering cross-disciplinary collaborations spanning the global landscape. We take pride in our robust engagement within both the European and Japanese research arenas. Within the EU, our lab spearheads pioneering research and development initiatives, propelling the domain of Materials for Energy to new horizons. We are resolutely focused on facilitating exascale simulations of quantum transport, unraveling complexities that hold the key to transformative advancements. Our endeavors transcend borders, as we cultivate robust ties with esteemed partners in Japan, notably nurturing a dynamic synergy with the illustrious RIKEN supercomputing center in Kobe.
What infrastructure, programs and tools are used in your group?
Guided by an unwavering commitment to excellence, SDLQM not only facilitates extensive-scale simulations on state-of-the-art HPC platforms but also pioneers the training of intricate learning models driven by insights garnered from in-silico simulations. SDLQM operates at the heart of the Juelich supercomputing center, a pioneering institute that presently houses one of the largest supercomputers across the European Union. This convergence of minds and machines is set to elevate to unprecedented heights, with Europe's inaugural exascale supercomputer slated for 2024. Immerse yourself in a realm of unbounded possibilities, as the lab cultivates expertise encompassing high-performance computing, parallel programming, numerical linear algebra, quantum transport simulation, functional Renormalization Group methods and Tensor Networks. As a nexus at the frontier of knowledge, we seamlessly unite the realm of materials science simulation with the realm of massively parallel machines, thereby nurturing a community driven by cutting-edge innovation and collaborative excellence.
What could a guest researcher learn in your group? How could he or she support you in your group?
The doctoral researcher will be granted privileged access to JSC's cutting-edge supercomputing hardware, ushering them into the realm of computational supremacy. Working in close tandem with the group's accomplished experts, they will embark on a transformative journey of code development, adaptation, and optimization. Moreover, the doctoral researcher will gain firsthand exposure to the dynamic intersection of numerical linear algebra and materials science simulation. This immersive journey will encompass code integration, delving into the intricacies of parallel programming best practices, and honing indispensable software management skills. Join us in shaping the future of quantum-centric materials research, where innovative simulations and data-driven discoveries converge.
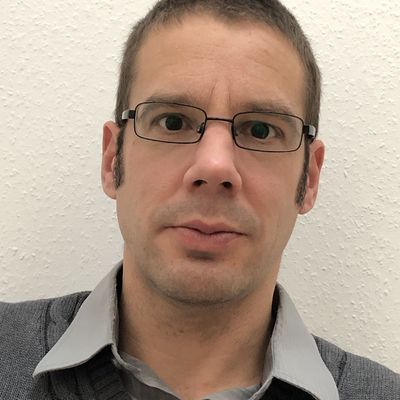
Andreas Kleefeld
Numerical and Statistical Methods
Ansprechpartner
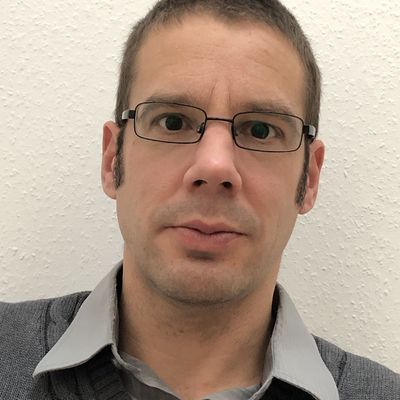
Three-sentence summary of your group's research: The ATML "Numerical and Statistical Methods" develops, implements, and tests new procedures/methods in the area of stochastic ordinary and partial differential equations that arise, for example, in image processing, acoustic, electromagnetic, and elastic scattering problems, as well as in reaction-diffusion-advection equations. In this context, the group also deals with algorithms for the solution of ill-posed inverse problems and the computation of eigenvalue problems taking real-world data into account. Furthermore, the group offers software support for supercomputers.
What infrastructure, programs and tools are used in your group? We develop software and tools in Fortran, Matlab, and Python depending on the requirements of the project.
For instance, solvers for acoustic, electromagnetic, and elastic scattering for time-harmonic waves in unbounded domains have been implemented and further used for related inverse problems. The high performance infrastructure of the Jülich Supercomputing Centre is at our disposal.
What could a guest researcher learn in your group? How could he or she support you in your group? A researcher in our group will learn how to efficiently solve partial differential equations in unbounded domains with boundary integral equations numerically. A recent development is mainly focusing on the efficient numerical calculation of interior transmission eigenvalues which can be used to visualize the interior of a given three dimensional object to uncover location, size, and shape of an inclusion for either given real-world or synthetic data which is the aim in non-destructive testing. A researcher can support our group with the development of this algorithm for HPC. Moreover, the mathematical theory still needs to be established. Finally note that a detailed description of our projects can be found at our homepage.
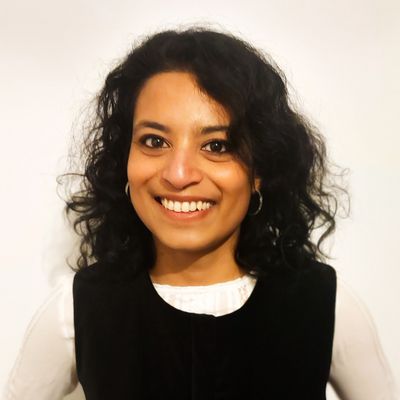
Jenna Poonoosamy
Reactive Transport
Ansprechpartner
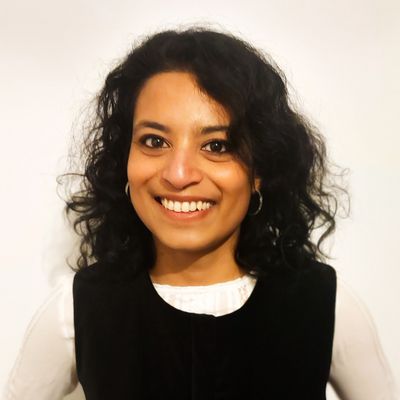
The institute performs cutting-edge research in the fields of nuclear waste management and safety, considering the process of “Energiewende”, the transition of the German energy system. This comprises fundamental as well as applied research and development for the safe management of nuclear wastes, covering issues from the atomic scale to the macroscopic scale of actual waste forms and waste packages or the engineered barrier system of deep geological repositories for nuclear wastes.
Short summary of your group's research: We apply innovative experimental and computational approaches across the scale to analyze and interpret complex coupled thermal-hydraulical-mechanical-chemical (THMC) processes in porous media related to energy applications. Recently, I have acquired an ERC project to investigate the gas water mineral interactions in confinement https://cordis.europa.eu/project/id/101040341. In this project, AI and Neural network tools will be used to (i) automate the analysis of microfluidics experiments and process experimental results (ii) derivation of constitutive equations to rationalize complex processes and (iii) accelerate geochemical processes.
What infrastructure, programs and tools are used in your group? Reactive transport tool software (Pflotran, Openegeosys-Gems, comsol multiphysics). Geochemical simulation. Image processing based on machine learning. In addition, we also have a microfluidic laboratory equipped with confocal Raman microscopy, IR and fluorescence microscopy.
What could a participant of the HIDA Trainee Network learn in your group? How could he or she support you in your group? Multiphase flow using Comsol multiphysics and geochemical modelling using phreeqc or GEMS. We have conducted novel experiments in the field of geochemistry; a lab on a chip approach to investigate radionuclide incorporation in mineral phases in a confined nano volume of (1-10nL) solution. The guest researcher would work on simulating droplet generation using comsol multiphysics and conduct geochemical modelling.
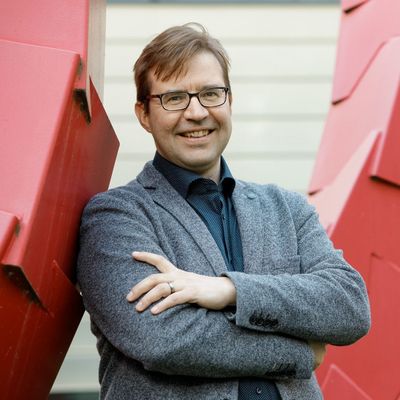
Stefan Sandfeld
Materials Data Science and Informatics
Ansprechpartner
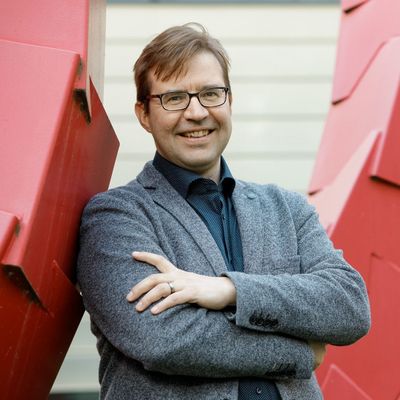
Three-sentence summary of your group's research: Research work at the IAS-9 is located at the intersection of data science and machine learning, research data management and semantic technology, and simulation in the field of computational materials science. In the data science department we investigate how predictive and generative machine learning models are able to replace or accelerate computationally expensive aspects of simulations; and as a second main topic we perform data mining of and method development for microscopy images and data. In the field of materials simulations we are particularly interested in predicting the structure-property relation in the context of solid-mechanical systems. In the field of RDM we are involved in software development and design ontologies and metadata schemas together with domain scientists.
What infrastructure, programs and tools are used in your group? Probably the main "tool" used in the IAS-9 is programming. 90% of the time, we use Python for writing code, but once in a while there is also some C/C++ or Java. Half of our activities are taking place on HPC hardware.
What could a guest researcher learn in your group? How could he or she support you in your group? A guest researcher can learn how (data) science is done in our team and how we sometimes also collaboratively work together on small projects -- sometimes with people from the field of simulation, research data management, and data science.
Helmholtz-Zentrum HEREON
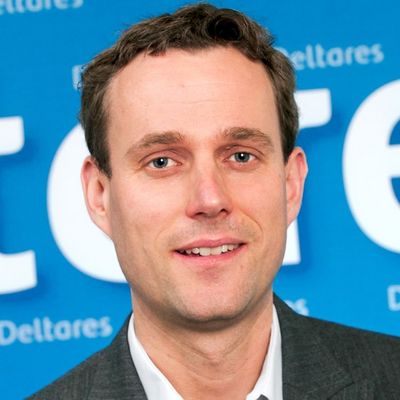
Laurens Bouwer
Climate Services for Adaptation
Ansprechpartner
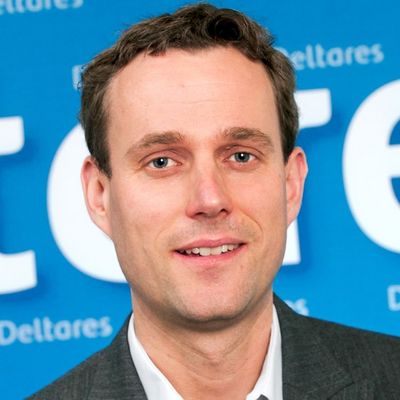
Three-sentence summary of your group's research: The interdisciplinary team at GERICS develops scientifically based prototype products and services to support decision-makers in politics, business and public administration in adapting to climate change. We are located in the historic "Chilehaus" in the centre of Hamburg. Specifically, my group develops applications in the field of water resources (water supply, flooding), as well as socio-economic impacts, such as damages and health impacts.
What infrastructure, programs and tools are used in your group? We operate our own regional climate model REMO, and participate in the WCRP EURO-CORDEX network. In addition, we develop state of the art tools for pre- and post-processing of climate and other environmental data.
What could a guest researcher learn in your group? How could he or she support you in your group? We use data science methods and machine learning for improving simulations as well as visualistion of our results. Example applications are the application of ML for data post-processing of weather and climate predictions (Link), impact models for flood damage assessment (Link), and health impact modelling (Link). We are also open to accept novel ideas and proposals of other applications of data science methods, that fit the goals of GERICS and our product portfolio.
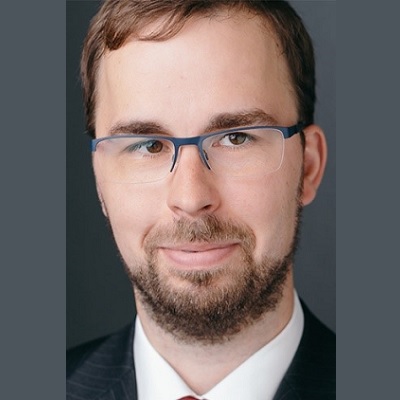
Christian Feiler
Surface Science
Ansprechpartner
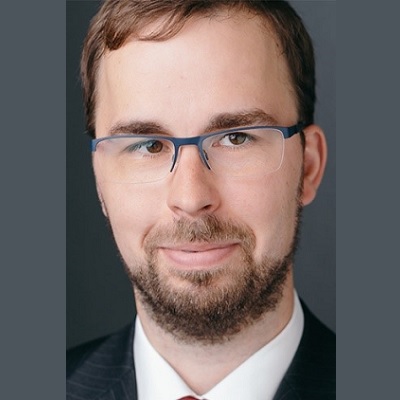
Short summary of your group's research: The research activities at the Institute of Surface Science revolve around the development of ultra‐light‐weight materials and surface protection technologies for the application areas air and ground transportation, infrastructures, regenerative medicine, as well as energy storage and functional materials. Gaining control over surface reactivity characteristics and a reliable prediction of service-life aspects of the employed materials are prerequisites to unlock their full potential in the respective application areas. In this context, we utilise information derived from multiscale simulations, experimental investigations in labs and field data from industrial end-users to gain a comprehensive insight into the material behaviour and employ a variety of supervised and unsupervised machine learning techniques for the discovery of effective additives prior to experimental testing as well as to predict how materials and structures behave during their service-life after exposure to the environment with focus on a sustainable circular economy.
What infrastructure, programs and tools are used in your group? Access to Hereon’s HPC Cluster, HPC workstations in the group for less demanding jobs, Several simulation software packages (DFT, MD, FEM) and tools to generate molecular descriptors (alvaDesc, rdkit), Python-based machine learning workflows.
What could a participant of the HIDA Trainee Network learn in your group? How could he or she support you in your group?
Our offer:
- Insights into material science with focus on materials degradation and corresponding corrosion mitigation strategies.
- Techniques to generate molecular descriptors for quantitative structure-activity/property relationship models and approaches to identify features that are relevant to the chosen target property as well as subsequent model development.
- Opportunity to validate the robustness of your machine learning model(s) directly with our experimentalists who provide the training data.
Your support:
- You could either apply your machine learning knowledge to our existing training data or shape a new project together with our interdisciplinary team with focus on corrosion mitigation and/or predictive maintenance.
- You could employ your experience in materials modelling to generate additional input features for our predictive models.
- You could help us to develop tools that can be utilised by end-users without any domain expertise
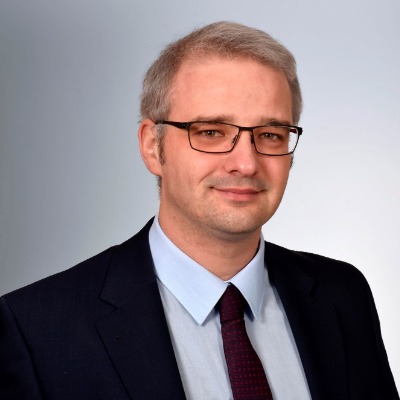
Paul Jerabek
Hydrogen Technology
Ansprechpartner
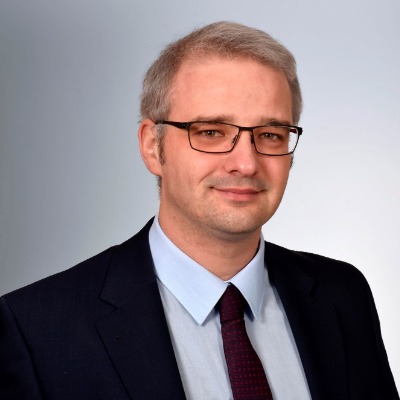
Short summary of your group's research: We perform state-of-the-art multi-scale simulations of hydrogen storage materials, e.g. interstitial metal hydrides or complex hydride systems. For that, we develop digital workflows bridging the atomistic scale with the mesocopic regime by coupling accurate ab-initio calculations with thermodynamic modeling and phase-field simulations. Our set out goal is to be able to understand and accurately predict materials properties as independently from experimental input as possible.
What infrastructure, programs and tools are used in your group? We have access to Hereon's own HPC cluster (more than 160 nodes with 48 CPUs/node) that we utilize for running various molecular and periodic quantum chemical program packages (VASP, QuantumEspresso, CASTEP, ORCA, Gaussian etc.)
Furthermore, we use open-source software to perform thermodynamic modeling (OpenCALPHAD) and phase-field simulations (FiPy, MOOSE).
What could a participant of the HIDA Trainee Network learn in your group? How could he or she support you in your group?
What you can learn in our group:
- You will be able to gain or deepen your understanding in quantum chemical methods like DFT or AIMD for structural optimization, calculation of reaction energies and pathways and prediction of spectroscopic properties
- You will learn how to perform thermodynamic modeling to predict phase diagrams for multi-component systems via an ab-initio assissted CALPHAD methodology
- You can be trained in utilizing phase-field simulations for prediction of microstructure evolution and kinetic properties of materials on the mesoscale
How you can support us:
- You can complement our team by working with our established methodologies on your own project on the topic of hydrogen storage materials
- You can help with automatizing and streamlining some of our scale-bridging, digital workflows
- You can kickstart our planned activities for utilizing machine-learning techniques for some of our computational methods
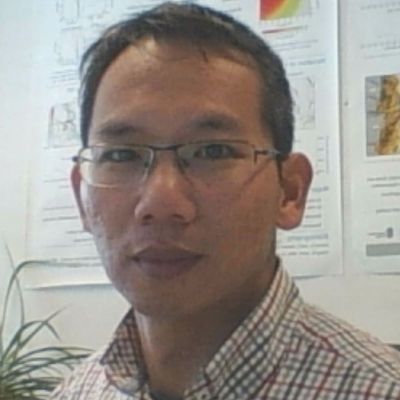
Wenyan Zhang
Sediment Transport and Morphodynamics
Ansprechpartner
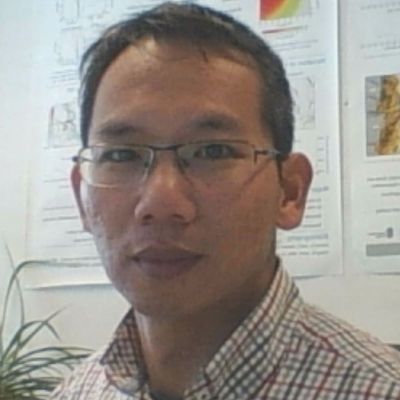
Short summary of your group's research: Continental margins are constantly changing their shape through erosion and sediment deposition. In terms of climate protection and sustainable coastal development, the “Sediment Transport and Morphodynamics” department studies in detail the interactions between morphodynamics, ecosystem functions and biogeochemistry. We apply process-based models combined with artificial intelligence to investigate particle transport, coastline change, morphodynamics and their role in ecosystem functioning.
What infrastructure, programs and tools are used in your group? We develop and apply numerical models (physics-biology-biogeochemistry coupled) with parameterizations optimized by data-driven approaches. The models include SCHISM, ROMS and Delft3D.
What could a participant of the HIDA Trainee Network learn in your group? How could he or she support you in your group? We provide guidance to learn hybrid modeling combining process-based models and data-driven optimization. We hope the guest research could bring the expertise in artificial intelligence with application to remote sensing data (especially satellite images) and causal linkage identification from multivariate datasets.
Helmholtz-Zentrum Dresden-Rossendorf - HZDR
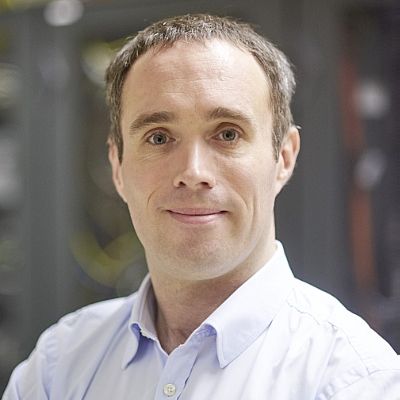
Michael Bussmann
Sustainable Systems Science
Ansprechpartner
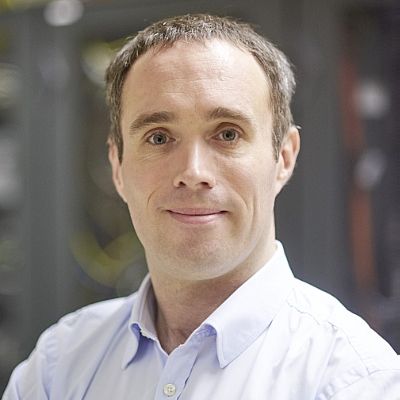
Short summary of your group's research: The Center for Advanced Systems Understanding is a new center working in the field of data-driven systems science. We strive to understand complex systems in an interdisciplinary way using newest digital technologies. From modelling the formation of complete organisms from a single cell to studying the interplay between ecosystems and biodiversity, from understanding what exoplanets look like to working on the mobility of the future, CASUS provides a diverse and welcoming research culture.
What infrastructure, programs and tools are used in your group? We provide access to a large HPC systems for both high performance compute simulations and large-scale AI. We have expertise in high performance computing, scalable AI, human-machine interaction, and strive for the development of professional research software and solutions. We have access to some of the largest compute resources on the planet.
What could a participant of the HIDA Trainee Network learn in your group? How could he or she support you in your group? Trainees have a large variety of options, from learning High Performance Computing (including GPU and FPGA computing) to newest developments in AI (physics-informed NN, Invertible NNs, Normalizing Flows, ...), from applied mathematics and data science foundations to using their skills for a variety of applications in physics, ecology, digital health, autonomous systems, cyber security, earth systems science and more.
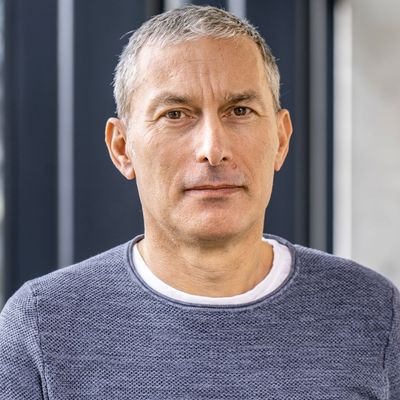
Richard Gloaguen
Exploration and characterization
Ansprechpartner
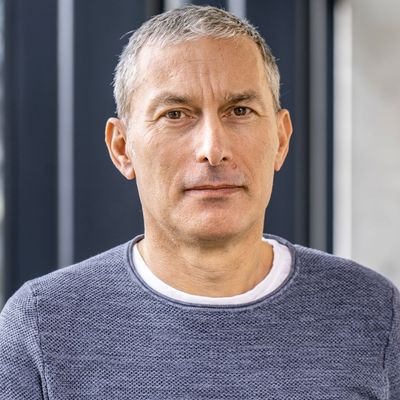
Short summary of your group's research: We are developing imaging technologies for the characterization of complex material streams and the Earth's surface. Our approach is based on sensor integration and processing using machine learning. Our platforms include drones, robots and conveyor systems.
What infrastructure, programs and tools are used in your group? We have our own imaging sensors, a GPU HPC and develop our own python tools.
What could a participant of the HIDA Trainee Network learn in your group? How could he or she support you in your group? A guest researcher would learn to work with cutting edge technology and process data with the latest developments in computer vision and machine learning.
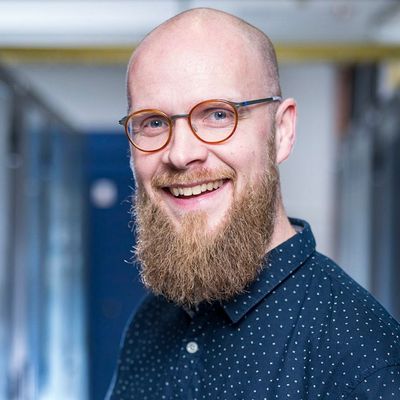
Peter Steinbach
Künstliche Intelligenz
Ansprechpartner
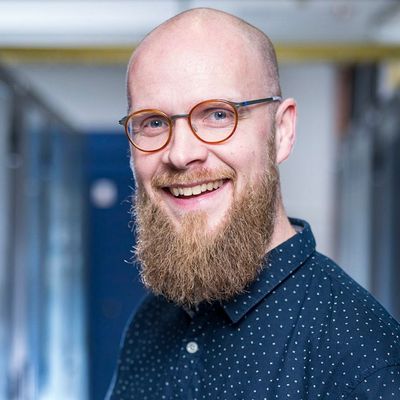
Three-sentence summary of your group's research: We are Helmholtz.AI consultants and work on a wide range of projects. Our core strengths presently are digital twins using machine learning (simulation based inference, surrogate modelling), pattern recognition and object detection in images and other modalities, anomaly detection, uncertainty quantification and prediction robustness. As a plus, we have a strong background in teaching.
What infrastructure, programs and tools are used in your group? We use HPC infrastructure locally and available across Germany. We so far, have concentrated on the use of libraries like pytorch, scikit-learn, scikit-image to which we occasionally contribute.
What could a guest researcher learn in your group? How could he or she support you in your group? In our team, a scientist could learn about how to make a machine learning model more trustworthy (with respect to stability, fairness, uncertainty, robustness and explainability). We can offer guidance on each of the topics we specialize in: digital twins, surrogate modelling, object recognition, anomaly detection, prediction robustness. We can also help to set up a reprucible and scalable machine learning workflow. If you like to learn more, please let me know.
We always welcome support in converting our learnings into open-source tools that the community at large can reuse subsequently.