Lernen Sie Helmholtz-Wissenschaftlerinnen und -Wissenschaftler kennen, die einen Gastforscher oder eine Gastforscherin für einen kurzfristigen Forschungsaufenthalt in ihrer Gruppe willkommen heißen möchten. Hier erfahren Sie, an welchen Projekten die Forschungsgruppen derzeit arbeiten, wie Ihr Beitrag dazu aussehen kann und was Sie von den spezifischen Forschungsansätzen lernen können.
Helmholtz-weite Kooperationen möglich
Wenn Sie einen passenden Host Supervisor suchen, beachten Sie bitte: Sie können zu diesem Zweck auch andere Wissenschaftlerinnen und Wissenschaftler (in der Regel PIs oder Head of Divisions) an den 18 Helmholtz-Zentren kontaktieren und mit Ihnen eine Teilnahme am Helmholtz Visiting Researcher Grant oder am HIDA Trainee Network vereinbaren. Die hier aufgeführte Liste stellt lediglich eine Auswahl von möglichen Hosts dar.
Eine Übersicht der Helmholtz-Zentren und der jeweiligen Forschungsbereiche finden Sie hier:
Helmholtz-Betreuerinnen und -Betreuer
Lernen Sie hier einige potentielle Gastgeberinnen und Gastgeber an verschiedenen Helmholtz-Zentren kennen und erfahren sie mehr über deren jeweilige Data Science-Forschung durch einen Klick auf die Karten.
Bitte beachten Sie: Kontaktieren Sie Ihren potenziellen Betreuer oder Ihre potenzielle Betreuerin bitte vorab per E-Mail, um ein Forschungsprojekt vorzuschlagen und zu besprechen. Reichen Sie erst nach dieser Klärung Ihre Bewerbung ein.
Wenn Sie Fragen haben, senden Sie bitte eine E-Mail an: hida@helmholtz.de
Sie möchten selbst gerne Helmholtz-Gastgeber werden und suchen nach Unterstützung für Ihr Forschungsprojekt? Dann wenden Sie sich ebenfalls an die oben genannte E-Mail Adresse.
CISPA Helmholtz-Zentrum für Informationssicherheit
Das CISPA Helmholtz-Zentrum für Informationssicherheit gehört zu den weltweit führenden Forschungseinrichtungen im Bereich Cybersicherheit. Es erforscht theoretisch und praktisch die Gefahren des digitalen Raums und entwickelt Schutzmaßnahmen gegen Cyberangriffe. Neben der Ausbildung künftiger Cybersicherheitsexpert:innen arbeitet CISPA interdisziplinär und verfolgt das Ziel, die digitale Zukunft durch exzellente Forschung sicherer zu gestalten.
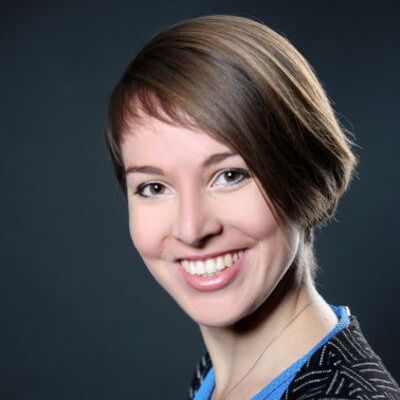
Franziska Boenisch
Trustworthy Machine Learning
Ansprechpartner
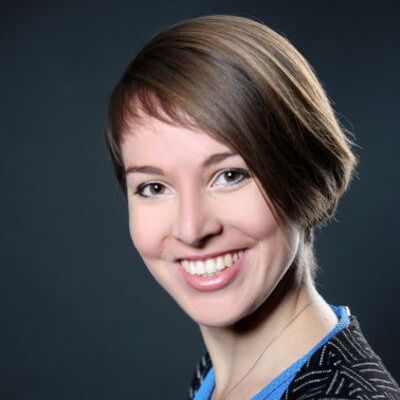
Three-sentence summary of your group's research: Our group, the SprintML lab focuses their research on Secure, Private, Robust, INterpretable, and Trustworthy Machine Learning. We make sure that data processing through machine learning models respects the privacy of their training data, and that after training, the model makes robust, secure, and interpretable predictions.
What infrastructure, programs and tools are used in your group? We rely on high performance computing infrastructure and GPU clusters from the Helmholtz association. Our software is mainly developed in Python and deployed to the GPU clusters in form of GitHub containers.
What could a guest researcher learn in your group? How could he or she support you in your group? A participant under the Visiting Researcher Grant will learn how to automatically process data and manage information through the lens of trustworthy machine learning. The participant will be given the opportunity to discover the risks to machine learning privacy, robustness, and security that arise from the data itself or the training procedure. They will explore mitigation methods to the most crucial risks, and get the chance to implement those themselves, or expand them to new contexts. Finally, the participant can extend their knowledge on publishing academic results in the area of trustworthy machine learning, and will, in the best case, contribute to a paper submission to a top tier machine learning conference. We welcome all participants with a background in machine learning, and an interest in developing data processing that goes beyond considering only utility of the resulting application.
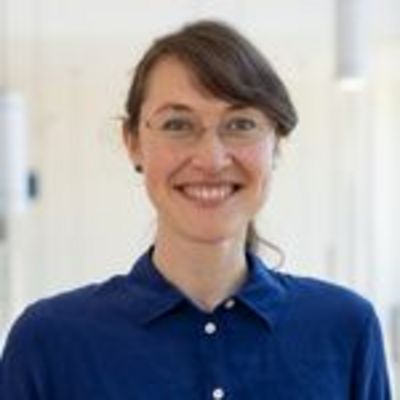
Rebekka Burkholz
Relational Machine Learning
Ansprechpartner
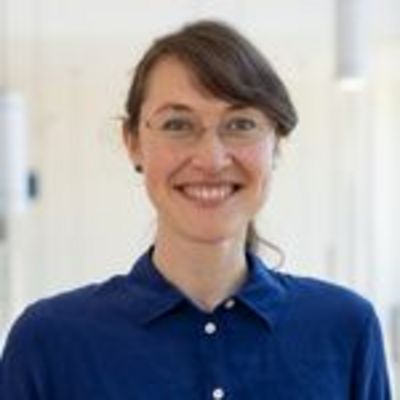
Three-sentence summary of your group's research: Our main goal is to reduce the computational costs associated with deep learning based on algorithmic innovations and theoretical insights with practical implications. We particularly enjoy tackling problems that involve graph based data. Most of the applications that motivate us are in the biomedical domain or economics ranging from modeling gene regulatory dynamics, gaining insights into cancer drivers, understanding sex differences, or analyzing systemic risk in international food trade.
What infrastructure, programs and tools are used in your group? As a lot of our projects involve deep learning and neural network sparsification, we make use of GPU clusters.
What could a guest researcher learn in your group? How could he or she support you in your group? We would be happy to collaborate on interesting applications as well as method development in the machine learning domain.
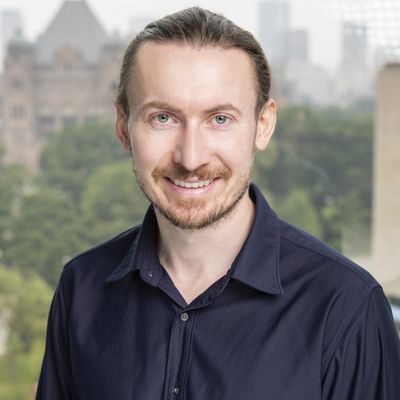
Adam Dziedzic
Machine Learning
Ansprechpartner
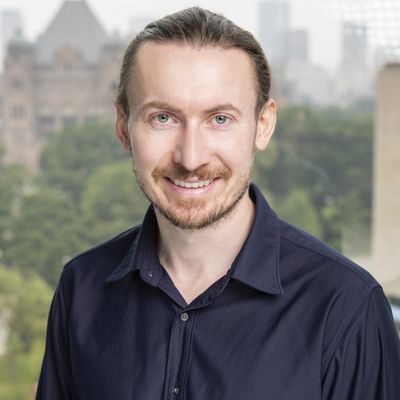
Three-sentence summary of your group's research: Our research is focused on secure and trustworthy Machine Learning as a Service (MLaaS). We design robust and reliable machine learning methods for training and inference of ML models while preserving data privacy and model confidentiality. We are focused on the state-of-the-art ML methods and models, such as, self-supervised learning, large language and vision models (LLMs), foundational models.
What infrastructure, programs and tools are used in your group? We use large scale GPU clusters and program in the latest ML frameworks.
What could a guest researcher learn in your group? How could he or she support you in your group? As a guest researcher you can learn how to use tools from data privacy and model confidentiality to enable trustworthy machine learning. We also support our guests with theoretical analysis as well as running large scale experiments to publish at the top ML conferences.
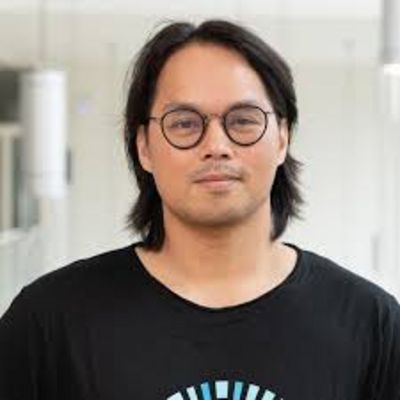
Krikamol Muandet
Machine Learning
Ansprechpartner
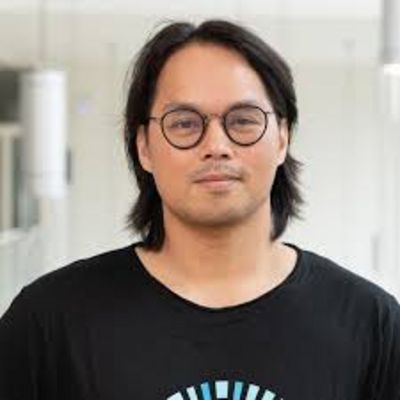
Three-sentence summary of your group's research:
The group’s research aims at understanding the principles that enable autonomous agents to learn from past experience and interact successfully with complex environments, and to use this understanding to design new machine learning algorithms.
What could a guest researcher learn in your group? How could he or she support you in your group?
Conducting and collaborating on world-class research projects that lie at the intersection of machine learning and economics.
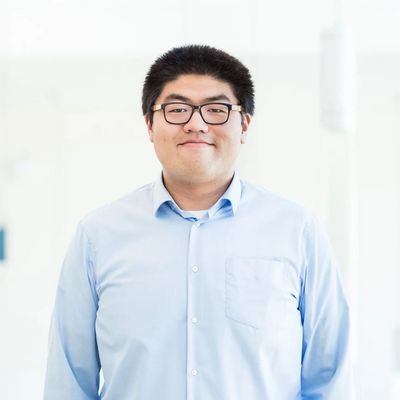
Yang Zhang
Learning and Intelligent Systems
Ansprechpartner
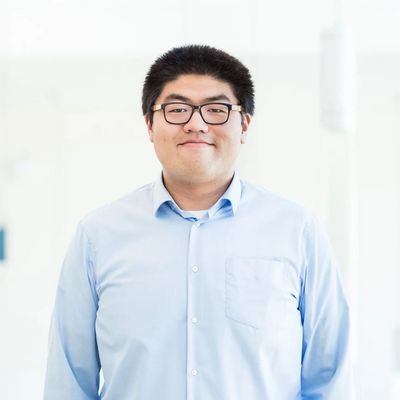
Three-sentence summary of your group's research: Yang Zhang is a faculty member at CISPA Helmholtz Center for Information Security, Germany. His research concentrates on trustworthy machine learning. Moreover, he works on measuring and understanding misinformation and unsafe content like hateful memes on the Internet. Over the years, he has published multiple papers at top venues in computer science, including CCS, NDSS, Oakland, and USENIX Security. His work has received the NDSS 2019 distinguished paper award and the CCS 2022 best paper award runner-up.
What could a guest researcher learn in your group? How could he or she support you in your group? The intern will either lead or assist in a research project conducted in the group. The intern will mainly work on the empirical side of the research, like collecting data, running machine learning models, etc.
What could a guest researcher learn in your group? How could he or she support you in your group? The guest researcher could learn experiences from our research domains and learn each other.
Helmholtz Munich
Helmholtz Munich widmet sich der Erforschung umweltbedingter Krankheiten – mit besonderem Schwerpunkt auf Ursachen, Behandlung und Vorbeugung von Diabetes, Fettleibigkeit, Allergien und chronischen Lungenerkrankungen.
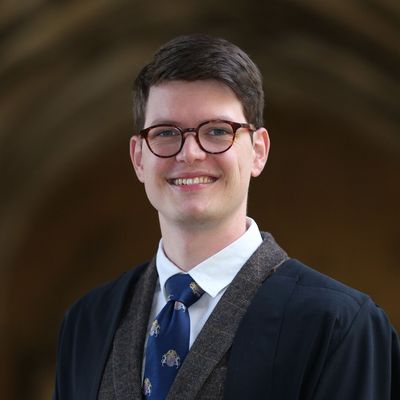
Vincent Fortuin
Efficient Learning and Probabilistic Inference for Science
Ansprechpartner
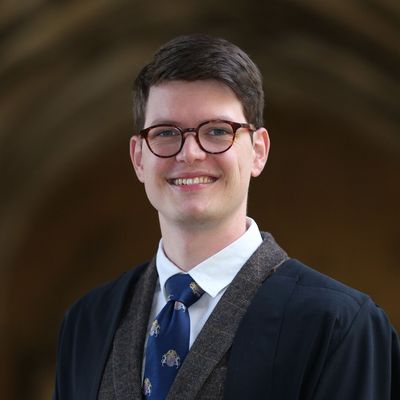
Three-sentence summary of your group's research: Our group focuses on the interface between Bayesian inference and deep learning with the goals of improving robustness, data-efficiency, and uncertainty estimation in these modern machine learning approaches. While deep learning often leads to impressive performance in many applications, it can be over-confident in its predictions and require large datasets to train. Especially in scientific applications, where training data is scarce and detailed prior knowledge is available, insights from Bayesian statistics can be used to drastically improve these models. Important research questions include how to effectively specify priors in deep Bayesian models, how to harness unlabeled data to learn re-usable representations, how to transfer knowledge between tasks using meta-learning, and how to guarantee generalization performance using PAC-Bayesian bounds.
What infrastructure, programs and tools are used in your group? We make use of high-performance CPU and GPU compute clusters (via Slurm) and regularly use deep learning frameworks (mostly PyTorch and JAX) together with the standard Python data analysis and visualization stack (scikit-learn, pandas, numpy, matplotlib, etc). On the theory side, we are interested Bayesian model selection, approximate Bayesian inference, and PAC-Bayesian generalization bounds.
What could a guest researcher learn in your group? How could he or she support you in your group? You can learn about the latest and greatest in Bayesian machine learning as well as how to train both small and (very) large deep learning models on one of the largest GPU clusters in Europe. You can apply cutting-edge ML models to a range of scientific data to push the boundaries of ML-powered scientific discovery, drug development, and understanding of health and disease. You can experience working directly at the intersection of theory and application with lots of freedom to explore either direction to suit your interests.
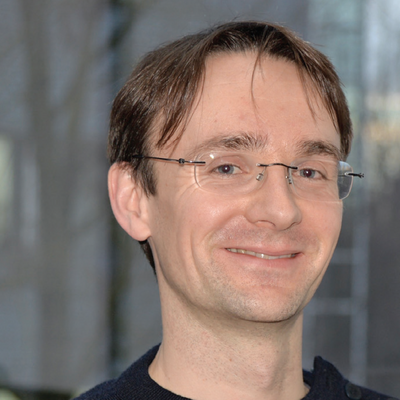
Julien Gagneur
Computational Molecular Medicine
Prof. Dr. Julien Gagneur
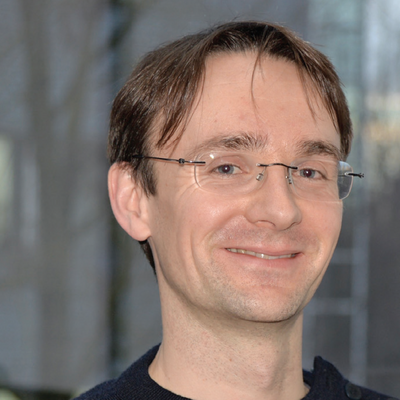
Three-sentence summary of your group's research: Our goal is an improved understanding of the genetic basis of gene regulation and its implication in diseases. To this end, we employ AI and statistical modeling of 'omic data and work in close collaboration with experimentalists. We are both based at the TUM and HMGU.
What infrastructure, programs and tools are used in your group? We work with python, ML libraries (pytorch, etc.) and R/Bioconductor.
We have developed multiple software for regulatory genomics and genetic diagnostics including :
- API and repos for trained ML models in genomics Kipoi
- sequence-based model of splicing MMSplice (GitHub)
- Base pair resolution modeling of genome-wide assays BPnet (Article regarding the topic)
- Omics based diagnosis of rare disorders: OUTRIDER, FRASER, DROP: (Protocol regarding the topic)
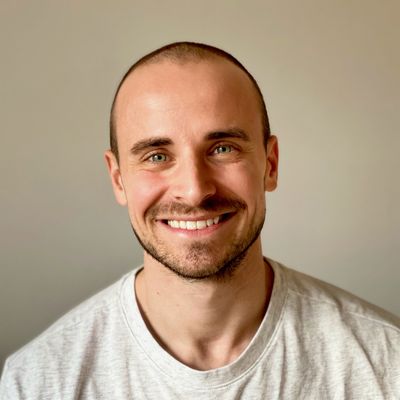
Niki Kilbertus
Ethics in Systems Design and Machine Learning
Ansprechpartner
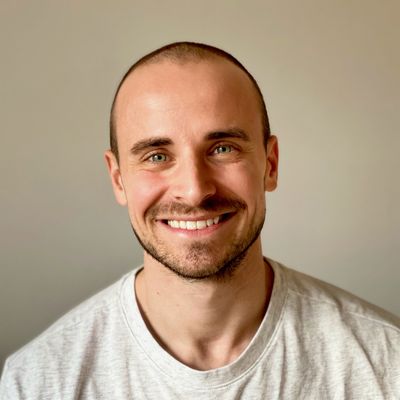
Three-sentence summary of your group's research: Our main research interests include causality, interpretable dynamical systems modeling, as well as machine learning systems that interact with humans, where we focus on reliable, fair and socially beneficial systems.
What infrastructure, programs and tools are used in your group? We make use of high performance CPU and GPU compute clusters (via Slurm) and regularly use ML frameworks (mostly jax and pytorch) together with the standard Python data analysis and visualization stack (scikit-learn, pandas, numpy, matplotlib, etc). On the theory side, we are interested in (partial) identifiability of causal effects in complex data modalities as well as in the identifiability and estimation of dynamical systems (ODEs, PDEs) from observational data.
What could a guest researcher learn in your group? How could he or she support you in your group? You can learn about the latest and greatest in causal learning and dynamic systems modeling as well as how to train both small and (very) large ML models on one of the largest GPU clusters in Europe. You can apply cutting-edge models to novel bio-medical data to push the boundaries of machine learning powered scientific discovery, drug development, and understanding of health and disease. You can experience working right at the intersection of theory and application with lots of freedom to explore either direction to whatever extent suits you.
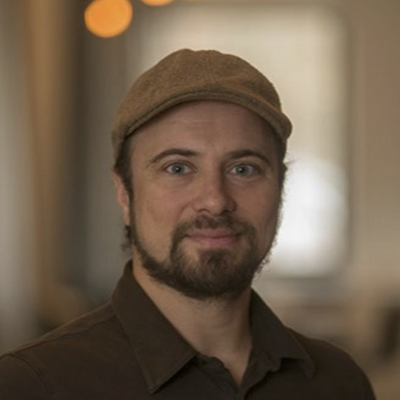
Christian L. Müller
Biomedical Statistics and Data Science
Ansprechpartner
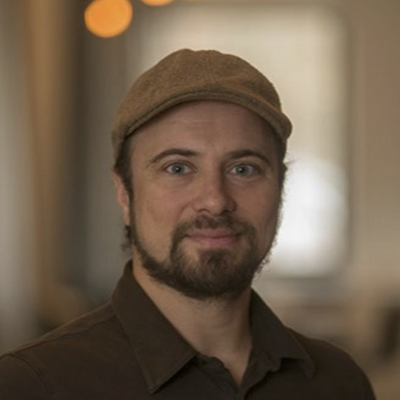
Three-sentence summary of your group's research: We do research in high-dimensional statistics, (non-)convex optimization, network inference, causal inference, and compositional data analysis with a special interest in microbiome research and microbial ecosystems. We care about sound statistical methodology and good software that is useful for answering broad statistical questions in computational biology and microbial ecology.
What infrastructure, programs and tools are used in your group? We use R, Python, (and MATLAB) for software development, and use GitHub for software deployment and versioning. Group communication is decentralized via Slack and Mattermost. We have (shared) office spaces at LMU Munich (City center) and Helmholtz Munich (in the North of Munich).
What could a guest researcher learn in your group? How could he or she support you in your group? High-dimensional statistics concepts, data analysis workflows for microbiome and microbial data, journal club covering state-of-the-art concepts ranging from experimental high-throughput biology to deep learning.
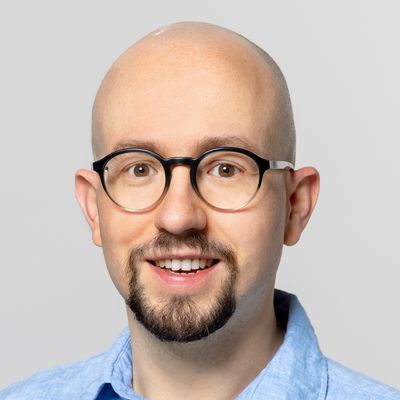
Bastian Rieck
AIDOS Lab at the Institute of AI for Health
Ansprechpartner
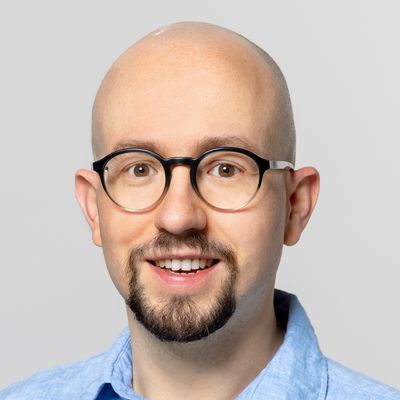
Short summary of your group's research: Our primary research interests are situated at the intersection of geometrical deep learning, topological machine learning, and representation learning. We want to make use of geometrical and topological information—also known as manifold learning—to imbue neural networks with more information in their respective tasks, leading to better and more robust outcomes. Following the dictum ‘theory without practice is empty,’ we also develop methods to address current challenges in biomedicine or healthcare applications.
What infrastructure, programs and tools are used in your group? HPC CPU/GPU cluster using Slurm, machine learning frameworks (mostly pytorch) and data analysis tools (scikit-learn, pandas, numpy) based on Python. On the theory side, we are world leaders in topological machine learning, as nascent branch of machine learning.
What could a participant of a HIDA Mobility Program learn in your group? How could he or she support you in your group? Getting to know cutting-edge AI research in geometrical deep learning or topological machine learning, including—but certainly not limited to—graph representation learning. At HMGU, we are sitting on a treasure trove of complex high-dimensional data sets, bearing the promise to substantially advance our understanding of disease-driving mechanisms, for instance. Visitors will benefit from being exposed to a unique combination of theory and practice, with the prospect of working on challenging, impactful applications. We need motivated doctoral researchers who can support our endeavour of developing the next generation of machine learning models to boldly tackle the challenges of today and tomorrow in healthcare!
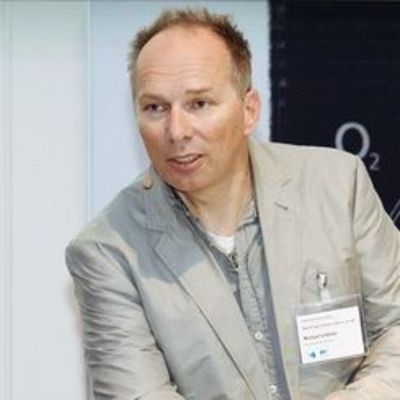
Michael Schloter
Comparative Microbiome Analysis
Ansprechpartner
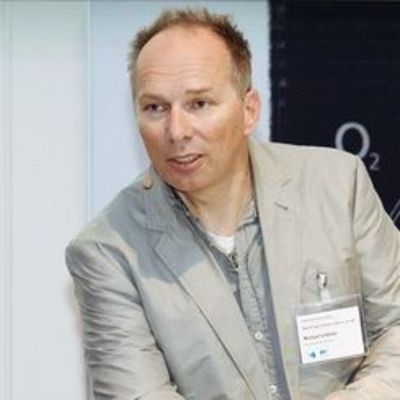
Three-sentence summary of your group's research: The human microbiome is a key component for our health. It is strongly influenced by environmental microbiota, which interact with the microbiome of barrier organs like skin or respiratory system. As a consequence, the reduced microbial diversity in the environment, resulting from climate- and global change, strongly impacts human – environment interactions, resulting in an increase in environmental diseases and infections. According to the planetary health concept the prevention of such diseases requires strategies which increase biodiversity in the environment.
We identify key microbiota from the environment, which trigger our health, develop strategies to promote the abundance of those microbiota in urban and indoor environments and analyze consequences for our health.
What infrastructure, programs and tools are used in your group?: We do next generation sequencing of microbiomes using metagneomics approaches from various sources and perform high throughput bioinformatics including KI based tools to reconstruct genomes of microborganisms.
What could a guest researcher learn in your group? How could he or she support you in your group?: In silco genome assembly of microbiota from metagenomics data and functional predications.
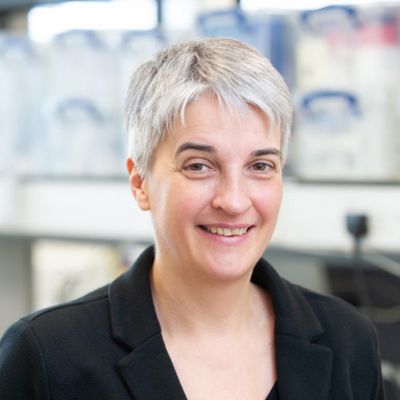
Julia Schnabel
Computational Imaging and AI in Medicine
Ansprechpartner
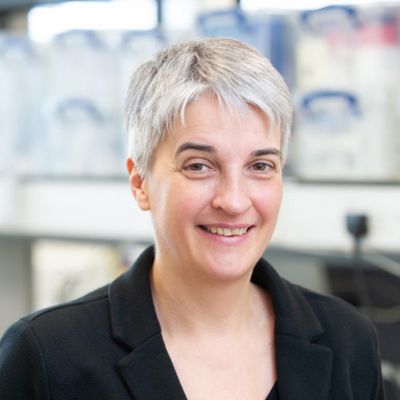
Short summary of your group's research: The Institute of Machine Learning in Biomedical Imaging (IML) focuses on research to leverage machine learning for the grand challenges in biomedical imaging in areas of unmet clinical need. Its goal is to fundamentally transform the use of imaging for diagnostics and prognostics. Novel and affordable solutions should empower clinics to make more accurate, fast and reliable decisions for early detection, treatment planning and improved patient outcome.
What infrastructure, programs and tools are used in your group? Novel and affordabel solutions should empower clinics to make more accurate, fast and reliablie decisions for early detection, treatment planning and improved patient outcome.
What could a participant of a HIDA mobility program learn in your group? How could he or she support you in your group? Deep learning and machine learning methods for intelligent imaging solutions, from imaging sensor to clinical applications
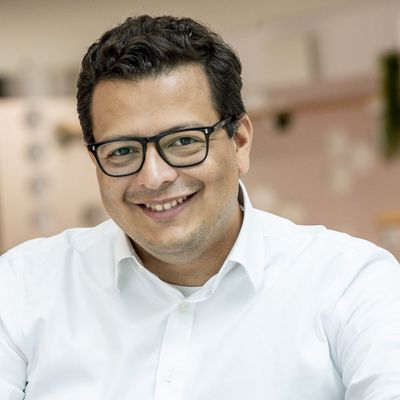
Carlos Talavera-López
Computational Immunobiology
Ansprechpartner
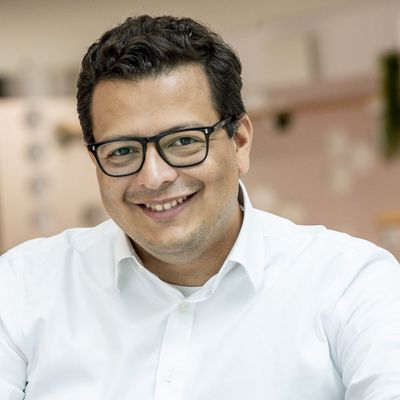
Short summary of your group's research: Our lab specialises in translational single cell biology with focus on infectious diseases. We develop integrative Carlos Talavera-LópezAI approaches to characterise cellular behaviour in health and disease with the aim of identifying diagnostic biomarkers that can be easily deployed in the clinic.
What could a participant of a HIDA Mobility Program learn in your group? How could he or she support you in your group? We are looking for a curious, dedicated scientist interested in learning to apply AI/ML methods to single cell multiome data, and to help us find ways to better communicate biological insights using novel data visualisations. Together, we will better understand the cellular social networks of inflammatory processes, and how these interactions could be potentially be used as diagnostic biomarkers.